Enhancing the Efficiency of UAV Swarms Communication in 5G Networks through a Hybrid Split and Federated Learning Approach
IWCMC(2023)
摘要
The integration of unmanned aerial vehicles (UAVs) with 5G networks presents a promising opportunity to revolutionize wireless communication and provide high-speed internet access to remote areas. Nevertheless, the vast quantity of data generated by UAVs requires the implementation of efficient distributed learning techniques. In this study, we present a novel hybrid approach that merges Federated Learning (FL) and Split Learning (SL) to optimize the performance of UAV swarms in 5G networks. While FL is capable of reducing communication overhead and preserving privacy, SL can enhance the accuracy of the model through the utilization of the local computational resources of each device. To realize the hybrid approach, we first locally train the model on each UAV using split learning. Subsequently, the encrypted model parameters are transmitted to a central server for federated averaging. Finally, the updated model is dispatched back to each UAV for local fine-tuning, and this cycle is repeated until convergence is achieved. The hybrid approach capitalizes on the strengths of both FL and SL to minimize communication overhead and increase accuracy. To tackle the challenge of selecting the most suitable UAVs for participation in the learning process, we propose a multiagent algorithm that considers factors such as communication latency and training time. Our experimental results indicate that the proposed approach leads to substantial improvements in communication overhead and accuracy compared to conventional methods.
更多查看译文
关键词
UAV swarms,split learning,federated learning,user selection,multi-agent reinforcement learning
AI 理解论文
溯源树
样例
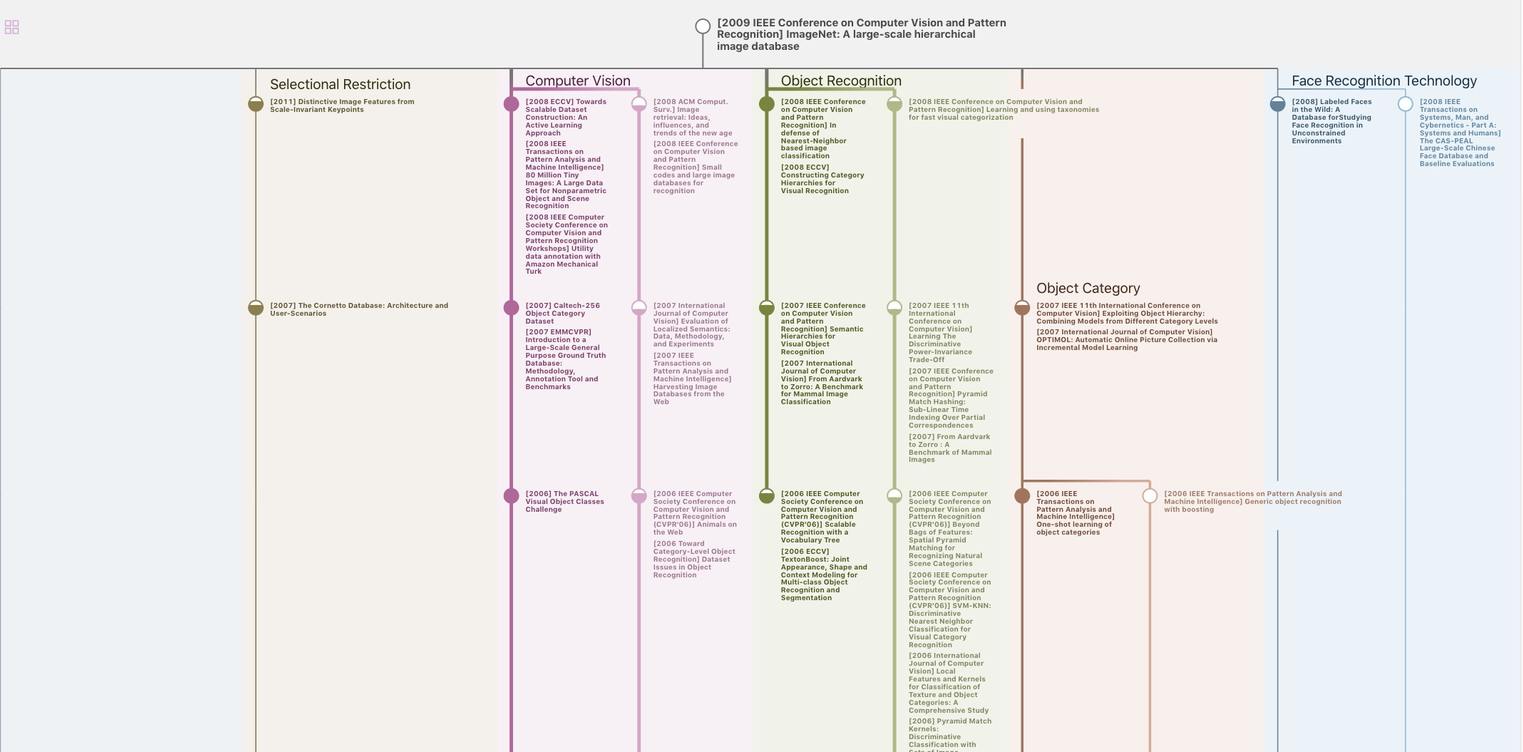
生成溯源树,研究论文发展脉络
Chat Paper
正在生成论文摘要