Modified SkipGram Negative Sampling Model for Faster Convergence of Graph Embedding.
DeLTA (Selected Papers)(2022)
摘要
Graph embedding techniques have been introduced in recent years with the aim of mapping graph data into low-dimensional vector spaces, so that conventional machine learning methods can be exploited. In particular, in the DeepWalk model, truncated random walks are employed in random walk-based approaches to capture structural links-connections between nodes. The SkipGram model is then applied to the truncated random walks to compute the embedded nodes. In this work, the proposed DeepWalk model provides a faster convergence speed than the standard one by introducing a new trainable parameter in the model. Furthermore, experimental results on real-world datasets show that the performance in downstream community detection and link prediction task is improved by using the proposed DeepWalk model.
更多查看译文
关键词
sampling,graph,faster convergence
AI 理解论文
溯源树
样例
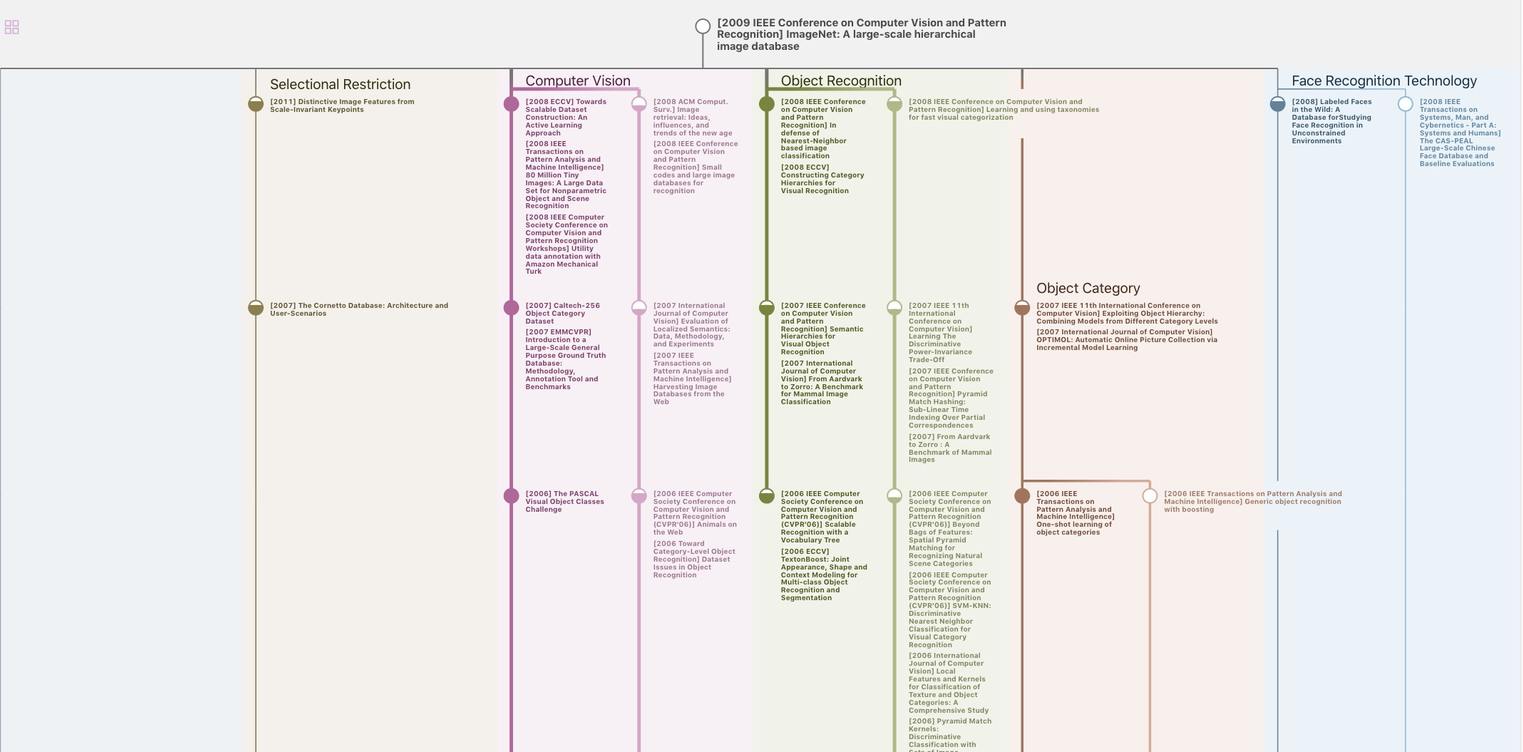
生成溯源树,研究论文发展脉络
Chat Paper
正在生成论文摘要