Breast Cancer Histologic Grade Identification by Graph Neural Network Embeddings.
IWBBIO (2)(2023)
Abstract
Deep neural networks are nowadays state-of-the-art methodologies for general-purpose image classification. As a consequence, such approaches are also employed in the context of histopathology biopsy image classification. This specific task is usually performed by separating the image into patches, giving them as input to the Deep Model and evaluating the single sub-part outputs. This approach has the main drawback of not considering the global structure of the input image and can lead to avoiding the discovery of relevant patterns among non-overlapping patches. Differently from this commonly adopted assumption, in this paper, we propose to face the problem by representing the input into a proper embedding resulting from a graph representation built from the tissue regions of the image. This graph representation is capable of maintaining the image structure and considering the relations among its relevant parts. The effectiveness of this representation is shown in the case of automatic tumor grading identification of breast cancer, using public available datasets.
MoreTranslated text
Key words
graph neural network embeddings,breast cancer
AI Read Science
Must-Reading Tree
Example
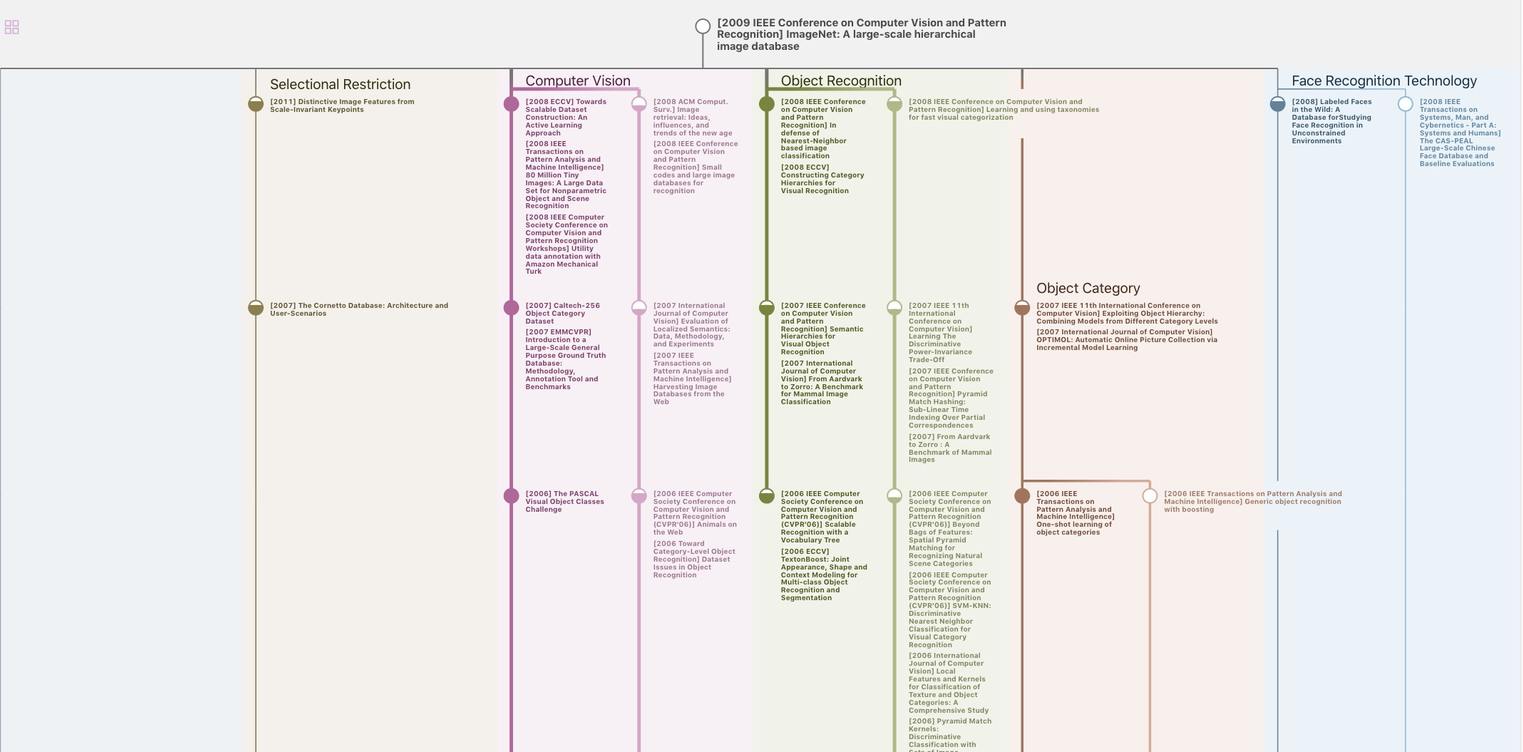
Generate MRT to find the research sequence of this paper
Chat Paper
Summary is being generated by the instructions you defined