Improving Power Quality measurements using deep learning for disturbance classification.
I2MTC(2023)
Abstract
Recent literature is investigating the problem of Power Quality (PQ) in electric power plant introducing artificial intelligence (AI) to classify disturbances using a sliding window approach. However, all the methods available in literature are capable of identify only one disturbance in a single window. In a previous work, we introduced an innovative algorithm called SSPQDD (Single Shot Power Quality Disturbance Detection) that allows to identify and classify properly multiple PQ disturbances (such as sag, swell, harmonics, transient, notch and interruption) in the same window. In this work, the SSPQDD algorithm is further developed and improved introducing a multiple sequence approach where multiple grids are analyzed simultaneously to further improve the algorithms capabilities. The improvement introduced in this work allows to recognize and classify two disturbances that happens simultaneously in the same window, which is currently not possible with any other algorithm available in literature. To further validate the proposed method, an experimental setup has been developed to acquire the electrical signal and identify real-time the PQ disturbances.
MoreTranslated text
Key words
Artificial intelligence,Classification algorithms,Convolutional neural networks,Power measurement,Power quality
AI Read Science
Must-Reading Tree
Example
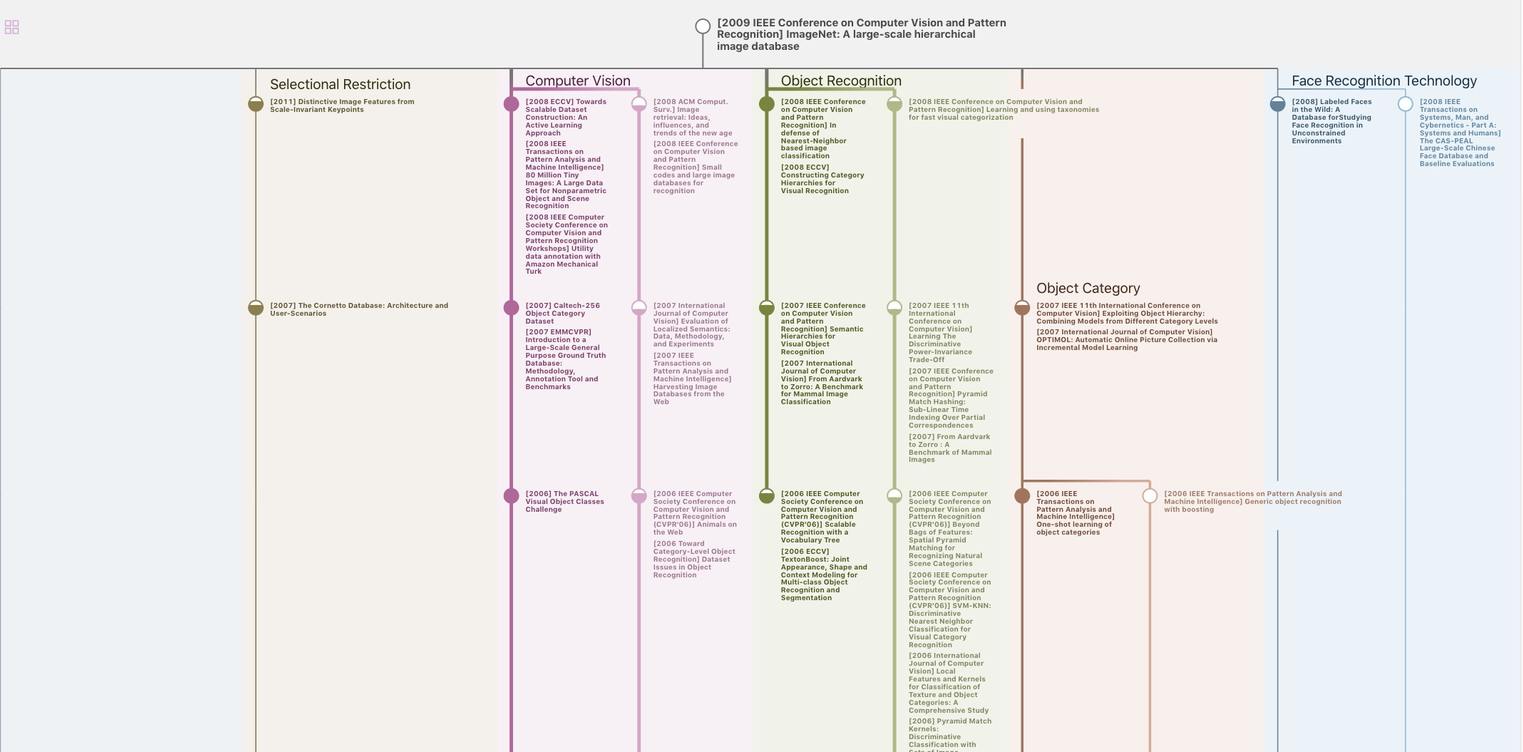
Generate MRT to find the research sequence of this paper
Chat Paper
Summary is being generated by the instructions you defined