From Open Loop to Real-time Recipe Optimization for Complex Industrial Batch Processes
ACC(2023)
摘要
We propose a fundamental-model-based optimization framework for open-loop and real-time batch recipe optimization using mathematical programing (MP) and reinforcement learning (RL). The dynamic fundamental model gives rise to a complex nonlinear differential algebraic equations (DAE) system. We introduce a decomposition-based initialization algorithm for solving the large-scale nonlinear program (NLP) resulting from the discretization of the DAE system. The proposed MP and RL-based approaches are implemented to optimize the recipe of a semi-batch process in the Dow Chemical Company. For open-loop optimization, we find the optimal profiles of two input variables and the batch length that maximize average profit. For real time optimization, we train the RL agent using the fundamental model with uncertainties. The trained agent can interact with the actual process and provide control actions in real time.
更多查看译文
关键词
batch length,complex industrial batch processes,complex nonlinear differential algebraic equations system,DAE system,decomposition-based initialization algorithm,dynamic fundamental model,fundamental-model-based optimization framework,large-scale nonlinear program,open-loop optimization,optimal profiles,real-time batch recipe optimization,real-time recipe optimization,reinforcement learning,RL agent,RL-based approaches,semibatch process,time optimization
AI 理解论文
溯源树
样例
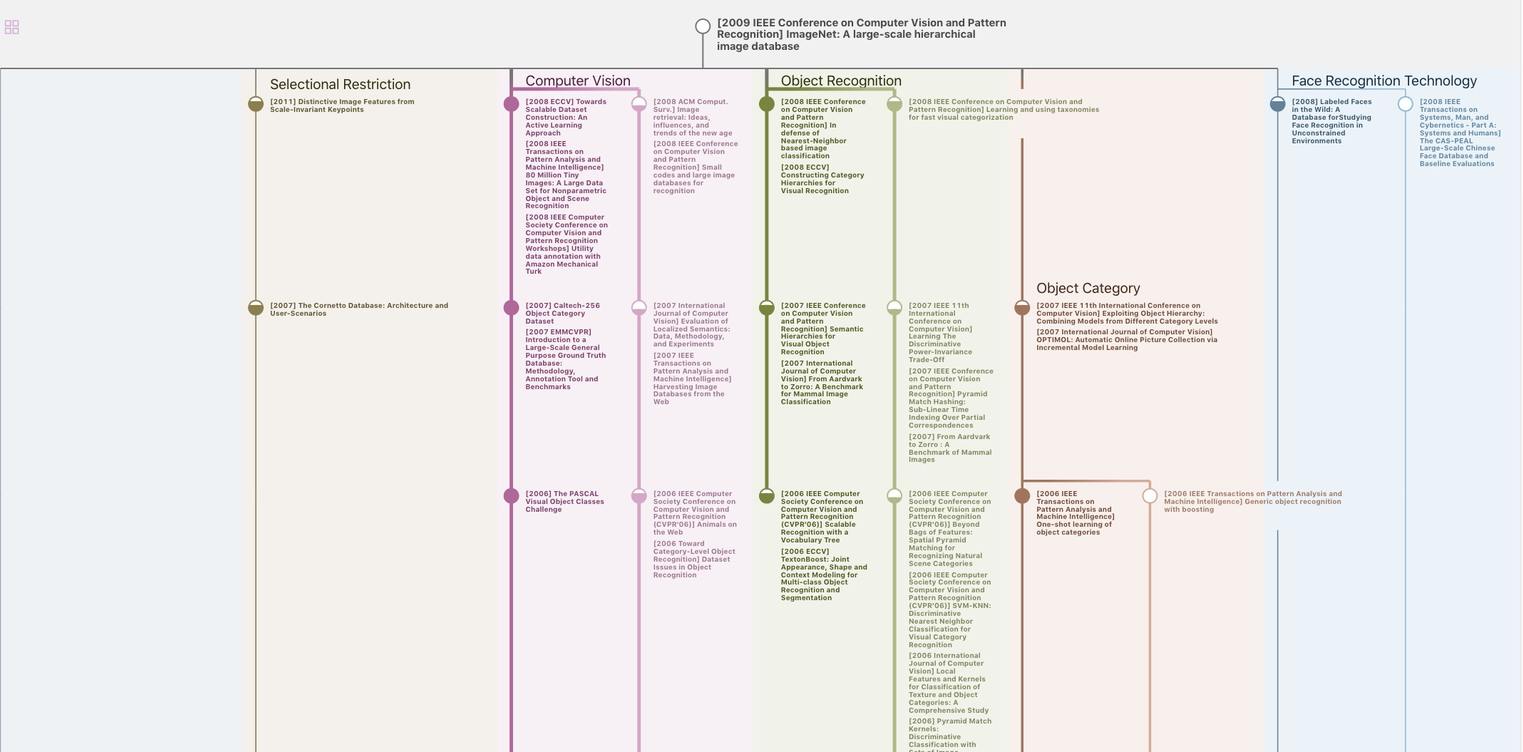
生成溯源树,研究论文发展脉络
Chat Paper
正在生成论文摘要