On the Complexity and Approximability of Optimal Sensor Selection for Mixed-Observable Markov Decision Processes
2023 AMERICAN CONTROL CONFERENCE, ACC(2023)
摘要
Mixed-Observable Markov Decision Processes (MOMDPs) are used to model systems where the state space can be decomposed as a product space of a set of state variables, and the controlling agent is able to measure only a subset of those state variables. In this paper, we consider the setting where we have a set of potential sensors to select for the MOMDP, where each sensor measures a certain state variable and has a selection cost. We formulate the problem of selecting an optimal set of sensors for MOMDPs (subject to certain budget constraints) to maximize the expected infinite-horizon reward of the agent and show that this sensor placement problem is NP-Hard, even when one has access to an oracle that can compute the optimal policy for any given instance. We then study a greedy algorithm for approximate optimization and show that there exist instances of the MOMDP sensor selection problem where the greedy algorithm can perform arbitrarily poorly. Finally, we provide some empirical results of greedy sensor selection over randomly generated MOMDP instances and show that, in practice, the greedy algorithm provides near-optimal solutions for many cases, despite the fact that one cannot provide general theoretical guarantees for its performance. In total, our work establishes fundamental complexity results for the problem of optimal sensor selection (at design-time) for MOMDPs.
更多查看译文
AI 理解论文
溯源树
样例
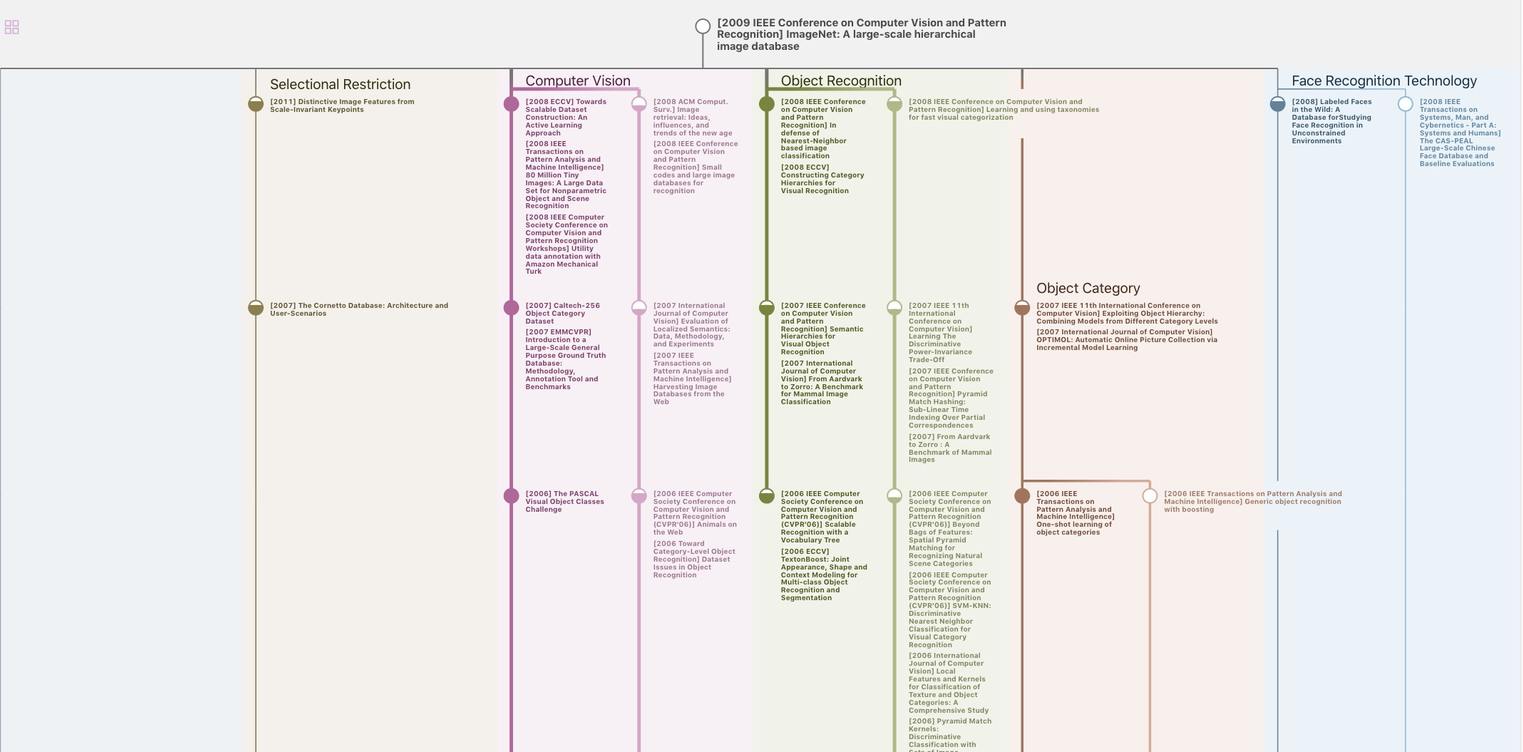
生成溯源树,研究论文发展脉络
Chat Paper
正在生成论文摘要