Cross Attention-based Fusion of Deep and Radiomics Features for Lung Nodule Invasiveness Prediction.
DSP(2023)
摘要
This paper presents an attention-based fusion algorithm that effectively combines two distinct sets of features obtained from chest Computed Tomography (CT) images to predict lung nodule invasiveness. Lung cancer has the highest number of cancer-related deaths worldwide and Lung Adenocarcinoma (LUAC) including three possible invasiveness stages for nodules is discovered as the most prevalent histologic subtype. In this case, developing a proper treatment plan requires accurate information about the invasiveness level of nodules to prevent unwarranted surgeries as pre-invasive/non-invasive nodules can be monitored over time by periodic imaging tests while invasive nodules demand surgical resections. The most reliable method of evaluating nodule invasiveness stage is pathological sampling which is not preferred as the primary diagnostic method due to its invasive nature and potential complications. Currently, chest CT scan is used as an early diagnostic tool by radiologists. However, radiologists’ analysis based on CT images is subjective and highly error-prone. The proposed fusion framework has two parallel feature extraction paths: (i) Deep Learning-based path that extracts deep features via a hierarchical vision transformer using shifted windows known as SWin transformer, and; (ii) Radiomics-based path that consists of extracting quantitative features related to nodule specifications appeared in CT slices. Extracted deep and radiomics features are then fused through a Cross-Attention mechanism acts as the fusion center of our framework to predict nodules invasiveness. Experimental results on our in-house dataset of 114 pathologically proven subsolid nodules present the superiority of the proposed fusion framework among its standalone models of parallel paths and achieved an accuracy of 89.46%, sensitivity of 83.99%, and specificity of 94.99% using 10-fold cross-validation.
更多查看译文
关键词
Lung Adenocarcinoma,Transformer,Subsolid Nodule,Self-Attention,Radiomics
AI 理解论文
溯源树
样例
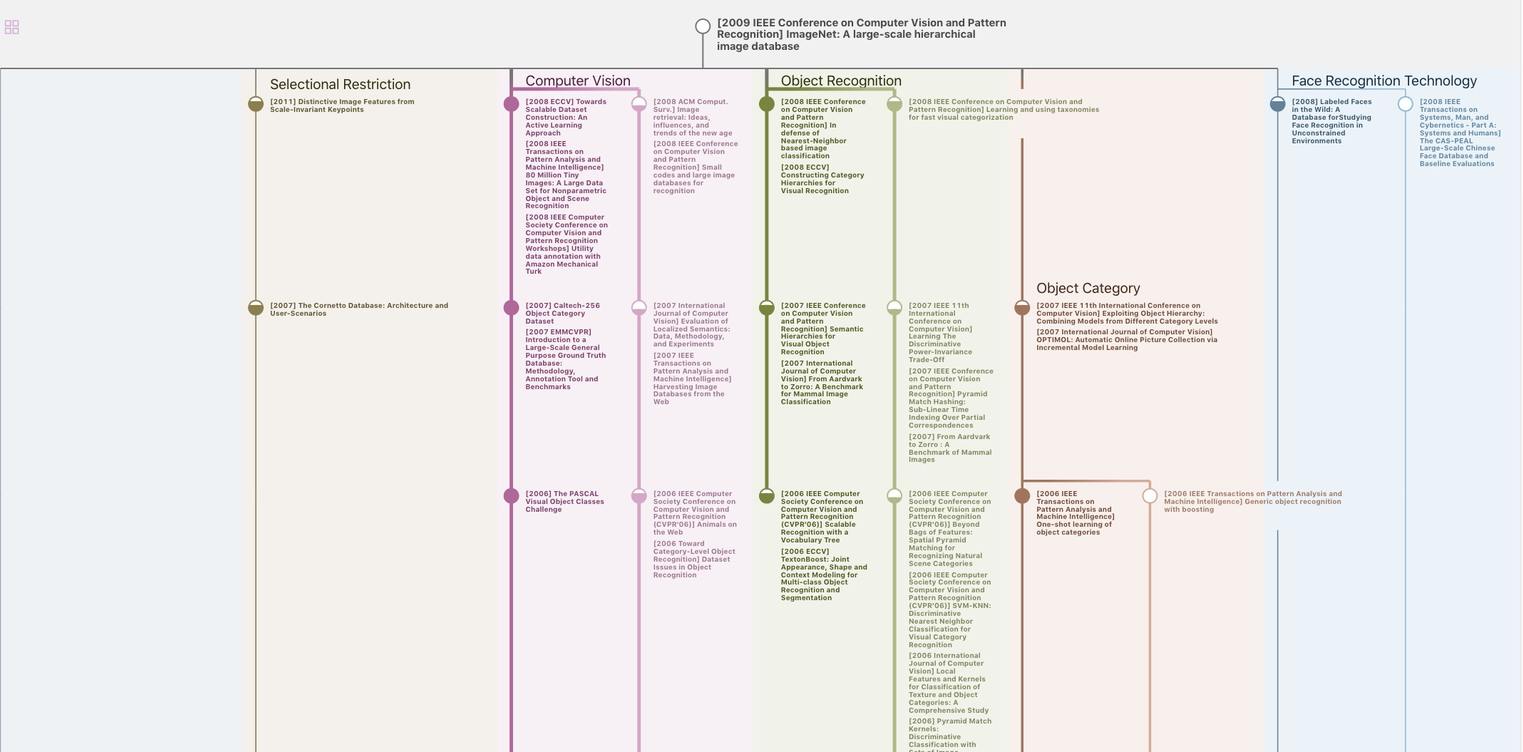
生成溯源树,研究论文发展脉络
Chat Paper
正在生成论文摘要