Popularizing Fairness: Group Fairness and Individual Welfare.
AAAI(2023)
摘要
Group-fair learning methods typically seek to ensure that some measure of prediction efficacy for (often historically) disadvantaged minority groups is comparable to that for the majority of the population. When a principal seeks to adopt a group-fair approach to replace another, more conventional approach, the principal may face opposition from those who feel that they have been disadvantaged as a result of the switch, and this, in turn, may deter adoption. We propose to mitigate this concern by ensuring that a group-fair model is also popular , in the sense that it yields a preferred distribution over outcomes compared with the conventional model for a majority of the target population. First, we show that state of the art fair learning approaches are often unpopular in this sense. We then present several efficient algorithms for postprocessing an existing group-fair learning scheme to improve its popularity while retaining fairness. Through extensive experiments, we demonstrate that the proposed postprocessing approaches are highly effective.
更多查看译文
关键词
group fairness,individual welfare
AI 理解论文
溯源树
样例
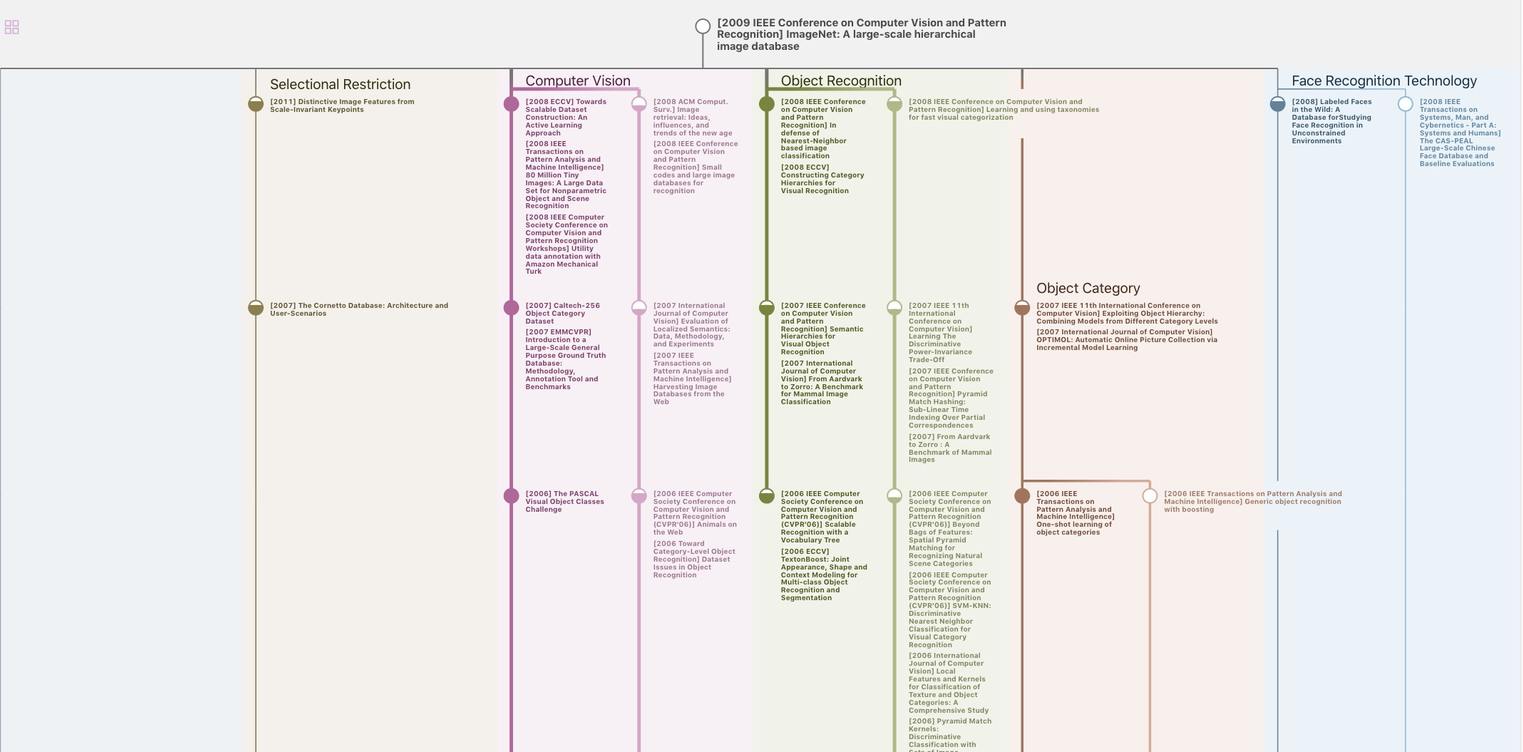
生成溯源树,研究论文发展脉络
Chat Paper
正在生成论文摘要