Towards Explainable Table Interpretation Using Multi-view Explanations.
ICDE(2023)
Abstract
Table interpretation (TI), which aims to predict the column types and relations of tables, plays an essential role in necessary decision-making actions for data management systems. Typically, TI is followed by a manual verification, where experts manually verify the correctness of TI’s predictions. Manual verification is able to ensure the quality of decision-making actions but labor-intensive. To reduce the labour costs, providing explanations for TI’s predictions is necessary as these explanations can help them do faster and more accurate verification. However, existing TI approaches overlook the manual verification process and lack explainability
1
. To fill this gap, this paper explores the challenging explainable table interpretation problem, which aims to provide faithful explanations and meanwhile achieve high prediction performance. Furthermore, we propose ExplainTI framework. ExplainTI consists of two phases: (i) tables are converted to sequences and lightweight column graphs; and (ii) a pre-trained transformer encoder is fine-tuned to provide multi-view explanations and aggregate contextual information. Extensive experiments on both real Web tables and database tables confirm that ExplainTI outperforms competitive baselines. Moreover, systematical analysis of explainability demonstrates that our framework can provide faithful explanations to facilitate the manual verification process.
MoreTranslated text
Key words
Table Interpretation,Explainability
AI Read Science
Must-Reading Tree
Example
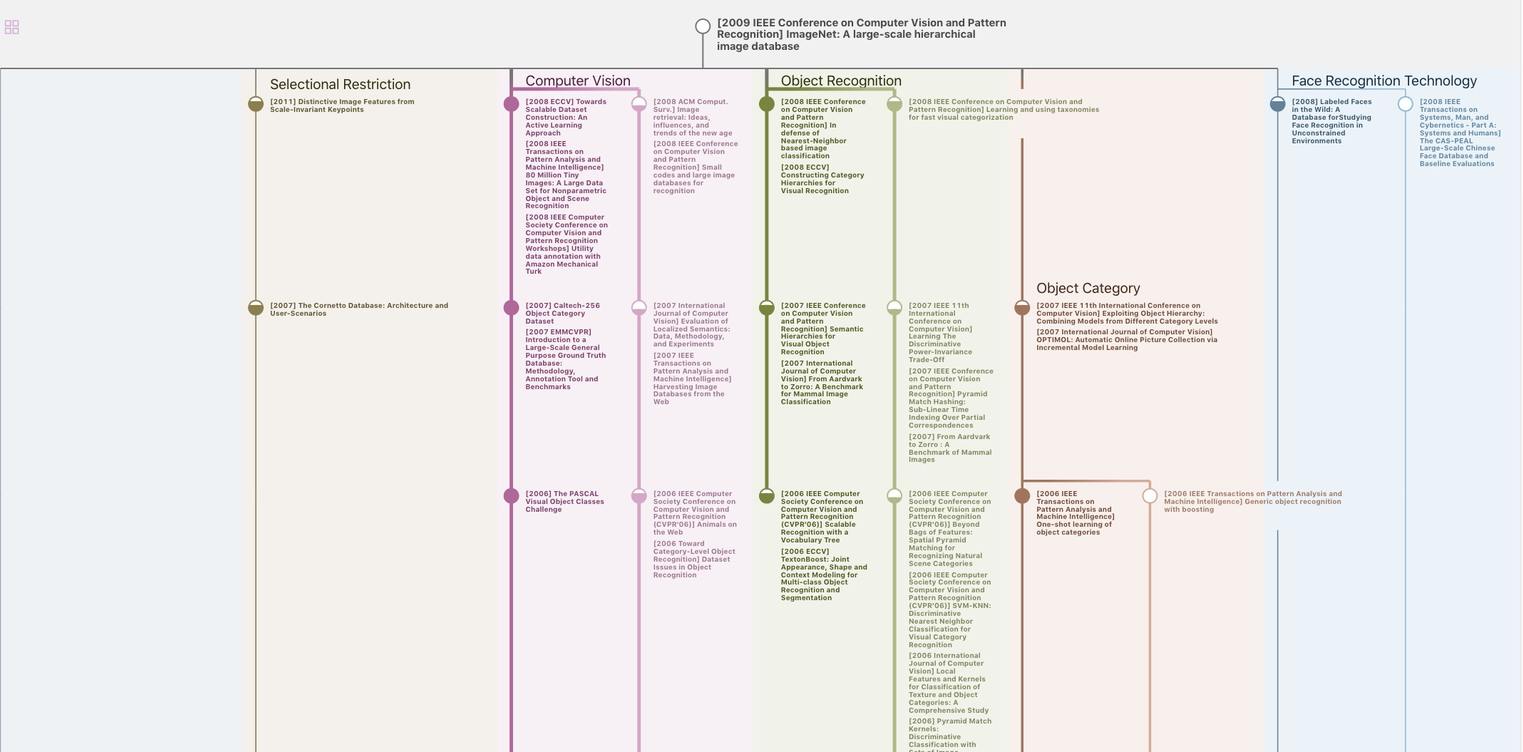
Generate MRT to find the research sequence of this paper
Chat Paper
Summary is being generated by the instructions you defined