A Reinforcement Learning Based Approach for Controlling Autonomous Vehicles in Complex Scenarios
IWCMC(2023)
摘要
Autonomous driving has gained an increased interest in both academia and industry, as autonomous vehicles (AVs) significantly improve road safety by reducing traffic accidents and human injuries. Motion control remains one of the main functions of Autonomous Vehicles, which generates the steering angle and velocity of the vehicle. While traditional Machine Learning techniques have been extensively used in the past to improve motion control in AVs, the attention has been recently drawn to the use of Deep Learning (DL) and Deep Reinforcement Learning (DRL) techniques. These techniques have been applied to improve motion control of AVs and to help them learn from their environment. However, existing works are limited to dealing with simple scenarios without taking into consideration other road participants (e.g., other vehicles, pedestrians, cyclists, and motorcycles). In this paper, we propose a DRL-based model using Deep-Q Networks to control the AV in a complex scenario with dense traffic involving road participants. The AV learns the policy of different actions to reach its destination in an intersection without accidents. We tested and validated our proposed approach using the CARLA simulator. The obtained results demonstrated the efficiency of our solution by achieving better learning in terms of travel delay and avoiding collisions.
更多查看译文
关键词
Autonomous Vehicles,Reinforcement Learning,Vehicle Control
AI 理解论文
溯源树
样例
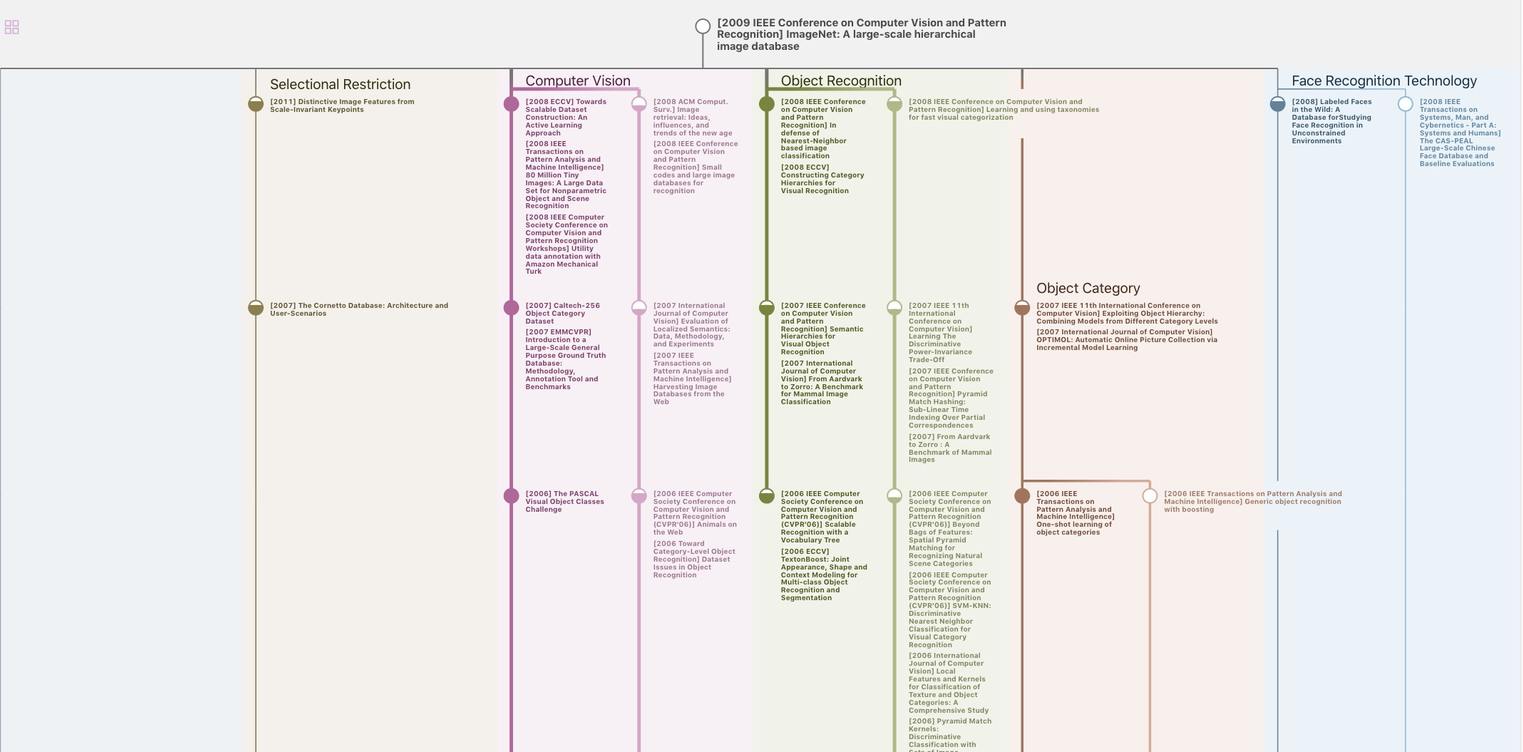
生成溯源树,研究论文发展脉络
Chat Paper
正在生成论文摘要