Active aging prediction from muscle electrical activity using HD-sEMG signals and machine learning
2023 IEEE 36TH INTERNATIONAL SYMPOSIUM ON COMPUTER-BASED MEDICAL SYSTEMS, CBMS(2023)
摘要
In this study, using High-Density Surface Electromyography (HD-sEMG) signals and extracted features, we propose a Machine Learning (ML) architecture for predicting active aging through motor functional age (MFA) estimation, a new concept introduced by Prof. S. Boudaoud and Prof. K. Kinugawa in EIT-Health CHRONOS project. In fact, MFA should be equal to Chronological Age (CA) for active subjects. For this purpose, several time and frequency features have been tested, coupled to classical ML classifiers, to predict active aging through MFA estimation. The classifiers have been trained on the CHRONOS database composed by 79 active subjects (International Physical Activity Questionnaire (IPAQ) : 2-3, from 25 to 74 yrs) and we tested, as a preliminary study, 4 sedentary subjects (IPAQ: 1, 45-54 yrs). The results show that the best classifier gave promising results (Accuracy = 71.6% +/- 3.86) for confirming active aging (MFA=CA) for active tested subjects and predicting sedentary aging (MFA>CA) for some sedentary subjects. This preliminary results have to be confirmed in future studies on larger cohort.
更多查看译文
关键词
machine learning,classification,aging,HD-sEMG
AI 理解论文
溯源树
样例
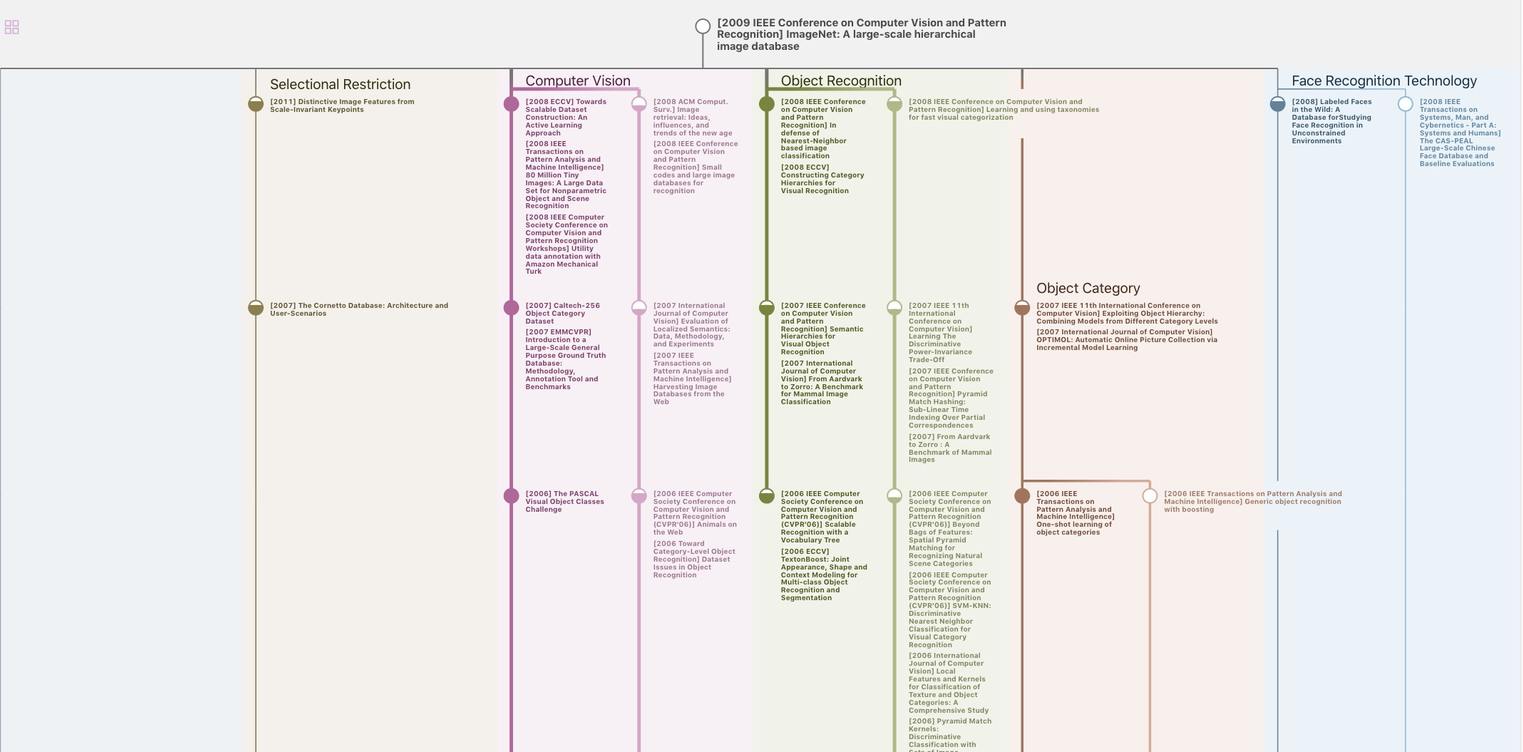
生成溯源树,研究论文发展脉络
Chat Paper
正在生成论文摘要