Re-thinking computation offload for efficient inference on IoT devices with duty-cycled radios
MobiCom(2023)
摘要
While a number of recent efforts have explored the use of "cloud offload" to enable deep learning on IoT devices, these have not assumed the use of duty-cycled radios like BLE. We argue that radio duty-cycling significantly diminishes the performance of existing cloud-offload methods. We tackle this problem by leveraging a previously unexplored opportunity to use early-exit offload enhanced with prioritized communication, dynamic pooling, and dynamic fusion of features. We show that our system, FLEET, achieves significant benefits in accuracy, latency, and compute budget compared to state-of-art local early exit, remote processing, and model partitioning schemes across a range of DNN models, datasets, and IoT platforms.
更多查看译文
关键词
edge computing, cloud computing, computation off-loading, deep neural networks
AI 理解论文
溯源树
样例
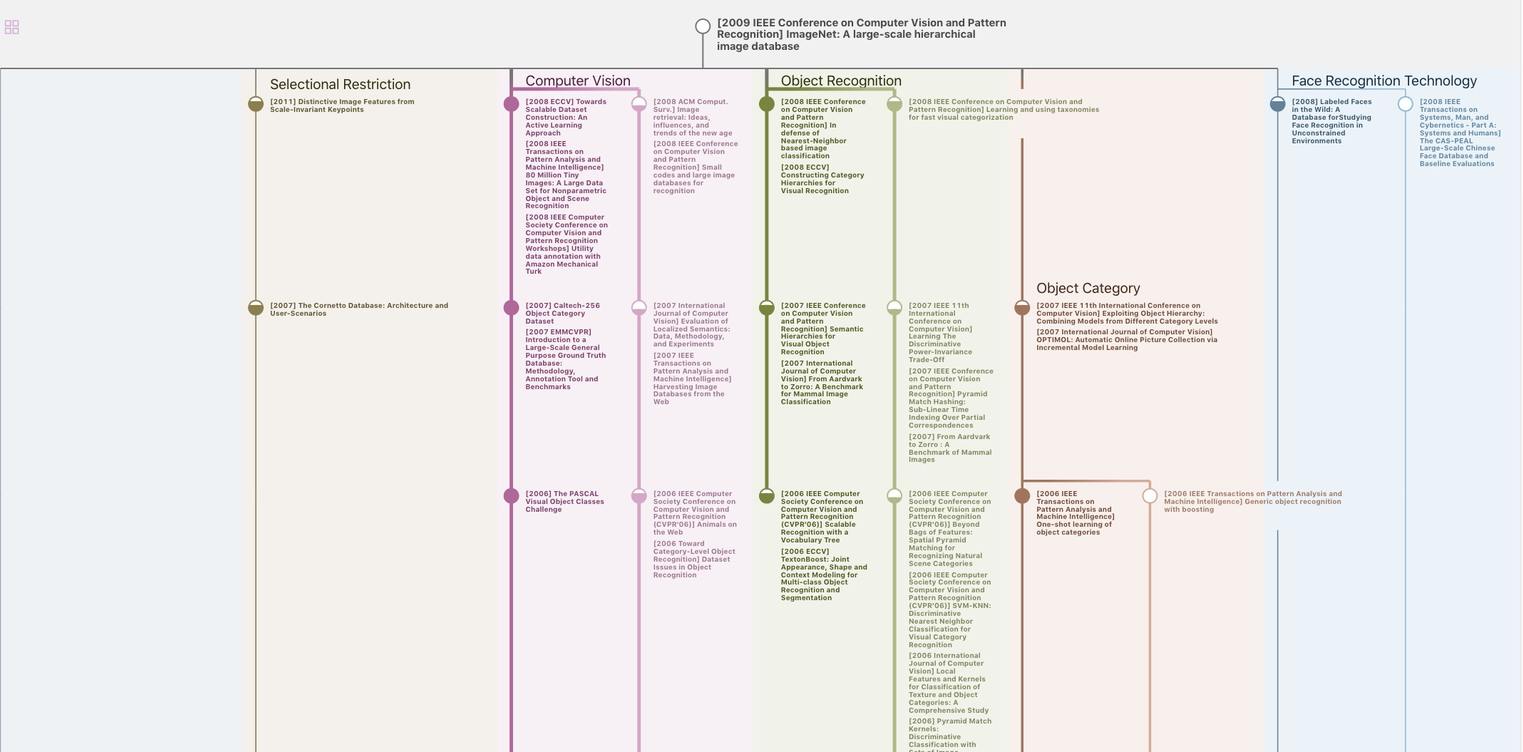
生成溯源树,研究论文发展脉络
Chat Paper
正在生成论文摘要