Suit Up: AI MoCap
SIGGRAPH Real Time Live!(2023)
摘要
We present a novel marker-based motion capture (MoCap) technology. Instead of leveraging initialization and tracking for marker labeling as traditional solutions do, the present system is built upon real-time and low-latency data-driven models and optimization techniques, offering new possibilities and overcoming limitations currently present in the MoCap landscape. Even though we similarly begin with unlabeled markers captured with optical sensing within a capturing area, our approach diverges as we follow a data-driven and optimization pipeline to simultaneously denoise the markers and robustly and accurately solve the skeleton per frame. Similarly to traditional marker-based options, our work demonstrates higher stability and accuracy than inertial and/or markerless optical MoCap. Inertial MoCap lacks absolute positioning and suffers from drifting, therefore, it is almost impossible to achieve comparable positional accuracy. Markerless solutions lack the existence of a strong prior (i.e., markers) to increase the capturing precision, while, due to the heavier workload, the capturing frequency cannot easily scale, resulting in inaccuracies in fast movements. On the other hand, traditional marker-based motion capture heavily relies on high-quality marker data, assuming precise localization, outlier elimination and consistent marker tracking. In contrast, our innovative approach operates without such assumptions, effectively mitigating input noise, including ghost markers, occlusions, marker swaps, misplacement and mispositioning. This noise tolerance enables our system to function seamlessly with cameras with lower cost and specifications. Our method introduces body structure invariance, empowering automatic marker layout configuration by selecting from a diverse pool of models trained with different marker layouts. Our proposed MoCap technology integrates various consumer-grade optical sensors, leverages efficient data acquisition, succeeds in precise marker position estimation and allows for spatio-temporal alignment of multi-view streams. Sequentially, by incorporating data-driven models, our system achieves low latency and real-time rate performances. Finally, efficient body optimization techniques further improve the final MoCap solving, enabling seamless integration into various applications requiring real-time, accurate and robust motion capture. Concluding, real-time communities can be benefited from our MoCap which is a) affordable; with the use of low-cost equipment, b) scalable; with processing on the edge, c) portable; with easy setup and spatial calibration, d) robust; on heavy occlusions, marker removal and camera coverage and e) flexible; no need for super precise marker placement, super precise camera calibration, body calibration per actor or manual marker configuration.
更多查看译文
AI 理解论文
溯源树
样例
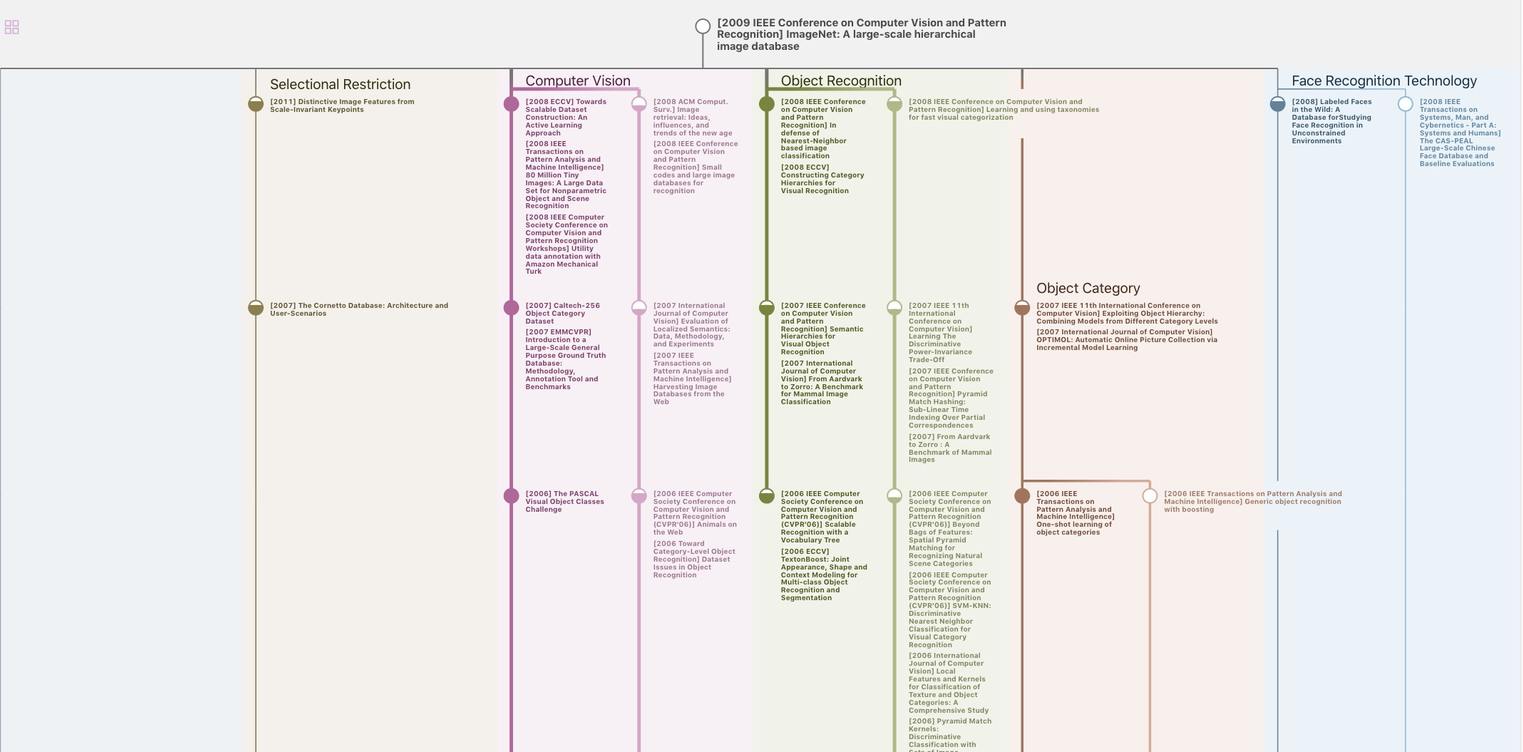
生成溯源树,研究论文发展脉络
Chat Paper
正在生成论文摘要