Towards an Architecture for Collecting a Multidimensional Glioblastoma Dataset
2023 IEEE 36TH INTERNATIONAL SYMPOSIUM ON COMPUTER-BASED MEDICAL SYSTEMS, CBMS(2023)
摘要
Glioblastoma is the most common malignant brain tumor with a poor survival rate due to its high intra- and intertumor heterogeneity. The current heterogeneity determination is based on a microscopic analysis of Hematoxilyn and Eosinstained tumor slides carried out by experienced neuropathologists. There is no standardized procedure yet, to quantify heterogeneity, though. With the hypothesis that the amount of heterogeneity impacts overall survival, we aim to develop an objective method to capture heterogeneity. We were able to successfully implement an initial Machine Learning classification model for determining heterogeneity. However, the available dataset was insufficient to train a resilient and stable system. Therefore, we propose an architecture for a semi-automatic data collection and preprocessing framework easing the collection of large quantities of required data. While there exists a multitude of frameworks tackling parts of the tumor research area, no simple ready-to-use solution is present for the easy collection of tumor data in daily clinical routine, especially for high-resolution pathological images. We plan to implement the proposed architecture at the University Hospital Augsburg, Germany in 2023. The dataset created in this process will then be used as a resilient basis for heterogeneity classification and for analyzing glioblastomas in general.
更多查看译文
关键词
Tumor Classification,Tumor Segmentation,Machine Learning,Glioblastoma,Data Acquisition
AI 理解论文
溯源树
样例
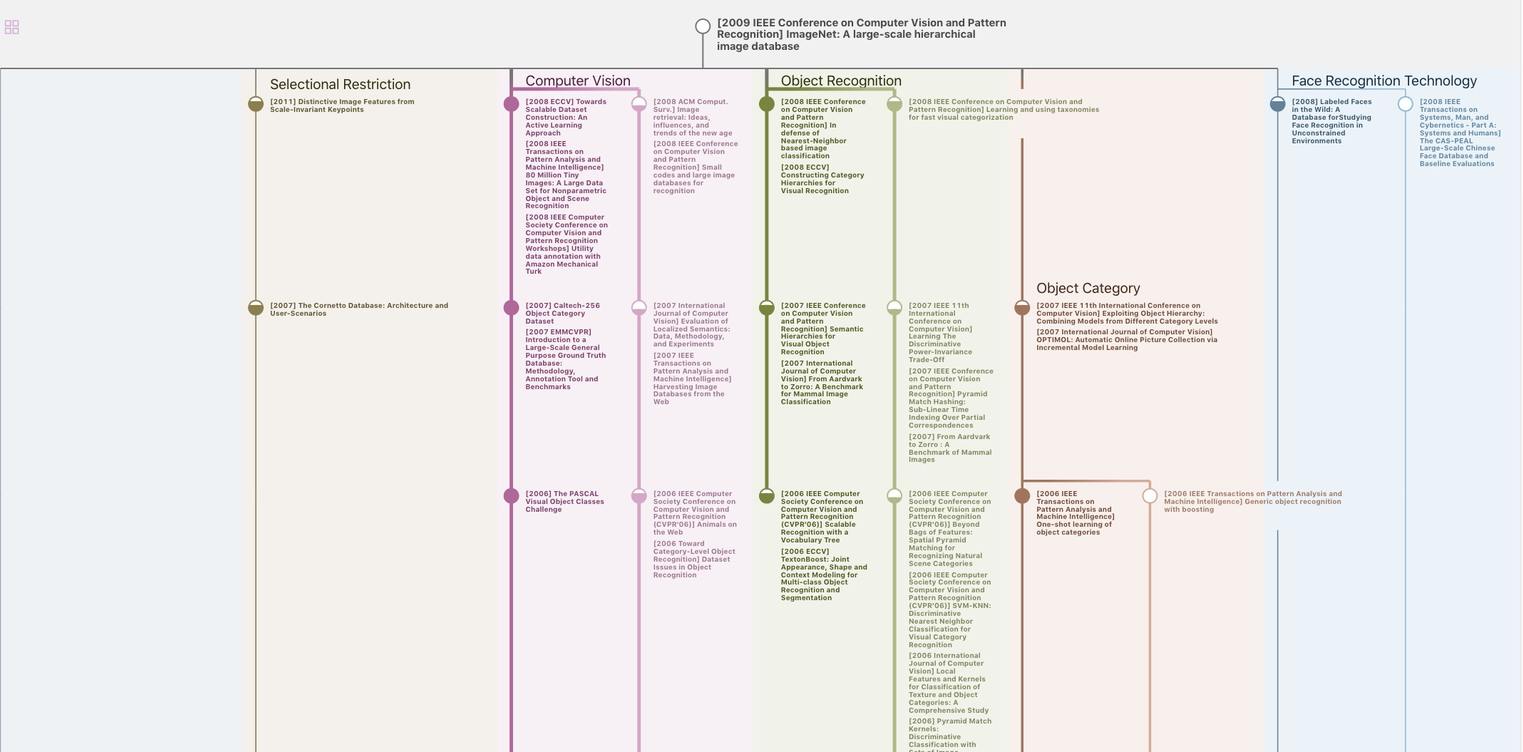
生成溯源树,研究论文发展脉络
Chat Paper
正在生成论文摘要