Assessing Driver Task Engagement Through Machine Learning Classification of Physiological Response
2023 IEEE SENSORS APPLICATIONS SYMPOSIUM, SAS(2023)
摘要
As autonomous driving features become more prevalent in the automotive industry, the need to assess driver engagement becomes crucial to ensure safety and facilitate a smooth transition from human-controlled to autonomous driving. Until level 5 autonomous vehicles (AVs) have achieved full adoption in the automobile market, drivers will still be required to supervise and potentially take over control of their AVs. While this is the case, drivers need to maintain an appropriate level of engagement, even when they are not driving. The following study outlines a method to apply ambient sensors to measure drivers' physiological states. The measurements collected by the ambient sensors were converted to time series metrics. These metrics were then analyzed by machine learning (ML) methods to classify drivers as driving in either a low or high engagement driving situation, where engagement was moderated by the introduction of a surprise event. AI explainability methods were used in conjunction with the ML models to understand the key physiological factors that contributed to understanding a driver's level of engagement. This research contributes to the advancement of sensor-based monitoring systems for driver engagement, which can enhance safety in the transportation sector.
更多查看译文
关键词
driver engagement measurement,autonomous vehicles,physiological measurements,machine learning
AI 理解论文
溯源树
样例
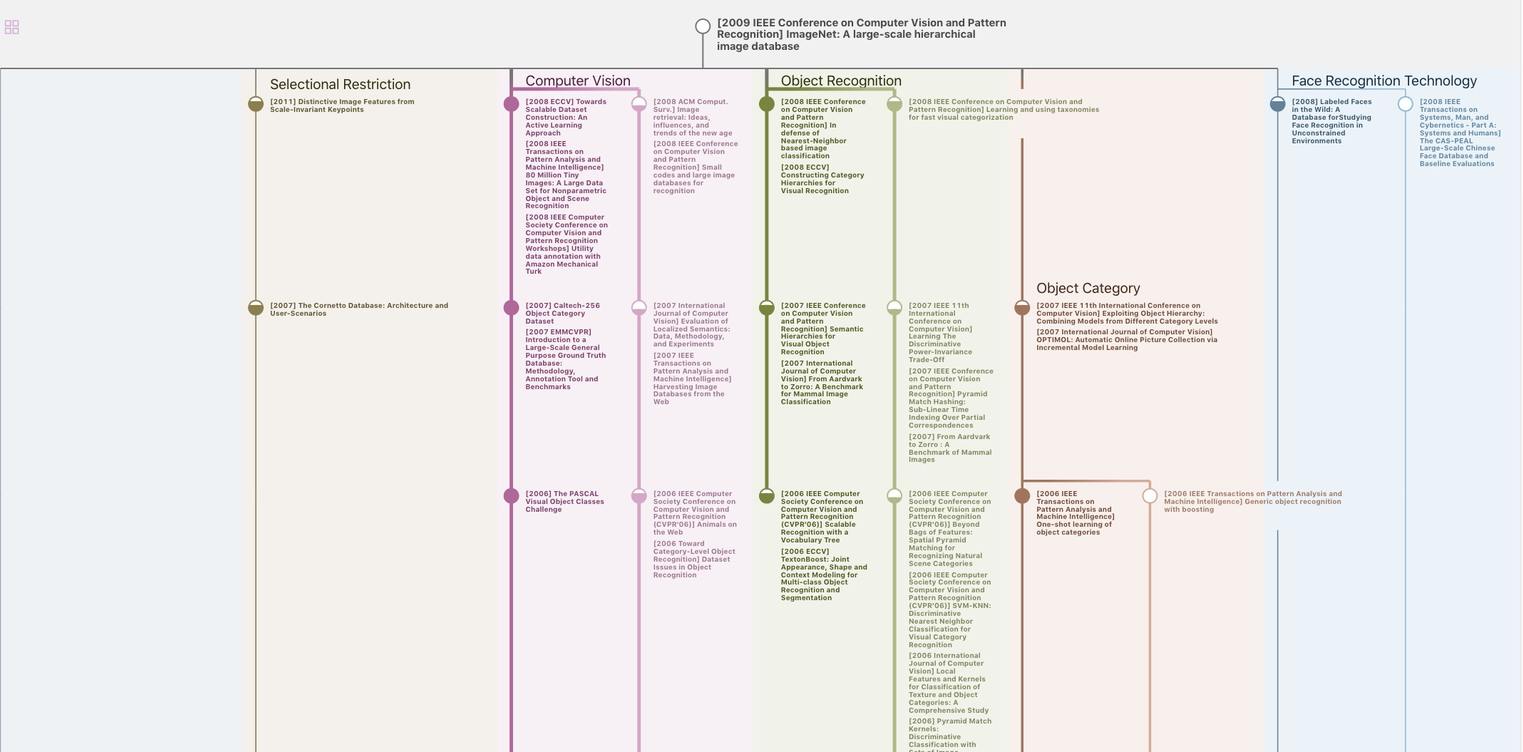
生成溯源树,研究论文发展脉络
Chat Paper
正在生成论文摘要