Incremental Reinforcement Learning with Dual-Adaptive ε-Greedy Exploration.
AAAI(2023)
摘要
Reinforcement learning (RL) has achieved impressive performance in various domains. However, most RL frameworks oversimplify the problem by assuming a fixed-yet-known environment and often have difficulty being generalized to real-world scenarios. In this paper, we address a new challenge with a more realistic setting, Incremental Reinforcement Learning , where the search space of the Markov Decision Process continually expands. While previous methods usually suffer from the lack of efficiency in exploring the unseen transitions, especially with increasing search space, we present a new exploration framework named Dual-Adaptive e-greedy Exploration (DAE) to address the challenge of Incremental RL. Specifically, DAE employs a Meta Policy and an Explorer to avoid redundant computation on those sufficiently learned samples. Furthermore, we release a testbed based on a synthetic environment and the Atari benchmark to validate the effectiveness of any exploration algorithms under Incremental RL. Experimental results demonstrate that the proposed framework can efficiently learn the unseen transitions in new environments, leading to notable performance improvement, i.e., an average of more than 80%, over eight baselines examined.
更多查看译文
关键词
exploration,learning,dual-adaptive
AI 理解论文
溯源树
样例
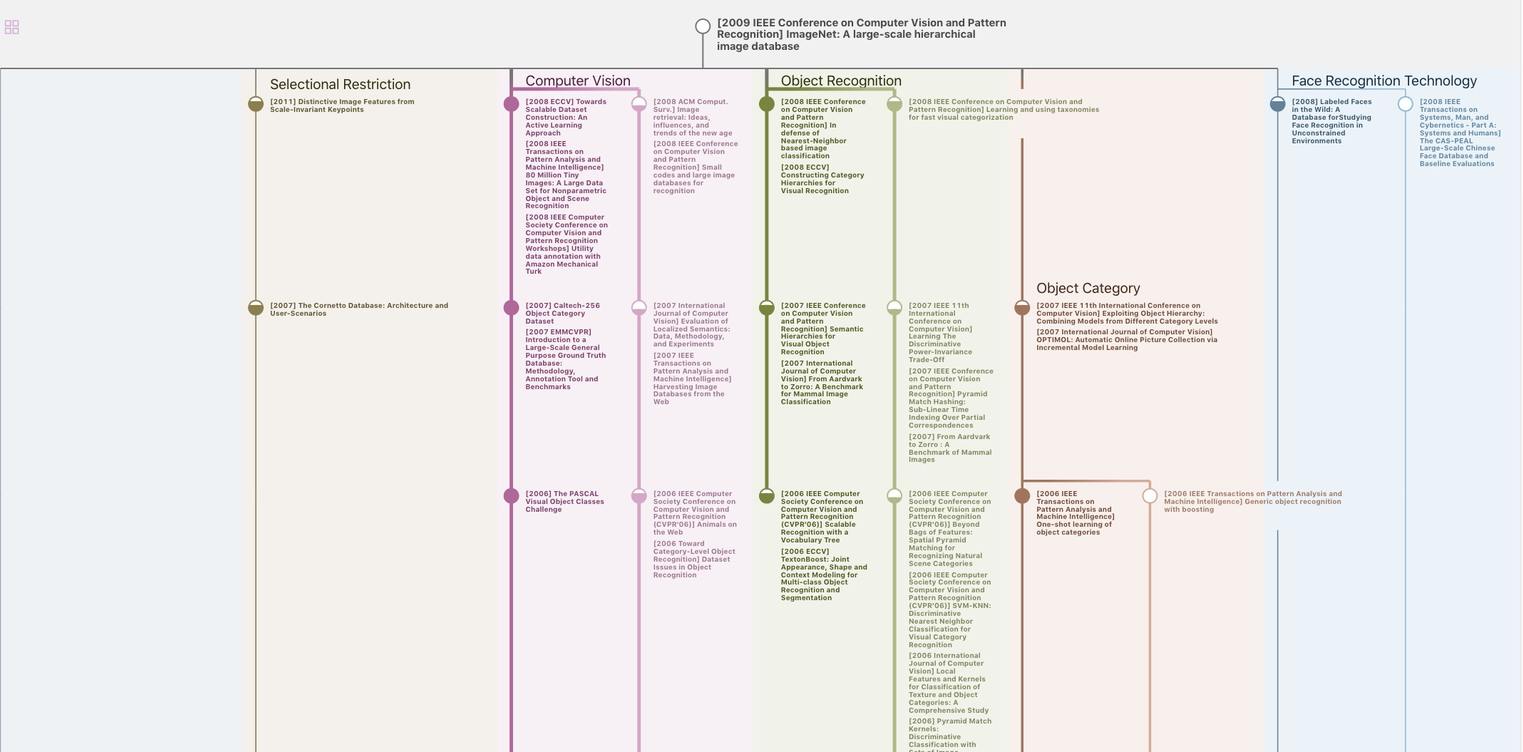
生成溯源树,研究论文发展脉络
Chat Paper
正在生成论文摘要