KerPrint: Local-Global Knowledge Graph Enhanced Diagnosis Prediction for Retrospective and Prospective Interpretations.
AAAI(2023)
摘要
While recent developments of deep learning models have led to record-breaking achievements in many areas, the lack of sufficient interpretation remains a problem for many specific applications, such as the diagnosis prediction task in healthcare. The previous knowledge graph(KG) enhanced approaches mainly focus on learning clinically meaningful representations, the importance of medical concepts, and even the knowledge paths from inputs to labels. However, it is infeasible to interpret the diagnosis prediction, which needs to consider different medical concepts, various medical relationships, and the time-effectiveness of knowledge triples in different patient contexts. More importantly, the retrospective and prospective interpretations of disease processes are valuable to clinicians for the patients' confounding diseases. We propose KerPrint, a novel KG enhanced approach for retrospective and prospective interpretations to tackle these problems. Specifically, we propose a time-aware KG attention method to solve the problem of knowledge decay over time for trustworthy retrospective interpretation. We also propose a novel element-wise attention method to select candidate global knowledge using comprehensive representations from the local KG for prospective interpretation. We validate the effectiveness of our KerPrint through an extensive experimental study on a real-world dataset and a public dataset. The results show that our proposed approach not only achieves significant improvement over knowledge-enhanced methods but also gives the interpretability of diagnosis prediction in both retrospective and prospective views.
更多查看译文
关键词
diagnosis,prediction,knowledge,local-global
AI 理解论文
溯源树
样例
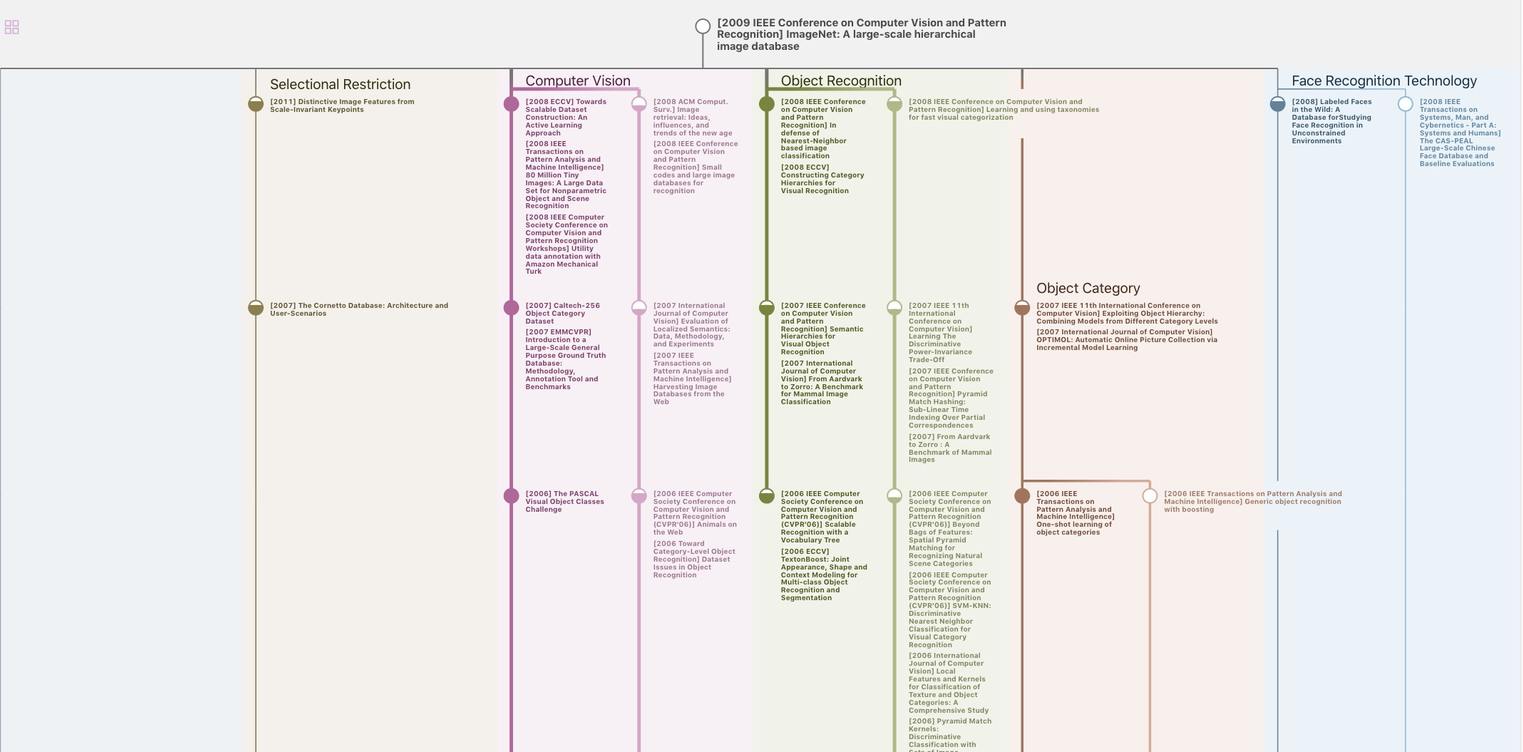
生成溯源树,研究论文发展脉络
Chat Paper
正在生成论文摘要