Graduated Moving Window Optimization as a Flexible Framework for Multi-Object Tracking
2023 AMERICAN CONTROL CONFERENCE, ACC(2023)
摘要
Continuous optimization methods for multiple object tracking allow to jointly estimate continuous object trajectories and perform implicit data association. However, the local minima that arise from including data association in a continuous optimization problem pose challenges. In addition, optimization is usually performed either over a fixed or an indefinitely growing time frame. This either discards valuable past information or is computationally unsustainable. Hence, in this work, a flexible continuous optimization based framework for multiple object tracking that accounts for these issues is proposed. The framework provides a unified approach to not only include data association, but also multiple motion models and temporary interactions between objects in a continuous optimization problem. It leverages the concept of graduated optimization, a heuristic, which allows avoiding local minima. The proposed framework's performance is benchmarked on a synthetic dataset, showing its capabilities and indicating areas of possible improvement.
更多查看译文
AI 理解论文
溯源树
样例
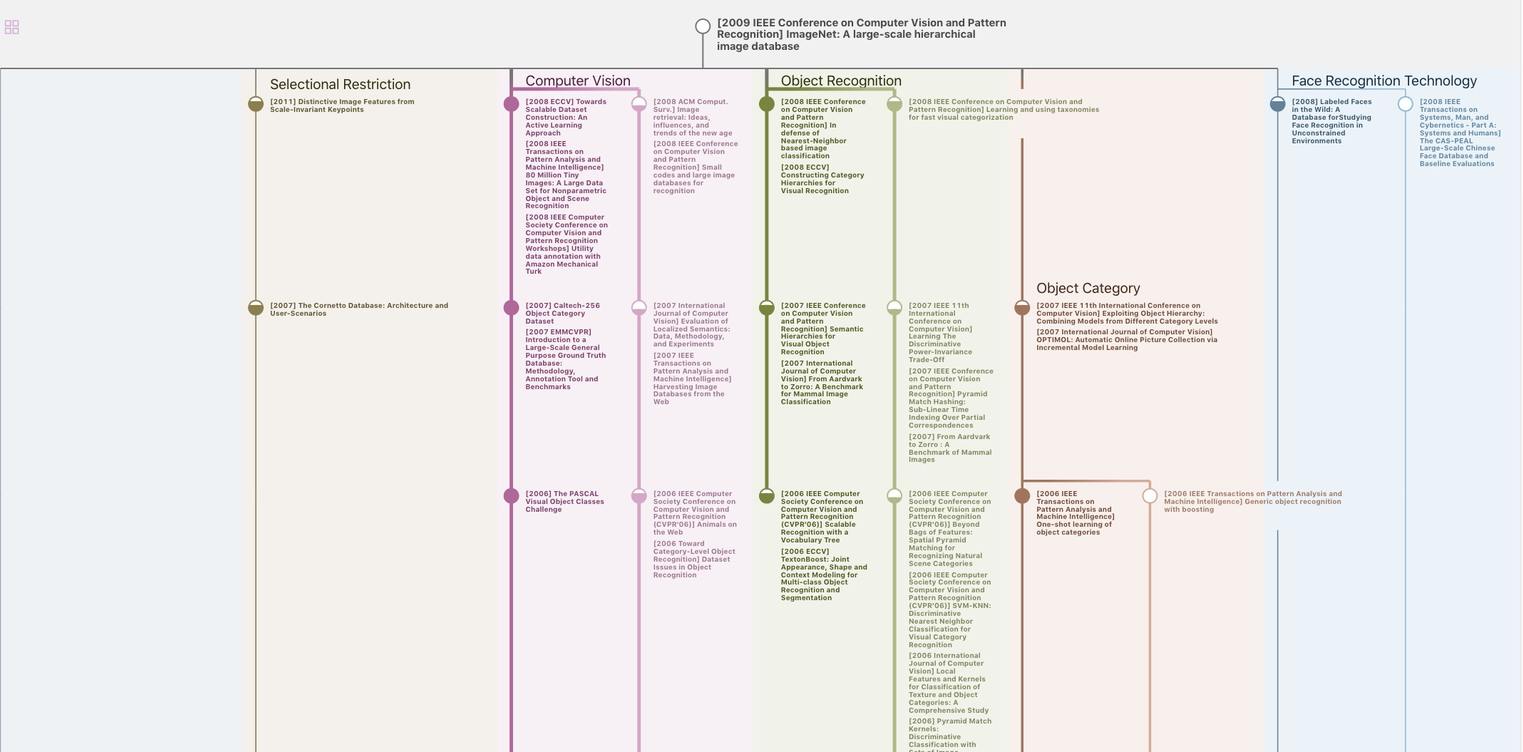
生成溯源树,研究论文发展脉络
Chat Paper
正在生成论文摘要