Distance Correlation GAN: Fair Tabular Data Generation with Generative Adversarial Networks.
HCI (40)(2023)
摘要
With the growing impact of artificial intelligence, the topic of fairness in AI has received increasing attention for valid reasons. In this paper, we propose a generative adversarial network for fair tabular data generation. The model is a WGAN, where the generator is enforcing fairness by penalizing distance correlation between protected attribute and target attribute. We compare our results with another state-of-the-art generative adversarial network for fair tabular data generation and a preprocessing repairment method on four datasets, and show that our model is able to produce synthetic data, such that training a classifier on it results in a fair classifier, beating the other two methods. This makes the model suitable for applications that concern with fairness and preserving privacy.
更多查看译文
关键词
fair tabular data generation,generative adversarial networks,gan
AI 理解论文
溯源树
样例
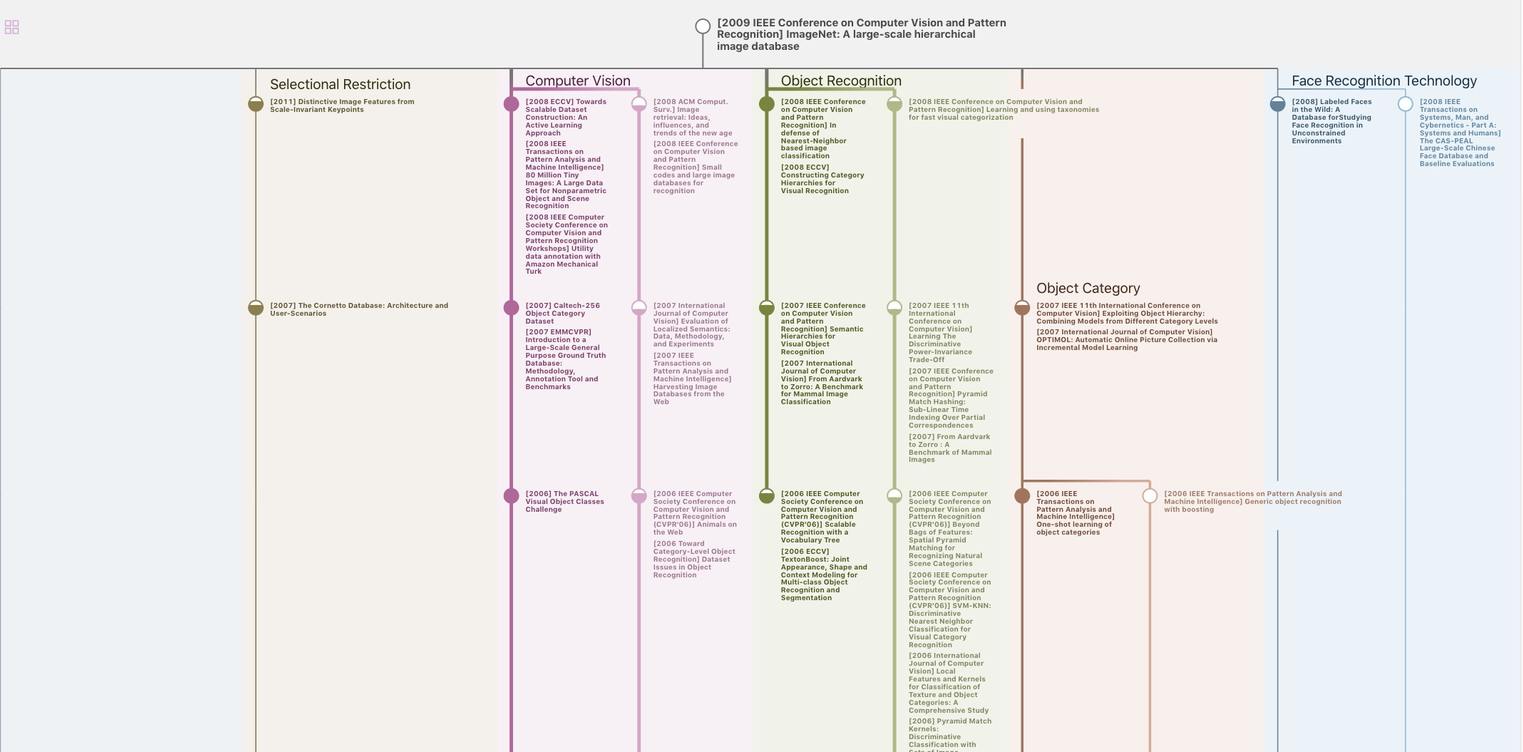
生成溯源树,研究论文发展脉络
Chat Paper
正在生成论文摘要