Use of Decentralized-Learning Methods Applied to Healthcare: A Bibliometric Analysis.
ICCS (3)(2023)
Abstract
The use of health data in research is fundamental to improve health care, health systems and public health policies. However, due to its intrinsic data sensitivity, there are privacy and security concerns that must be addressed to comply with best practices recommendations and the legal framework. Decentralized-learning methods allow the training of algorithms across multiple locations without data sharing. The application of those methods to the medical field holds great potential due to the guarantee of data privacy compliance. In this study, we performed a bibliometric analysis to explore the intellectual structure of this new research field and to assess its publication and collaboration patterns. A total of 3023 unique documents published between 2013 and 2023 were retrieved from Scopus, from which 488 were included in this review. The most frequent publication source was the IEEE Journal of Biomedical and Health Informatics (n = 27). China was the country with the highest number of publications, followed by the USA. The top three authors were Dekker A (n = 14), Wang X (n = 13), Li X (n = 12). The most frequent keywords were “Federated learning” (n = 218), “Deep learning” (n = 62) and “Machine learning” (n = 52). This study provides an overall picture of the research literature regarding the application of decentralized-learning in healthcare, possibly setting ground for future collaborations.
MoreTranslated text
Key words
healthcare,decentralized-learning
AI Read Science
Must-Reading Tree
Example
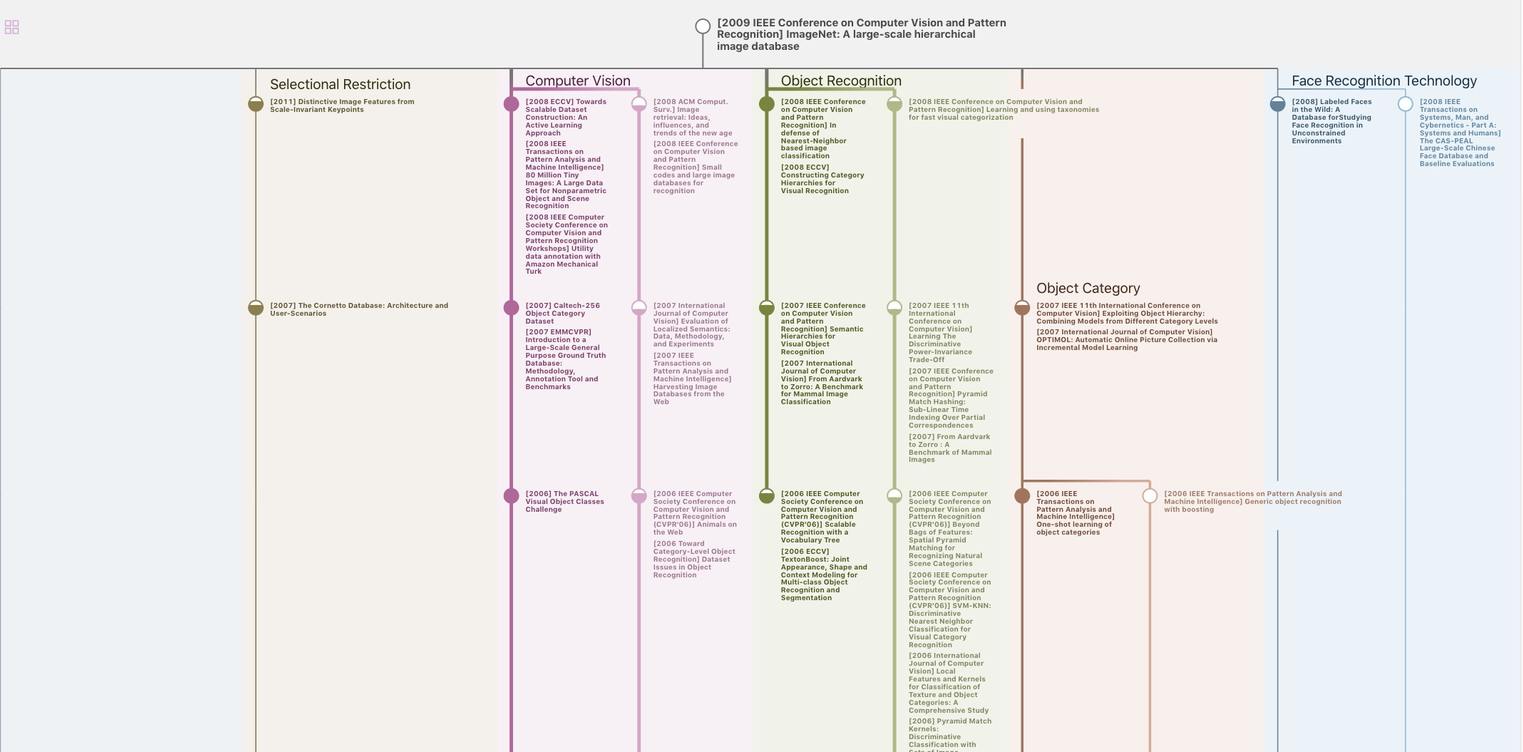
Generate MRT to find the research sequence of this paper
Chat Paper
Summary is being generated by the instructions you defined