Multi-Robot Expansive Planning and Trajectory Evaluation for Tracking and Localization of Marine Life
38TH ANNUAL ACM SYMPOSIUM ON APPLIED COMPUTING, SAC 2023(2023)
摘要
Traditional techniques for marine life tracking use stationary receivers that detect and obtain measurements from tagged animals. Recently, such static systems have been replaced by multiple mobile robots, e.g., autonomous underwater vehicles (AUVs), equipped with omni-directional hydrophones that can accurately localize marine life. In this paper, the application of homogeneous multi-AUV systems to track and localize marine life is used as a motivating example to develop new MRMP (Multi-Robot Motion Planning) algorithms. These algorithms generate trajectories that maximize a new fitness function that incorporates 1) probabilistic motion models generated from historical data of live sharks, and 2) ideal AUV formations for observing a shark from multiple sensor vantage points. The two expansive RRT variants, named Independent State Expansion (ISE) planning and Joint State Expansion (JSE) planning, differ in how new samples are randomly generated during the algorithm's random search. The fitness function was developed to quantify how accurately the positioning of AUVs would trilaterate the target animal. Through simulation, it was found that the Joint planner was 70% faster with respect to run time than Independent planner, while both could produce similar mean fitness function values. The fitness for these variants was also measured for simulations where different target motion models were used when calculating the fitness function, highlighting the improved performance when using actual target motion motion models.
更多查看译文
关键词
Multi-robot Systems,Autonomous Robots,Cooperative Robots,Decentralized Algorithms
AI 理解论文
溯源树
样例
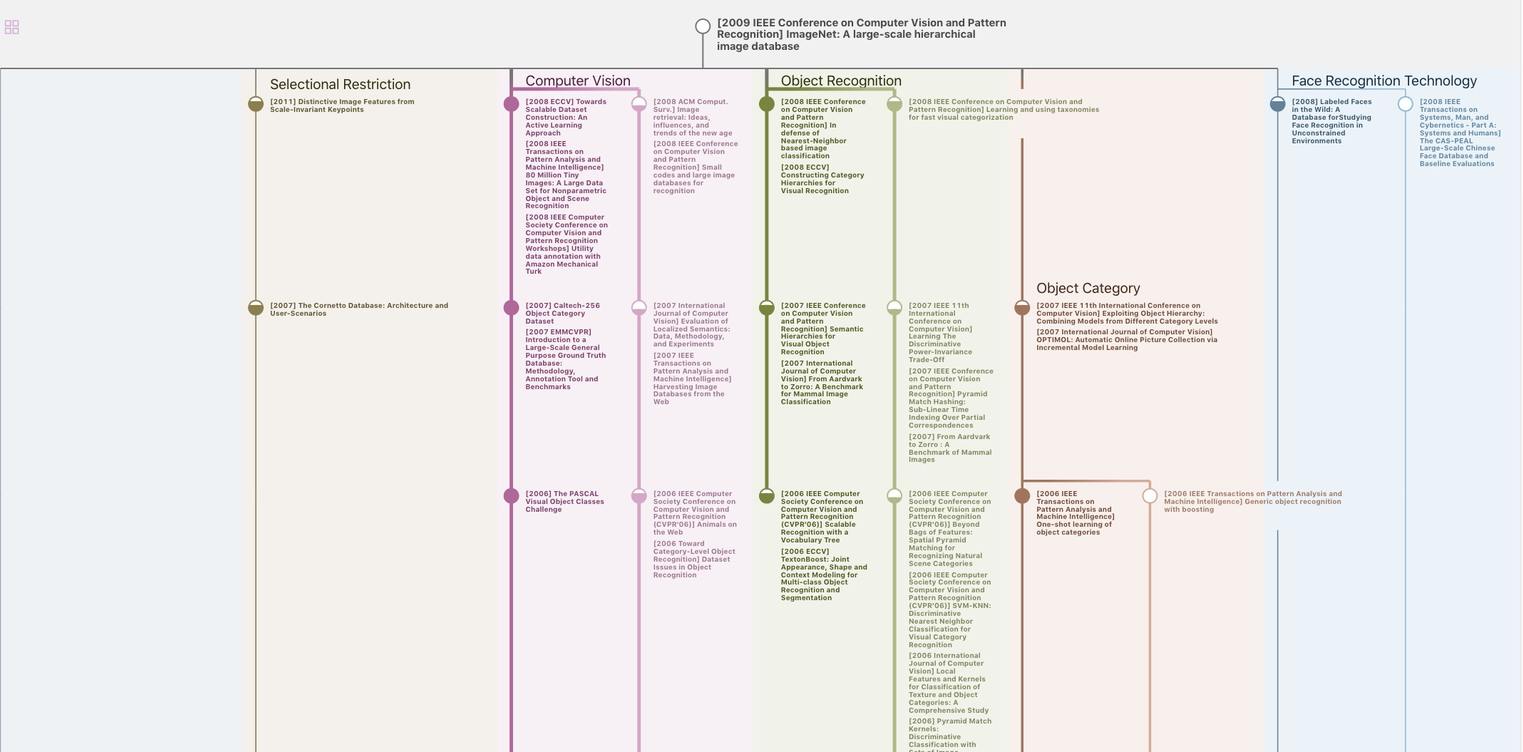
生成溯源树,研究论文发展脉络
Chat Paper
正在生成论文摘要