Diffusion Maps for Textual Network Embedding
ADVANCES IN NEURAL INFORMATION PROCESSING SYSTEMS 31 (NIPS 2018)(2019)
摘要
Textual network embedding leverages rich text information associated with the network to learn low-dimensional vectorial representations of vertices. Rather than using typical natural language processing (NLP) approaches, recent research exploits the relationship of texts on the same edge to graphically embed text. However, these models neglect to measure the complete level of connectivity between any two texts in the graph. We present diffusion maps for textual network embedding (DMTE), integrating global structural information of the graph to capture the semantic relatedness between texts, with a diffusion-convolution operation applied on the text inputs. In addition, a new objective function is designed to efficiently preserve the high-order proximity using the graph diffusion. Experimental results show that the proposed approach outperforms state-of-the-art methods on the vertex-classification and link-prediction tasks.
更多查看译文
关键词
textual network,diffusion,maps
AI 理解论文
溯源树
样例
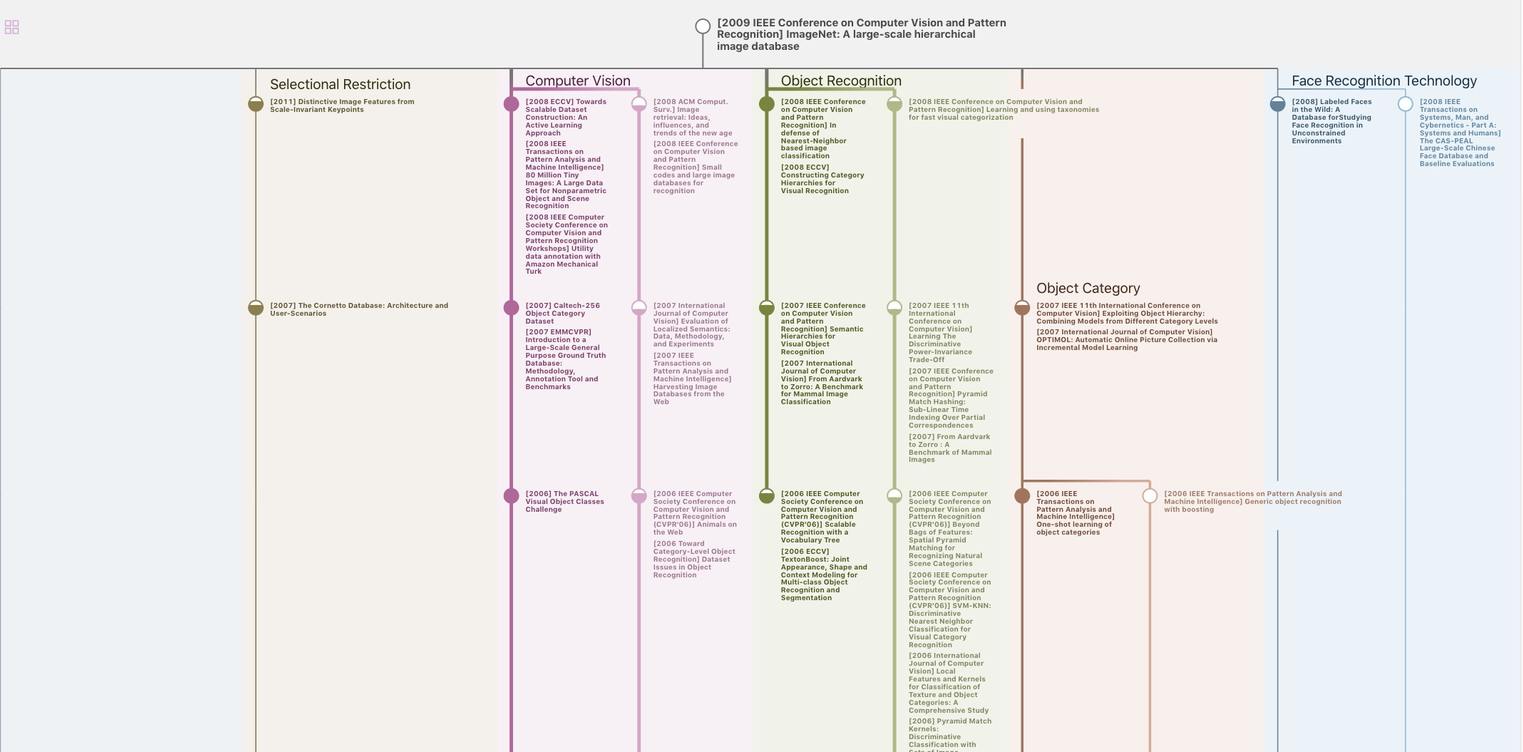
生成溯源树,研究论文发展脉络
Chat Paper
正在生成论文摘要