A Fault Detection Method for Railway Turnout with Convex Hull-based One-Class Tensor Machine
2023 IEEE INTERNATIONAL INSTRUMENTATION AND MEASUREMENT TECHNOLOGY CONFERENCE, I2MTC(2023)
Abstract
Railway turnout is critical equipment for changing trains' directions, which directly impacts the safety and efficiency of operation. This study presents a novel abnormal detection method for railway turnouts with convex hull-based one-class tensor machine (CH-OCSTM) and monitoring signal images. As opposed to existing methods, it fully preserves the spatial structure and profile information in both data processing and model-building processes. Besides, a novel tensor-form classifier called CH-OCSTM is developed to improve the one-class support tensor machine (OCSTM)'s limitations in high computing complexity. First, the one dimensional original time-series signals are converted into two-dimensional images by data preprocessing and 2D representation. Next, the feature tensor is calculated by the CANDECOMP/PARAFAC decomposition method with the curve image data. Then, the CH-OCSTM model is built with the extracted feature tensor to implement the abnormal detection. The performance of the proposed method is evaluated and tested on two real-world operational current and power datasets. Experimental results show that the proposed method performs better than other existing approaches in accuracy and recall.
MoreTranslated text
Key words
Railway Turnout,Abnormal Detection,Support Tensor Machine
AI Read Science
Must-Reading Tree
Example
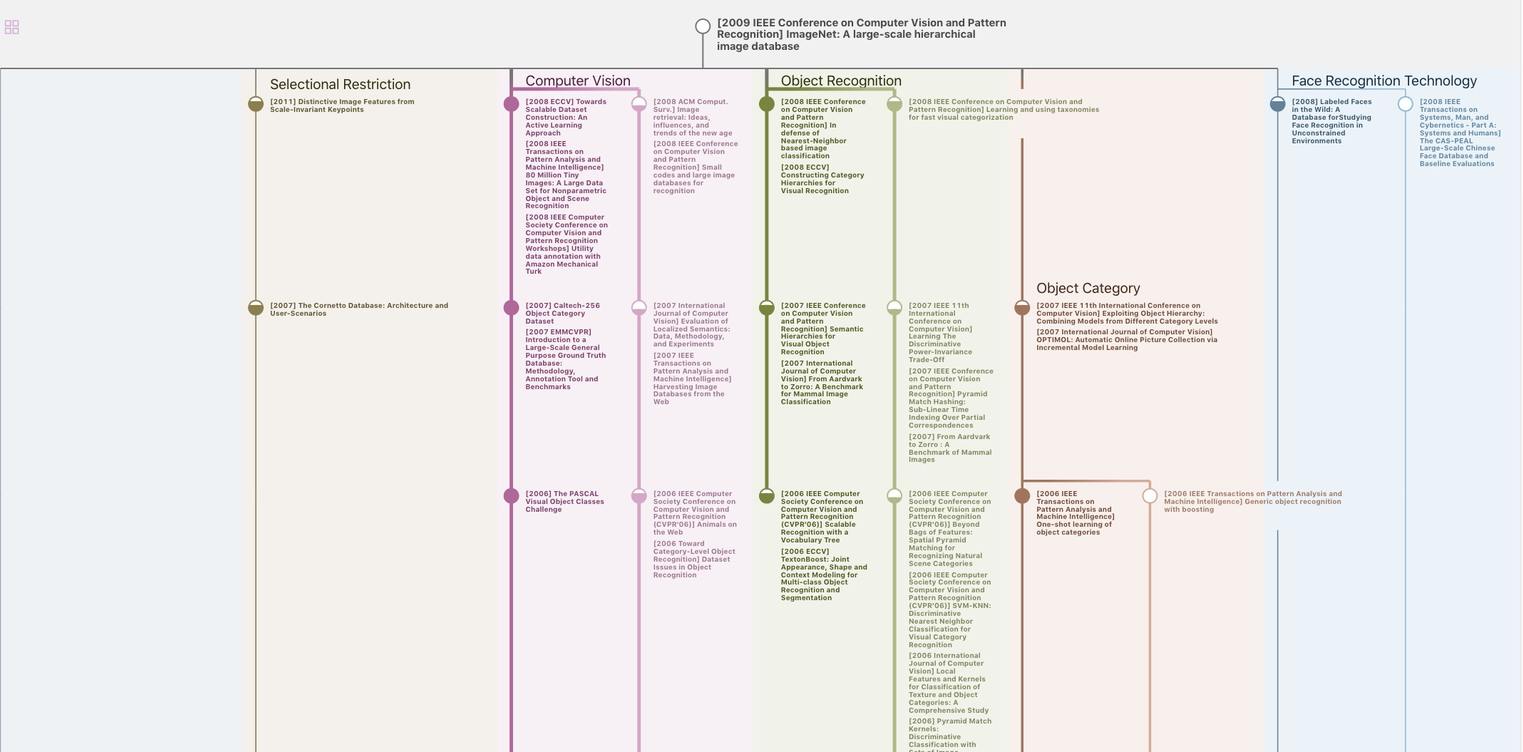
Generate MRT to find the research sequence of this paper
Chat Paper
Summary is being generated by the instructions you defined