Evolving Better Initializations For Neural Networks With Pruning
PROCEEDINGS OF THE 2023 GENETIC AND EVOLUTIONARY COMPUTATION CONFERENCE COMPANION, GECCO 2023 COMPANION(2023)
摘要
Recent work in deep learning has shown that neural networks can be pruned before training to achieve similar or even better results than training the full network. However, existing pruning methods are limited and do not necessarily yield optimal solutions. In this work, we show that perturbing the network by re-initializing the pruned weights and re-pruning can improve performance. We propose to iteratively re-initialize and re-prune using a hill climbing (1 + 1) evolution strategy. We examine the cause of these improvements and show that this method can consistently improve the subnetwork without increasing its size, pointing to a potential new application of evolutionary computing in deep learning.
更多查看译文
关键词
neuroevolution,neural network pruning,neural network initialization,lottery ticket hypothesis,evolution strategies
AI 理解论文
溯源树
样例
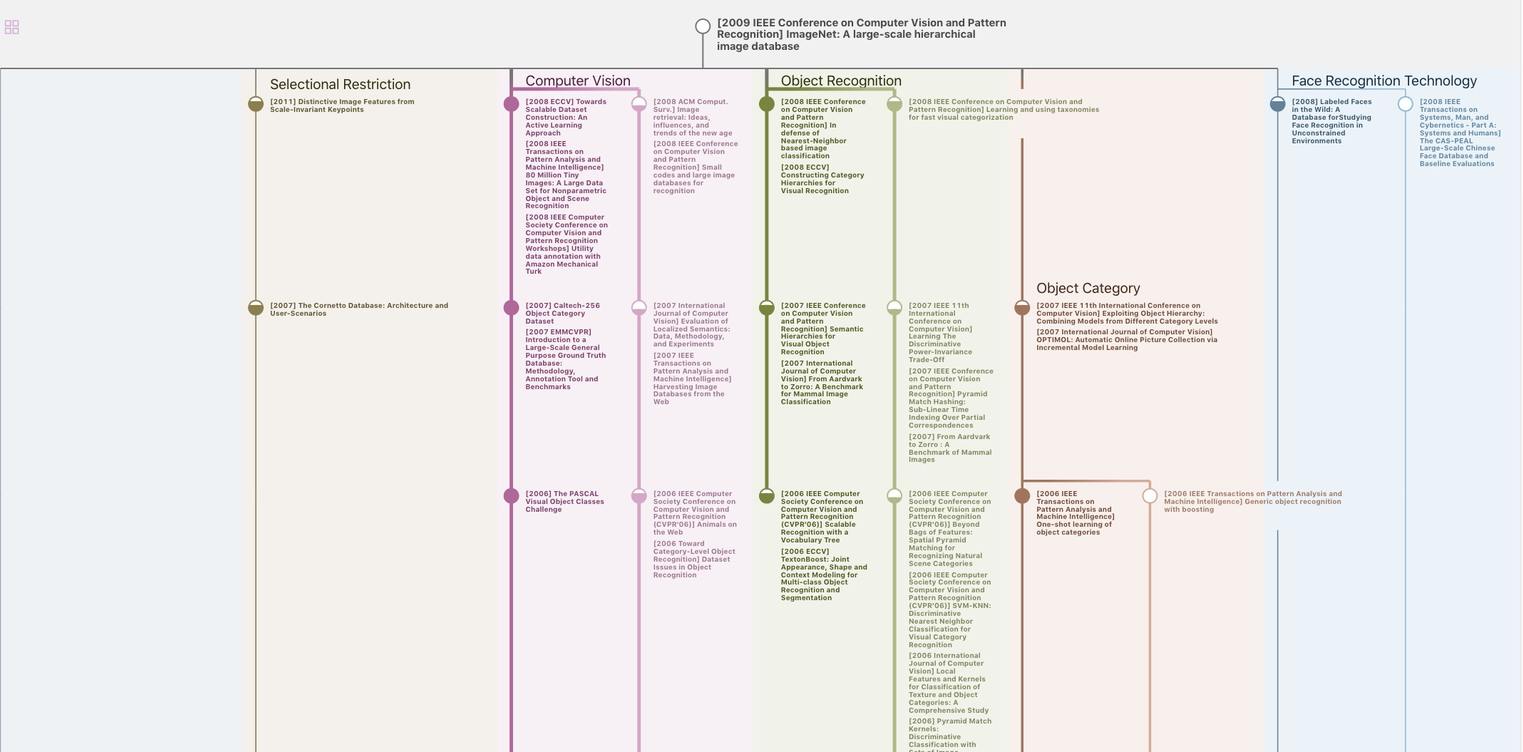
生成溯源树,研究论文发展脉络
Chat Paper
正在生成论文摘要