Online Shipping Container Pricing Strategy Achieving Vanishing Regret with Limited Inventory.
ICDE(2023)
摘要
With the growing demand for global trade transportation, the shipping container market has gained an increasingly important position. As a key issue of the market, container pricing is regarded as an important indicator to adjust the market supply and demand as well as the revenue of liner enterprises. Although various methods aimed at increasing enterprise revenue, such as expert pricing and dynamic pricing, have been proposed by industry and academia in recent years, these approaches rarely yield worst-case performance guarantee for the double-sided online scenarios of commodities and buyers.To cater to the double-sided online scenario and provide theoretical performance guarantee, we propose an online learning-based pricing framework named Balancing Inventory and Revenue with -chasing Decider (BIRD). BIRD determines container price by combining advantages of given multiple online pricing strategies. We utilize a strategy selector A to select a proper target strategy and use an ϵ-chasing decider ${{{\mathfrak{D}}}^{Cha\operatorname{s} ing}}$ to determine the price. BIRD is proven to combine the advantages of multiple online pricing strategies to achieve the performance close to the posterior optimal strategy for any sequence of online buyers on realistic sales platforms with inventory limitation. BIRD is proved to yield a vanishing regret for the online posted pricing problem with the features of limited inventory and multi-unit demand. Based on the historical data provided by COSCO, one of the largest liner enterprises in the world, we experimentally demonstrate the effectiveness of the proposed algorithm.
更多查看译文
关键词
Posted pricing,shipping container,limited inventory,multi-unit purchase,online learning,vanishing regret
AI 理解论文
溯源树
样例
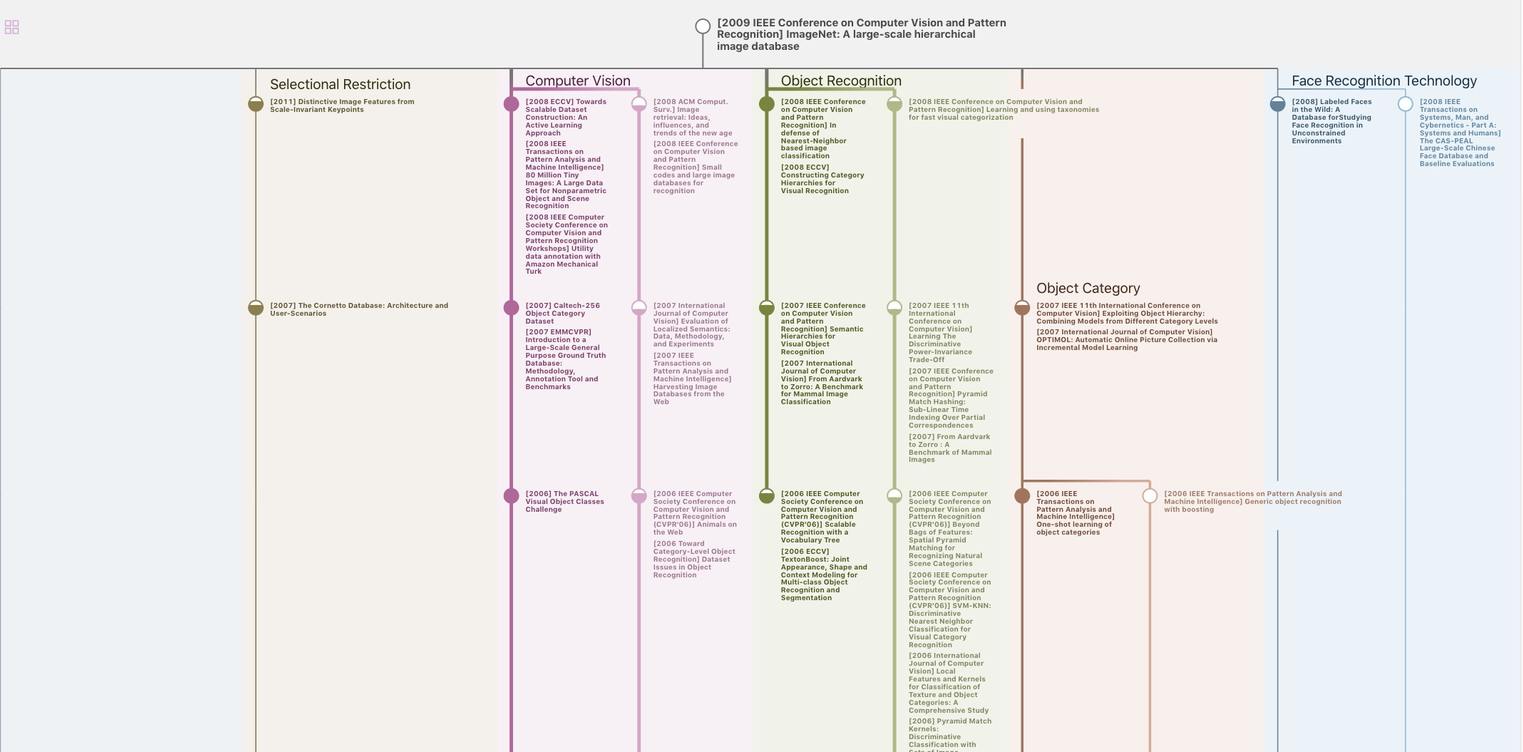
生成溯源树,研究论文发展脉络
Chat Paper
正在生成论文摘要