One-for-All: Proposal Masked Cross-Class Anomaly Detection.
AAAI(2023)
摘要
One of the most challenges for anomaly detection (AD) is how to learn one unified and generalizable model to adapt to multi-class especially cross-class settings: the model is trained with normal samples from seen classes with the objective to detect anomalies from both seen and unseen classes. In this work, we propose a novel Proposal Masked Anomaly Detection (PMAD) approach for such challenging multi-and cross-class anomaly detection. The proposed PMAD can be adapted to seen and unseen classes by two key designs: MAE-based patch-level reconstruction and prototype-guided proposal masking. First, motivated by MAE (Masked AutoEncoder), we develop a patch-level reconstruction model rather than the image-level reconstruction adopted in most AD methods for this reason: the masked patches in unseen classes can be reconstructed well by using the visible patches and the adaptive reconstruction capability of MAE. Moreover, we improve MAE by ViT encoder-decoder architecture, combinational masking, and visual tokens as reconstruction objectives to make it more suitable for anomaly detection. Second, we develop a two-stage anomaly detection manner during inference. In the proposal masking stage, the prototype-guided proposal masking module is utilized to generate proposals for suspicious anomalies as much as possible, then masked patches can be generated from the proposal regions. By masking most likely anomalous patches, the "shortcut reconstruction" issue ( i.e. , anomalous regions can be well reconstructed) can be mostly avoided. In the reconstruction stage, these masked patches are then reconstructed by the trained patchlevel reconstruction model to determine if they are anomalies. Extensive experiments show that the proposed PMAD can outperform current state-of-the-art models significantly under the multi-and especially cross-class settings.
更多查看译文
关键词
detection,anomaly,one-for-all,cross-class
AI 理解论文
溯源树
样例
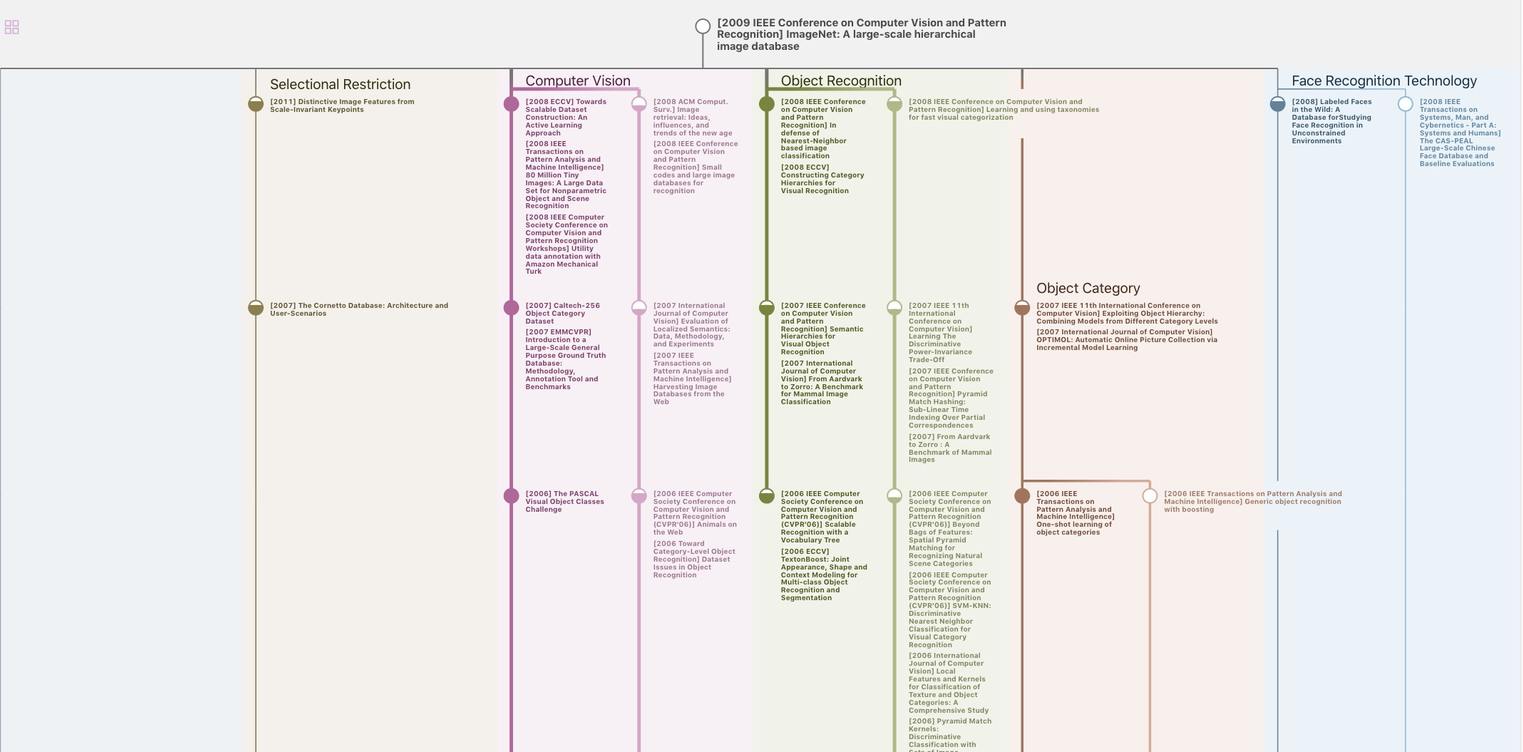
生成溯源树,研究论文发展脉络
Chat Paper
正在生成论文摘要