Style-Content Metric Learning for Multidomain Remote Sensing Object Recognition.
AAAI(2023)
摘要
Previous remote sensing recognition approaches predominantly perform well on the training-testing dataset. However, due to large style discrepancies not only among multidomain datasets but also within a single domain, they suffer from obvious performance degradation when applied to unseen domains. In this paper, we propose a style-content metric learning framework to address the generalizable remote sensing object recognition issue. Specifically, we firstly design an inter-class dispersion metric to encourage the model to make decision based on content rather than the style, which is achieved by dispersing predictions generated from the contents of both positive sample and negative sample and the style of input image. Secondly, we propose an intra-class compactness metric to force the model to be less style-biased by compacting classifier's predictions from the content of input image and the styles of positive sample and negative sample. Lastly, we design an intra-class interaction metric to improve model's recognition accuracy by pulling in classifier's predictions obtained from the input image and positive sample. Extensive experiments on four datasets show that our style-content metric learning achieves superior generalization performance against the state-of-the-art competitors.
更多查看译文
关键词
remote sensing,recognition,learning,style-content
AI 理解论文
溯源树
样例
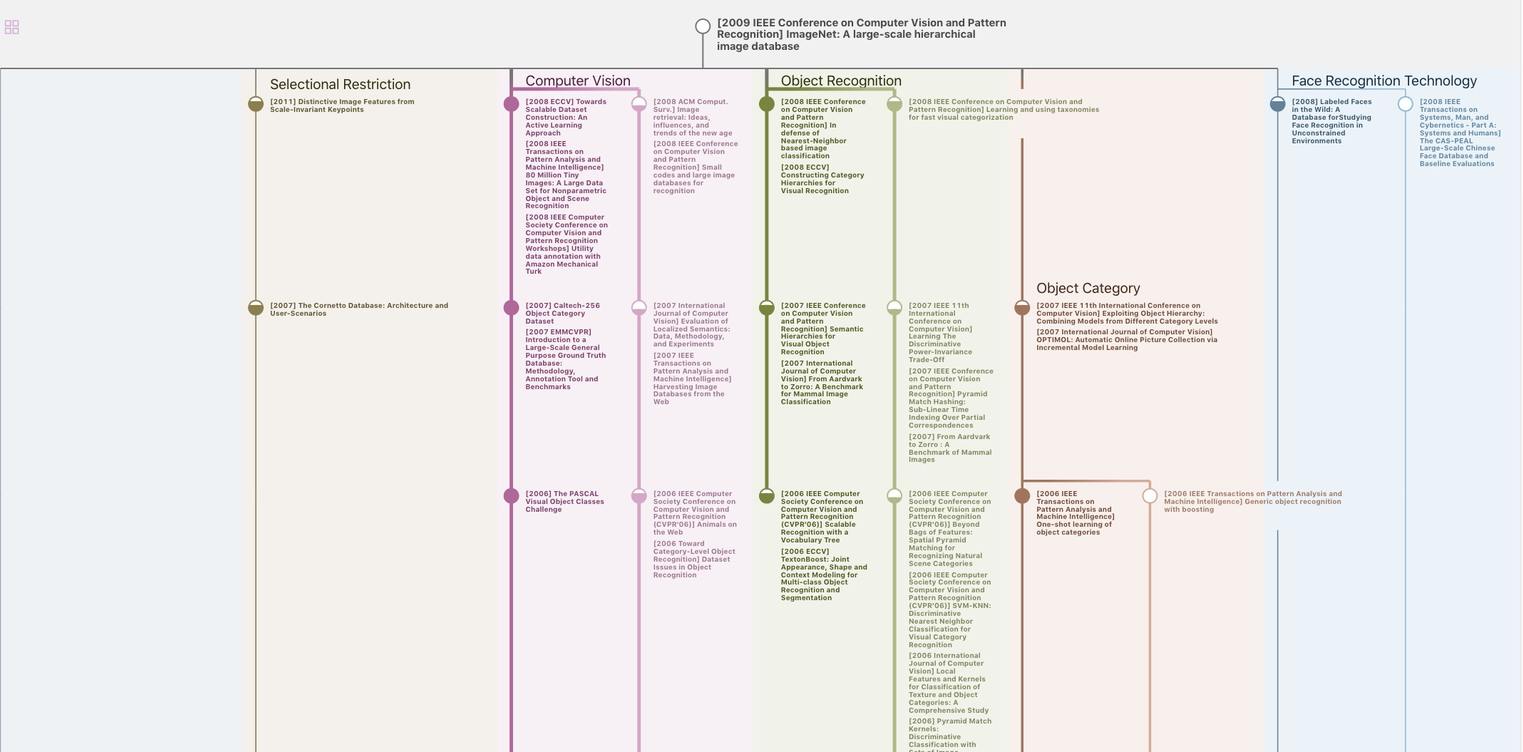
生成溯源树,研究论文发展脉络
Chat Paper
正在生成论文摘要