Efficient Deep Ensemble Inference via Query Difficulty-dependent Task Scheduling.
ICDE(2023)
摘要
Deep ensemble learning has been widely adopted to boost accuracy through combing outputs from multiple deep models prepared for the same task. However, the extra computation and memory cost it entails could impose an unacceptably high deadline miss rate in latency-sensitive tasks. Conventional approaches, including ensemble selection, focus on accuracy while ignoring deadline constraints, and thus cannot smartly cope with bursty query traffic and queries with different hardness. This paper explores redundancy in deep ensemble model inference and presents Schemble, a query difficulty-dependent task scheduling framework. Schemble treats ensemble inference progress as multiple base model inference tasks and schedules tasks for queries based on their difficulty and queuing status. We evaluate Schemble on real-world datasets, considering intelligent Q&A system, video analysis and image retrieval as the running applications. Experimental results show that Schemble achieves a 5× lower deadline miss rate and improves the accuracy by 30.8% given deadline constraints.
更多查看译文
关键词
Ensemble learning,Deep learning,Efficient inference,Task scheduling
AI 理解论文
溯源树
样例
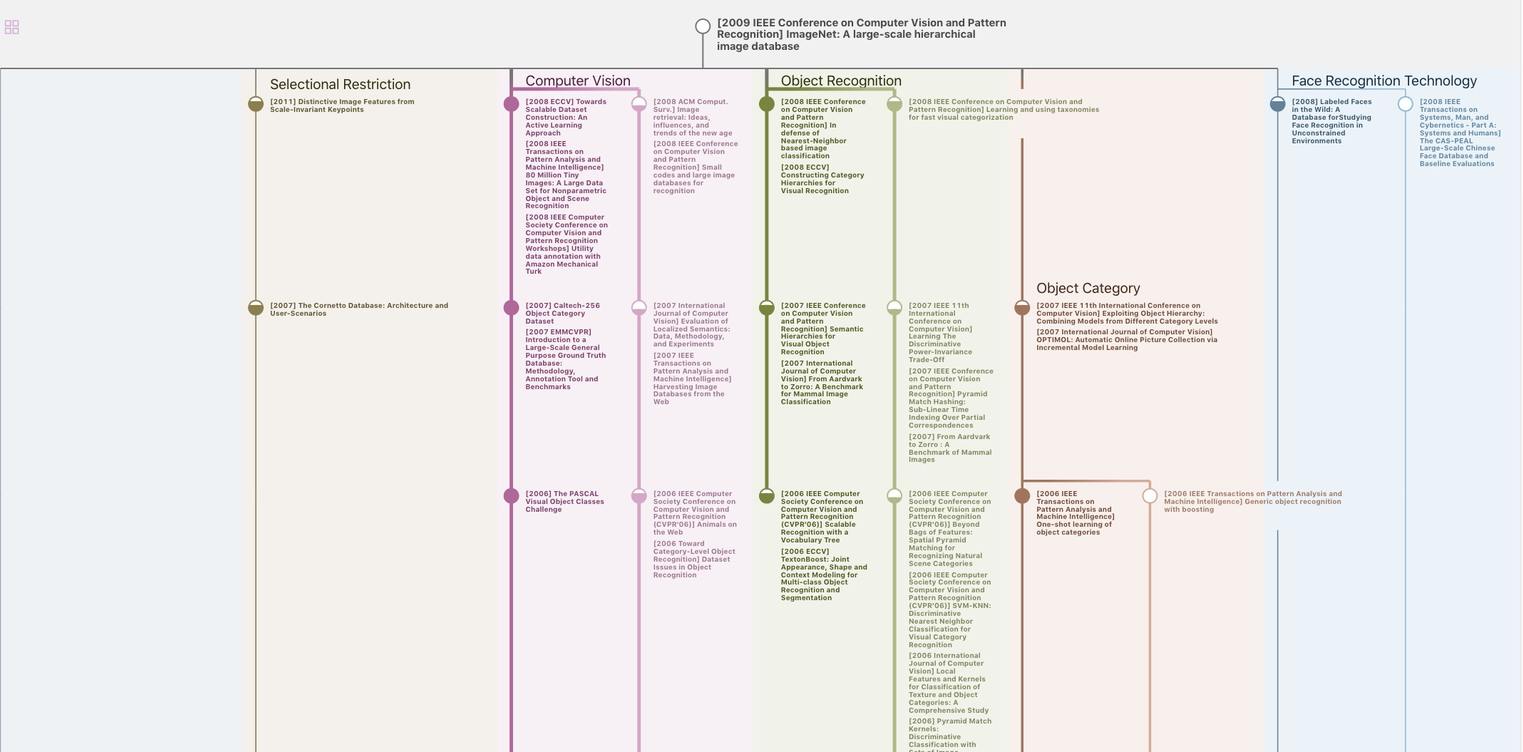
生成溯源树,研究论文发展脉络
Chat Paper
正在生成论文摘要