Existence of a Unique Invariant Measure and Ergodic Property in AIMD-based Multi-resource Allocation
2023 AMERICAN CONTROL CONFERENCE, ACC(2023)
摘要
Distributed resource allocation arises in many application domains, such as smart energy systems, intelligent transportation systems, cloud computing, edge computing, etcetera. To realize many of these applications, agents in a network may require multiple shared resources to complete a task and aim to maximize the network utility. Additionally, they may demand resources based on their preferences. Furthermore, they may not wish to share their cost functions, partial derivatives of the cost functions, etc., with other agents or a central server; however, they share their resource demands with the central server that aggregates the demands and sends onebit resource-capacity constraint notification in the network. The single-resource allocation algorithms are inefficient and provide sub-optimal solutions for multi-resource allocations, especially when the cost functions are multi-variate and non-separable. We present additive increase and multiplicative decrease algorithm (AIMD)-based distributed solutions for multi-resource allocation. We formulate the resource allocations problem over finite window sizes and model the system as a homogeneous Markov chain with place-dependent probabilities. We show that the time-averaged allocations over the finite window size converge to a unique invariant measure. We also show that the ergodic property holds for the model.
更多查看译文
关键词
Distributed optimization,AIMD,multiresource allocation,Ergodicity,Invariant measure
AI 理解论文
溯源树
样例
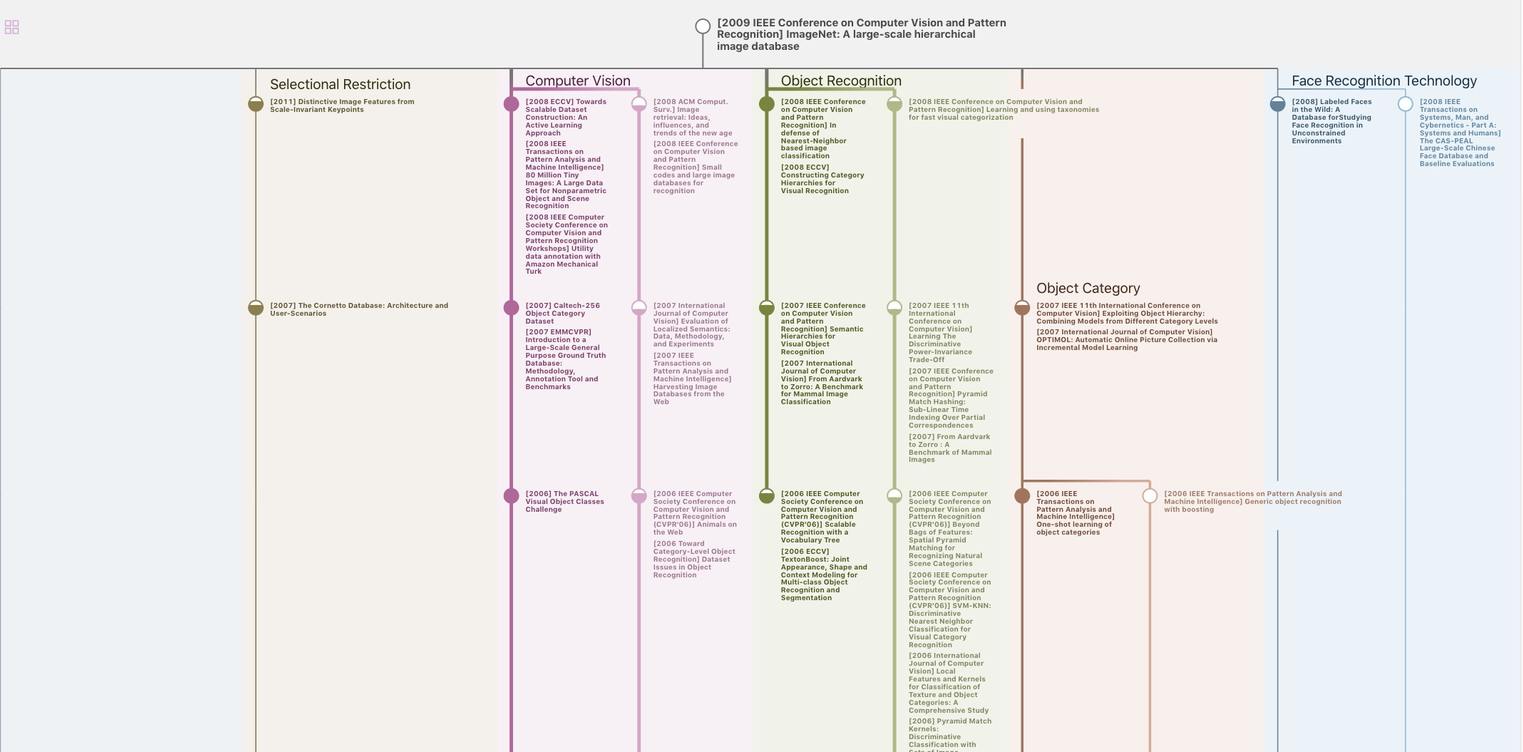
生成溯源树,研究论文发展脉络
Chat Paper
正在生成论文摘要