A comparative study on ML-based approaches for Main Entity Detection in Financial Reports.
DSP(2023)
摘要
Modern AI technologies which exploit the classification and/or prediction capacities of Deep Neural Architectures demonstrate superior performance to traditional approaches in most cases. However, they come with the unavoidable shortcoming of lack of transparency in their outcomes. This attribute renders them unsuitable for big industrial sectors, such as finance, investment management, etc. Specifically, their "black-box" nature makes them unattractive in cases where human understanding in the decision making process is required and may be legally mandatory. In such cases, traditional (i.e., non-deep learning) ML approaches are still preferred, to minimize for example the presence of false positives. In this context, this paper introduces an unsupervised, trustful, bottom-up probabilistic approach for Named Entity Recognition (NER) in financial reports, while in parallel it provides a comparative study on well-known ML-approaches in terms of their performance. The proposed approach builds on the probability of appearance of representative tokens within the given reports and utilizes Kronecker’s Delta and the Total Probability Theorem to construct a probabilistic model that estimates the overall classification probability of a document.
更多查看译文
关键词
big industrial sectors,classification probability,decision making process,Deep Neural Architectures,false positives,finance,financial reports,given reports,human understanding,investment management,main Entity detection,ML-based approaches,modern AI technologies,Named Entity Recognition,nondeep learning,probabilistic approach,transparency,well-known ML-approaches
AI 理解论文
溯源树
样例
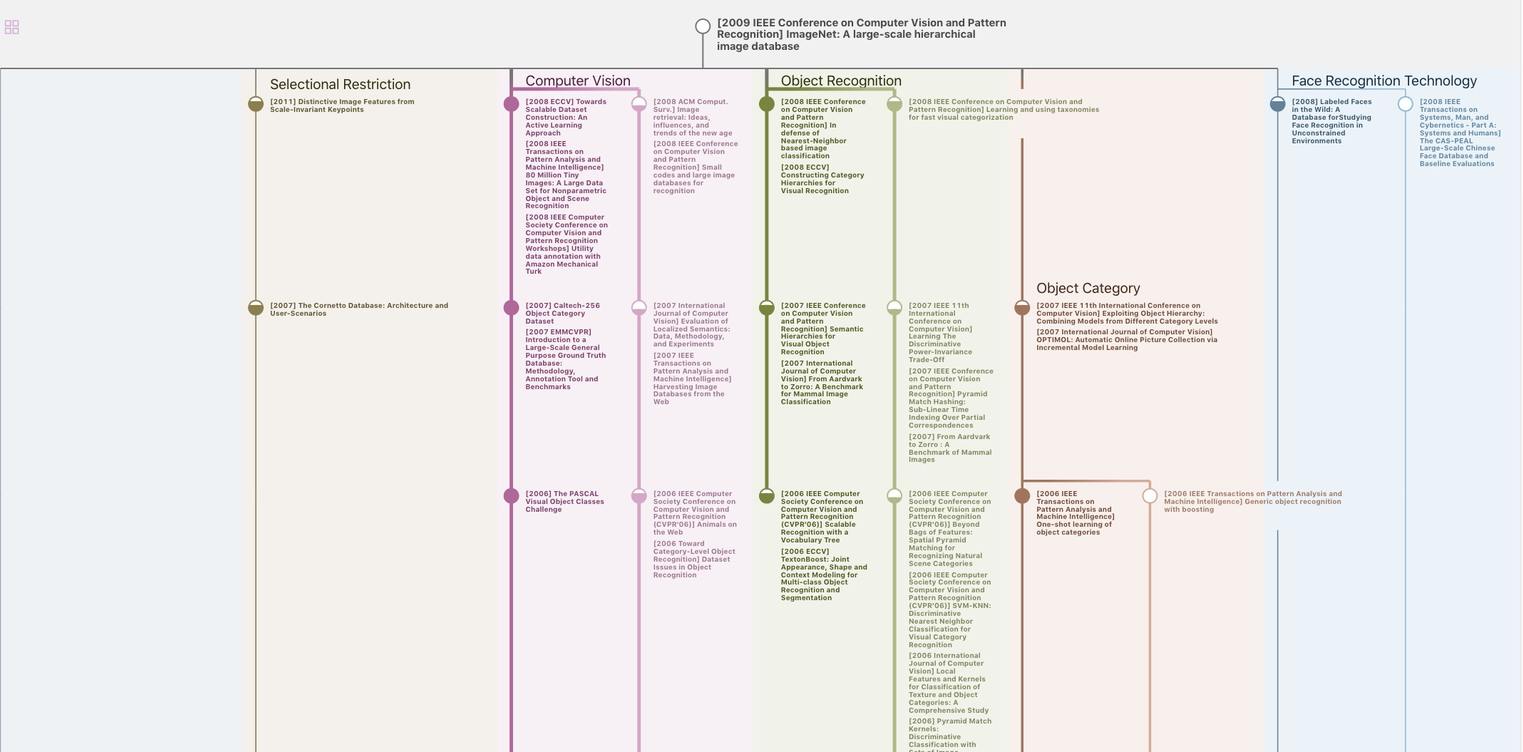
生成溯源树,研究论文发展脉络
Chat Paper
正在生成论文摘要