Efficient Cross Dynamic Task Assignment in Spatial Crowdsourcing.
ICDE(2023)
摘要
As a novel intelligent sensing paradigm, spatial crowdsourcing has received extensive attention. Task assignment is a key issue in spatial crowdsourcing. In practice, tasks are unevenly distributed in time and space. Accordingly, the problem of cross task assignment attracts growing attention in both industry and academia. Although there has been a research on this problem, it focuses only on maximizing total revenues for inner platforms. Therefore, it can also be improved to bring a multi-win situation for outer workers and task requesters as well as the inner platform. Inspired by this, we first formulate a new cross dynamic task assignment (CDTA) problem by introducing the reputation scores of workers, and prove it to be NP-hard. For the CDTA problem, a hybrid batch-based framework is presented on the basis of a new cross-platform incentive mechanism and a hybrid batch processing strategy, which are efficient in solving the problem of uneven spatial and time distribution of tasks, respectively. After that, a KM-based algorithm and a density-aware greedy algorithm are proposed to gain an accurate assignment result of tasks in each batch and good performance, respectively. Furthermore, the CDTA problem is modeled as a potential game that is proven to have at least a pure Nash Equilibrium theoretically. Last but not least, a game-theoretic approach is developed to maximize the revenues of the inner platform and outer workers at the same time. Extensive experiments on both real and synthetic datasets are conducted to demonstrate the effectiveness and efficiency of the proposed algorithms.
更多查看译文
关键词
Game Theory,Spatial Crowdsourcing,Task Assignment
AI 理解论文
溯源树
样例
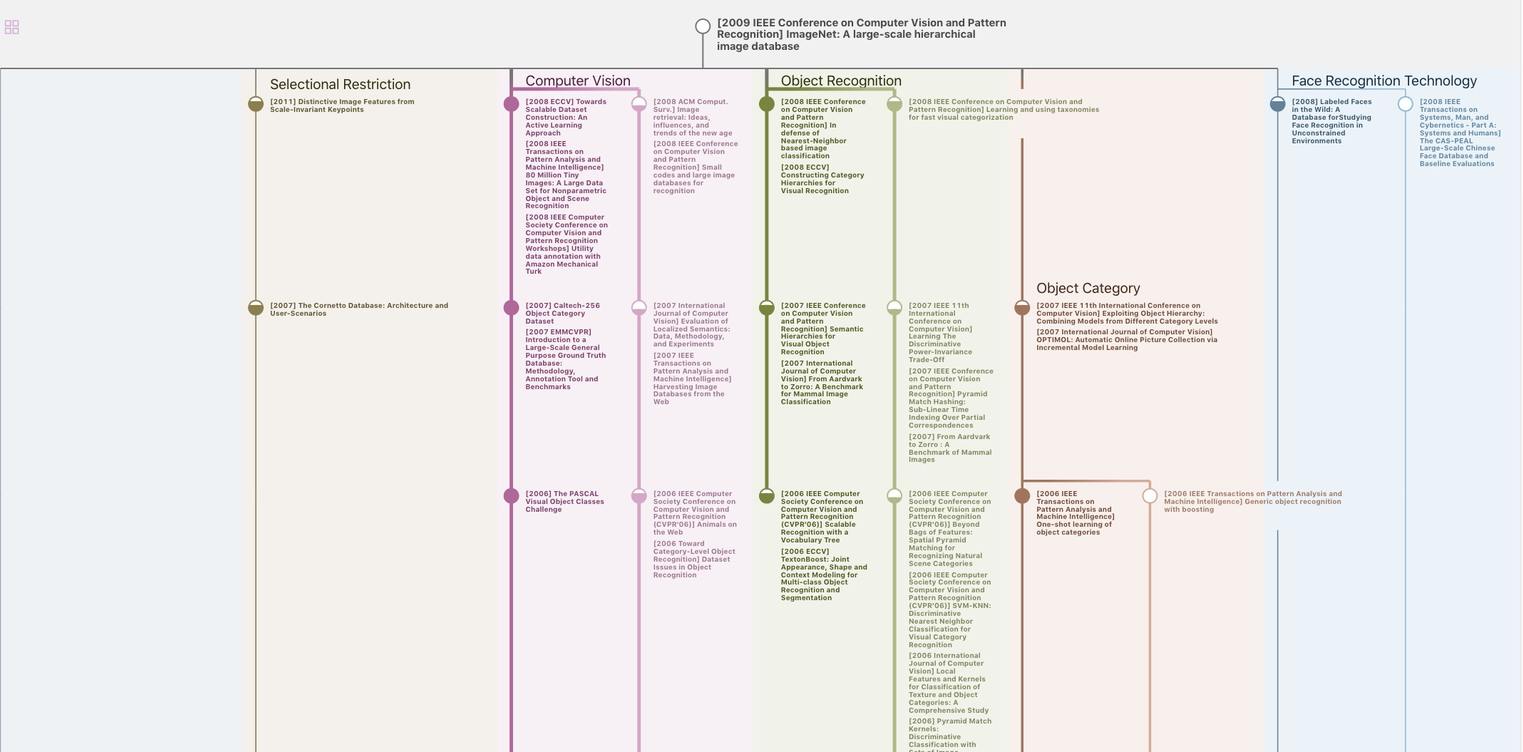
生成溯源树,研究论文发展脉络
Chat Paper
正在生成论文摘要