Multivariate Time Series Evapotranspiration Forecasting using Machine Learning Techniques
38TH ANNUAL ACM SYMPOSIUM ON APPLIED COMPUTING, SAC 2023(2023)
Abstract
The actual evapotranspiration (AET) could be forecasted using meteorological variables to manage and plan water resources even though it is challenging to choose the relevant variables for prediction. The Pearson correlation method was applied to select candidate variables and further, tolerance and VIF scores are implemented to avoid multicollinearity problems among variables. As a result, five relevant variables are selected for training the AET prediction models. In this paper, we proposed three methods for forecasting AET: (i) deep learning-based (LSTM, GRU, and CNN), (ii) classical machine learning (SVR and RF), and (iii) a statistical technique (SARIMAX). The performance of each model is measured with statistical indicators (RMSE, MSE, MAE, and R-2). The results showed that relatively high performance is measured in the LSTM model.
MoreTranslated text
Key words
evapotranspiration,deep learning,machine learning,multivariate time series analysis
AI Read Science
Must-Reading Tree
Example
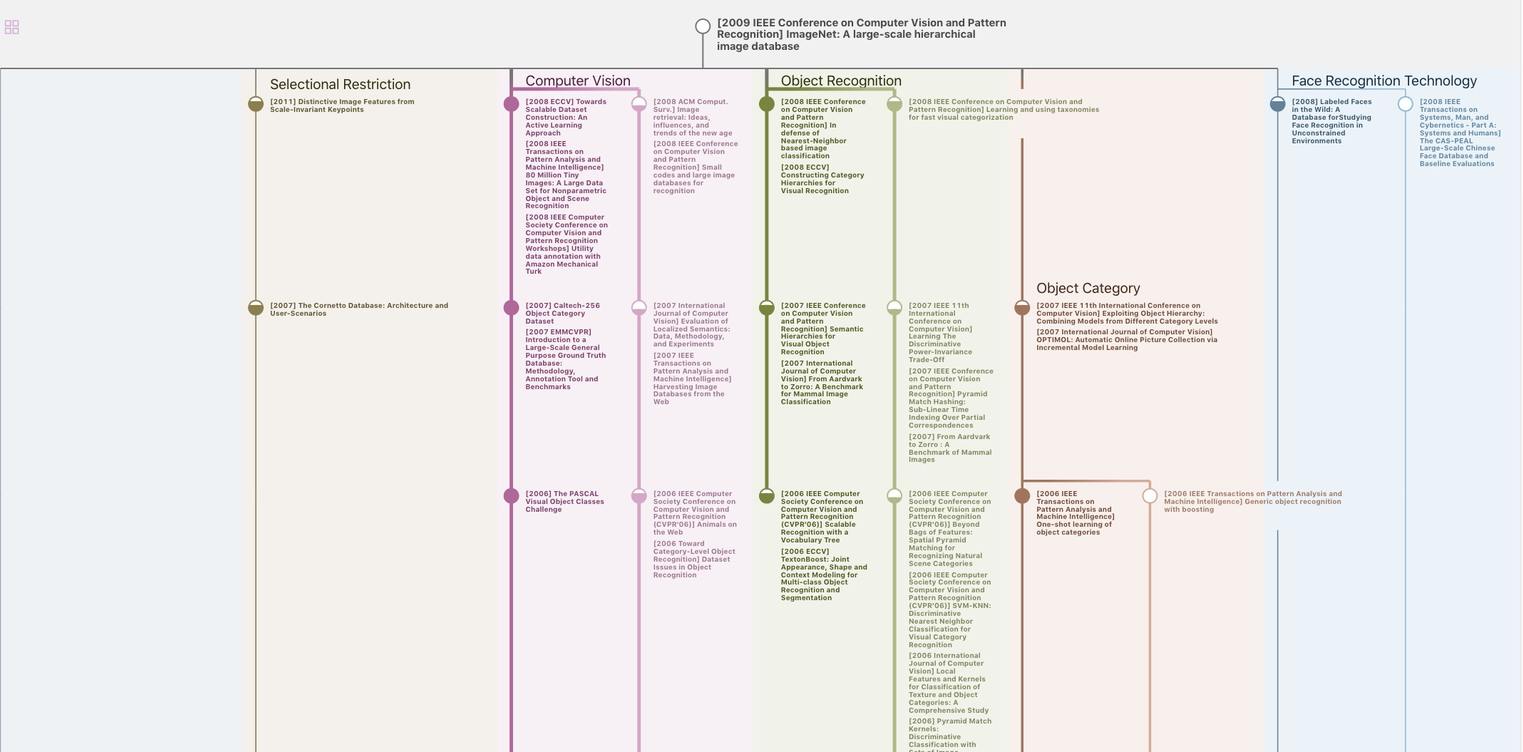
Generate MRT to find the research sequence of this paper
Chat Paper
Summary is being generated by the instructions you defined