Towards Realistic 3D Ultrasound Synthesis: Deformable Augmentation using Conditional Variational Autoencoders
2023 IEEE 36TH INTERNATIONAL SYMPOSIUM ON COMPUTER-BASED MEDICAL SYSTEMS, CBMS(2023)
摘要
For training deep neural networks, large data sets are required. Especially in the medical 3D ultrasound (US) image domain, the amount of available data is limited. A common method to enlarge small data sets is using data augmentation. However, simple geometric augmentation techniques like rotation or sheering can lead to unrealistic US images. In this study, a novel structure-dependent deformable augmentation method for 3D US patches is proposed. The approach is based on learning realistic motion patterns from 3D liver US images using a conditional variational autoencoder (CVAE) where the condition represents the anatomical structure type. The CVAE augmentation performance is compared to a baseline variational autoencoder (VAE). It is shown that the CVAE generates deformable augmentations 18.5 percentage points more similar to realistic deformations than the VAE. Furthermore, the method is evaluated in an expert study where experienced radiologists were asked to rate the realism of US patches. The results show that the experts could not distinguish between CVAE augmented and original US patches. Applying the proposed method in a target detection application improved the performance of a neural network by 41 %. The proposed augmentation method is able to apply realistic deformation to 3D US patches which can be used to enlarge a small data set for usage in deep learning applications.
更多查看译文
关键词
Deep learning,representation learning,data augmentation
AI 理解论文
溯源树
样例
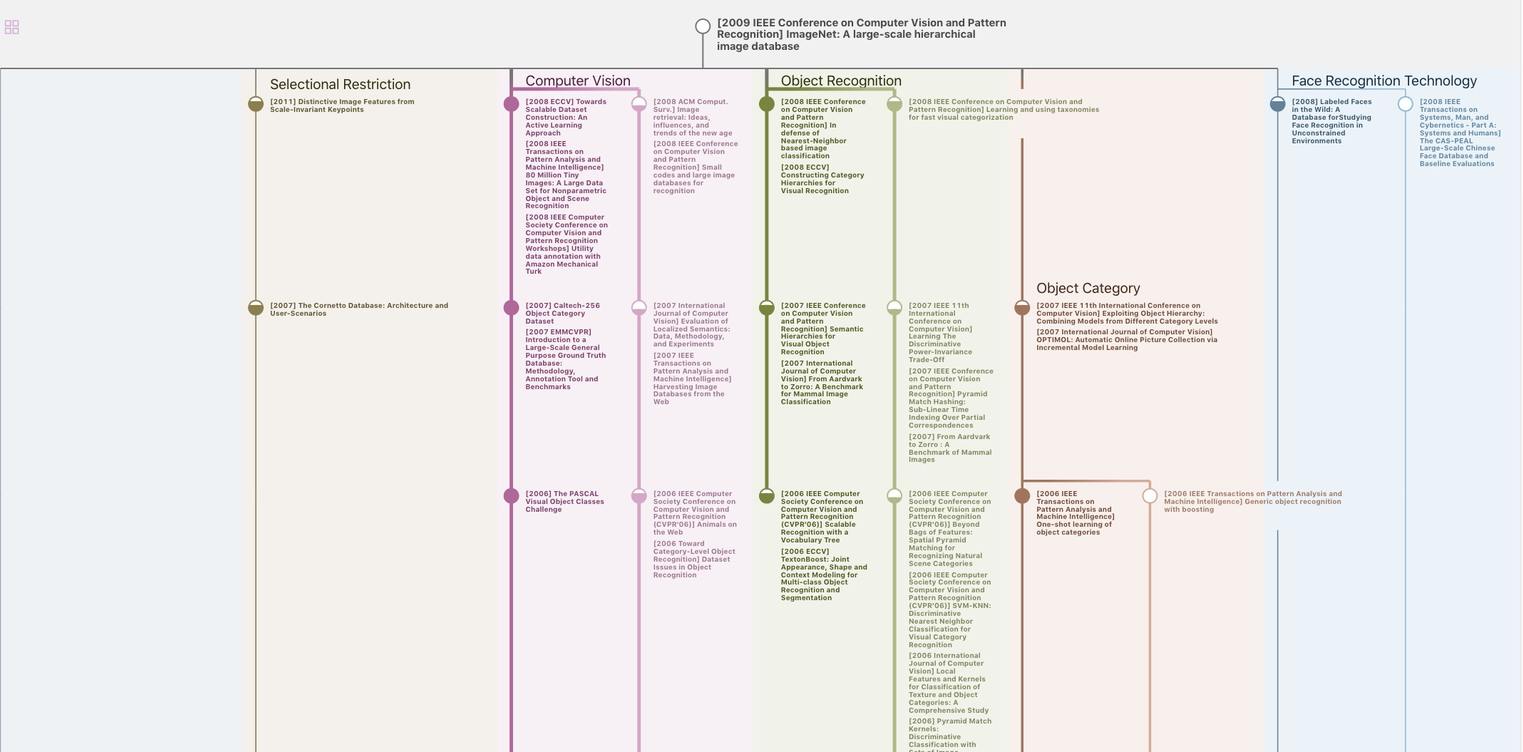
生成溯源树,研究论文发展脉络
Chat Paper
正在生成论文摘要