CXL-ANNS: Software-Hardware Collaborative Memory Disaggregation and Computation for Billion-Scale Approximate Nearest Neighbor Search.
USENIX Annual Technical Conference(2023)
摘要
We propose CXL-ANNS, a software-hardware collaborative approach to enable highly scalable approximate nearest neighbor search (ANNS) services. To this end, we first disaggregate DRAM from the host via compute express link (CXL) and place all essential datasets into its memory pool. While this CXL memory pool can make ANNS feasible to handle billion-point graphs without an accuracy loss, we observe that the search performance significantly degrades because of CXL's far-memory-like characteristics. To address this, CXL-ANNS considers the node-level relationship and caches the neighbors in local memory, which are expected to visit most frequently. For the uncached nodes, CXL-ANNS prefetches a set of nodes most likely to visit soon by understanding the graph traversing behaviors of ANNS. CXL-ANNS is also aware of the architectural structures of the CXL interconnect network and lets different hardware components therein collaboratively search for nearest neighbors in parallel. To improve the performance further, it relaxes the execution dependency of neighbor search tasks and maximizes the degree of search parallelism by fully utilizing all hardware in the CXL network. Our empirical evaluation results show that CXL-ANNS exhibits 111.1x higher QPS with 93.3% lower query latency than state-of-the-art ANNS platforms that we tested. CXL-ANNS also outperforms an oracle ANNS system that has DRAM-only (with unlimited storage capacity) by 68.0% and 3.8x, in terms of latency and throughput, respectively.
更多查看译文
AI 理解论文
溯源树
样例
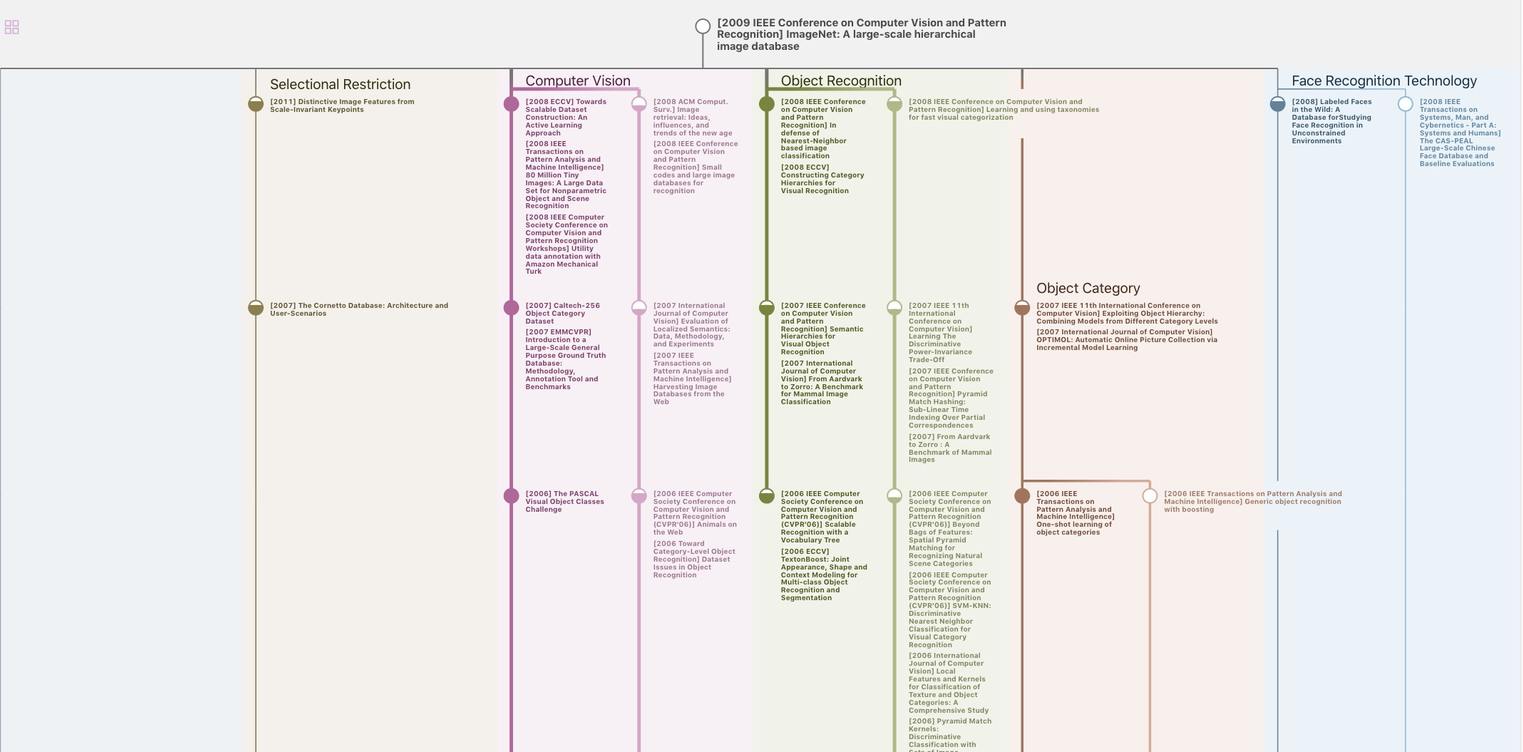
生成溯源树,研究论文发展脉络
Chat Paper
正在生成论文摘要