MCAN: Interpretable Multi-scale Component Analysis Network for Mechanical Fault Diagnosis.
I2MTC(2023)
摘要
Deep neural networks have provided unprecedented performance gains in the field of mechanical fault diagnosis. However, the practical deployment of deep networks is hindered by their black-box nature, i.e. lack of interpretability. In this paper, a multi-scale component analysis Network (MCAN) with high performance and interpretability was proposed for mechanical equipment fault diagnosis. MCAN is developed by unrolling the iterative solution algorithm of a morphological component analysis (MCA) model that incorporates multi-scale priors of the vibration signals into a network, so its network architecture is interpretable. In addition, this paper designs a feature visualization method to verify whether the network extracts meaningful features corresponding to fault mechanisms. Experimental verification results show that compared with other advanced networks, MCAN has the best performance and can effectively extract fault features.
更多查看译文
关键词
Interpretable neural network, morphological component analysis (MCA), algorithm unrolling, prognostic and health management (PHM)
AI 理解论文
溯源树
样例
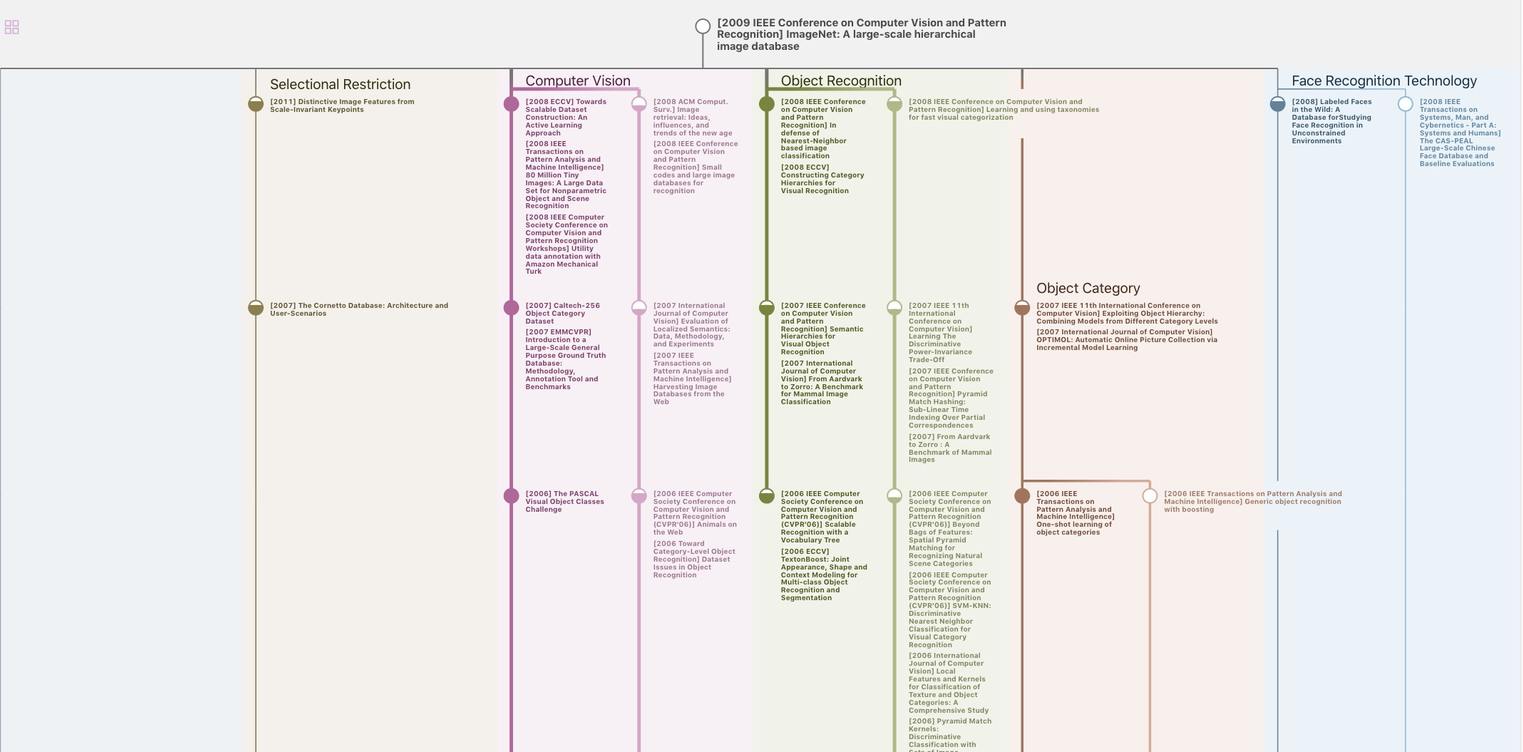
生成溯源树,研究论文发展脉络
Chat Paper
正在生成论文摘要