Factor Graph-based Deep Reinforcement Learning for Path Selection Scheme in Full-duplex Wireless Multihop Networks
2023 INTERNATIONAL WIRELESS COMMUNICATIONS AND MOBILE COMPUTING, IWCMC(2023)
摘要
Wireless Multihop Network (WMN) is set of wirelessly connected nodes without an aid of centralized infrastructure that can forward any message via relaying nodes by multihop fashion. In WMN, there are still some issues that need to be resolved, like due to the uncertainty of source node choosing a path to send the message and the nature of multihop fashion, the performance of network capacity can degrade drastically. To solve these problems, in this research we propose two novel path selection algorithms called SNR-based learning path selection (NLPS) algorithm and SINR-based learning path selection (INLPS) algorithm, which are incorporated with the deep reinforcement learning (DRL) to select the best multihop path from source node to destination node with highest end-to-end throughput. Factor graph (FG) representation is used to reduce the computation time. Our simulation results reveal that both NLPS and INLPS can achieve high network capacity and moderate computation time. Meanwhile, nested lattice code (NLC) is used in compute-and-forward strategy to reduce the time slots. As a result, the network capacity can increase more.
更多查看译文
关键词
Deep Reinforcement Learning,Factor Graph,Nested Lattice Code,Learning Path Selection Algorithm,Wireless Multihop Networks
AI 理解论文
溯源树
样例
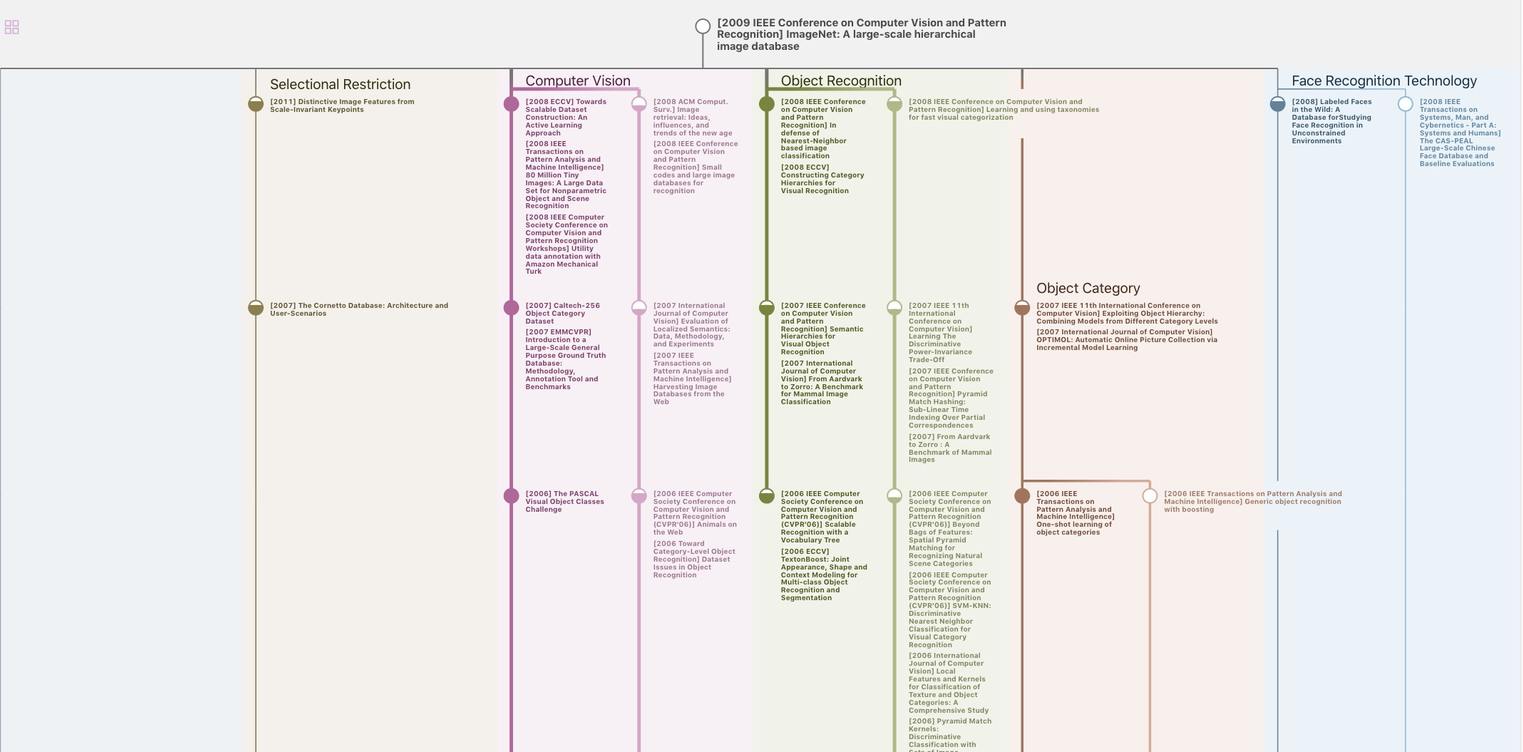
生成溯源树,研究论文发展脉络
Chat Paper
正在生成论文摘要