Quantifying and Visualizing the Sharpness of Attraction Basins in Neural Network Error Landscapes
PROCEEDINGS OF THE 2023 GENETIC AND EVOLUTIONARY COMPUTATION CONFERENCE COMPANION, GECCO 2023 COMPANION(2023)
摘要
Algorithms used to train neural networks (NNs) converge in valley-like parts of the loss landscape. The point of convergence is considered a flat minimum when the valley is wide, and a sharp minimum when the valley is narrow. It has been hypothesized that flat minima in wide valleys generalize better than sharp minima in narrow valleys, but this has not been shown to hold in general. Theoretical studies propose conflicting ideas about the relevance, shape, and definition of sharp and flat minima. This study proposes a method for sampling the neighborhood around local minima of NN error landscapes. Using this method, we define two metrics to quantify the sharpness of minima as well as a 2-dimensional visualization for approximating the structure of the basins of attraction. We then conduct experiments to verify or refute the correlation between the sharpness of a valley around a local minimum and the ability of the NN to generalize well at that minimum. Results provide no evidence to support this correlation.
更多查看译文
关键词
neural networks,error landscape analysis,error landscape visualization,sharp and flat minima,basins of attraction,generalization
AI 理解论文
溯源树
样例
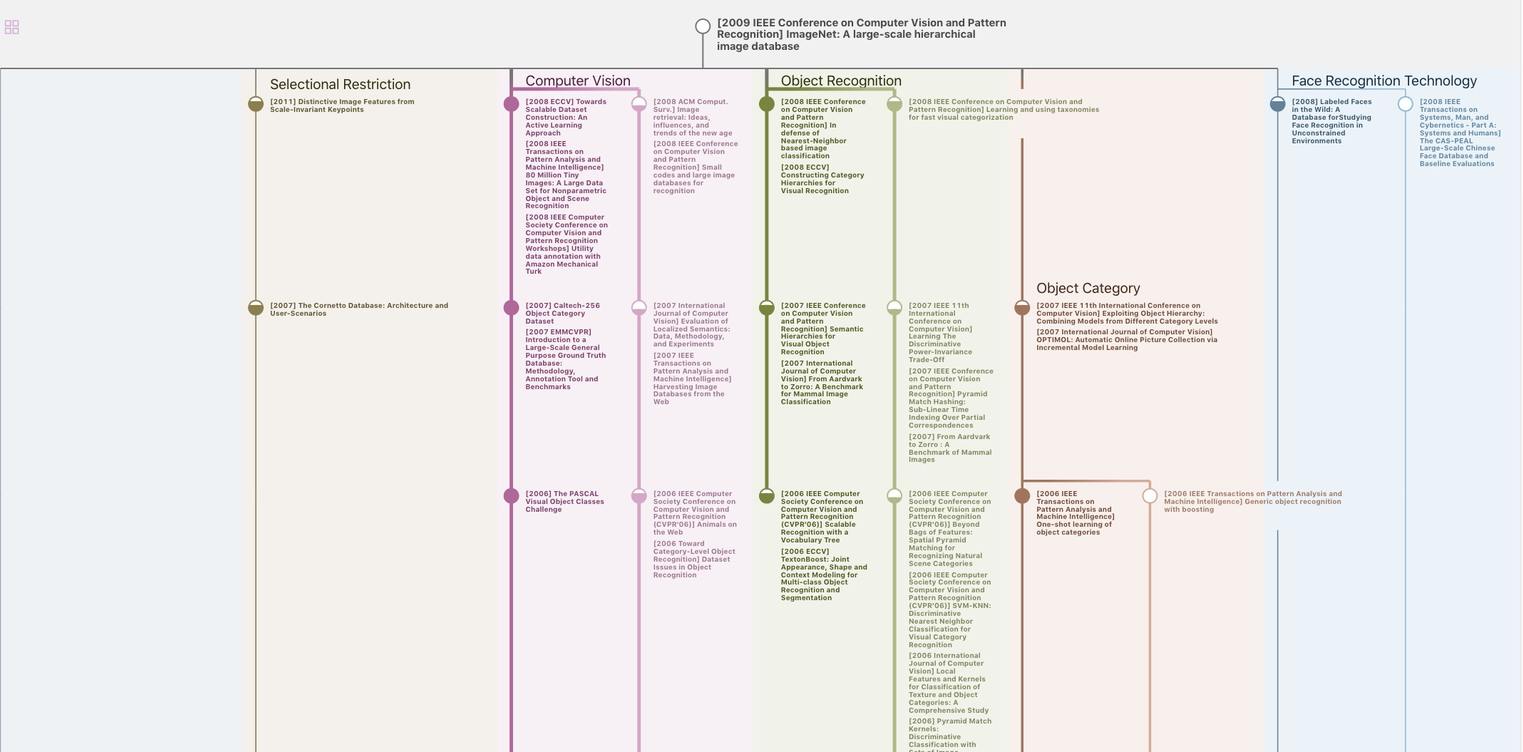
生成溯源树,研究论文发展脉络
Chat Paper
正在生成论文摘要