See Your Emotion from Gait Using Unlabeled Skeleton Data.
AAAI(2023)
摘要
This paper focuses on contrastive learning for gait-based emotion recognition. The existing contrastive learning approaches are rarely suitable for learning skeleton-based gait representations, which suffer from limited gait diversity and inconsistent semantics. In this paper, we propose a Cross-coordinate contrastive learning framework utilizing Ambiguity samples for self-supervised Gait-based Emotion representation (CAGE). First, we propose ambiguity transform to push positive samples into ambiguous semantic space. By learning similarities between ambiguity samples and positive samples, our model can learn higher-level semantics of the gait sequences and maintain semantic diversity. Second, to encourage learning the semantic invariance, we uniquely propose cross-coordinate contrastive learning between the Cartesian coordinate and the Spherical coordinate, which brings rich supervisory signals to learn the intrinsic semantic consistency information. Exhaustive experiments show that CAGE improves existing self-supervised methods by 5%-10% accuracy, and it achieves comparable or even superior performance to supervised methods.
更多查看译文
关键词
unlabeled skeleton data,emotion,gait
AI 理解论文
溯源树
样例
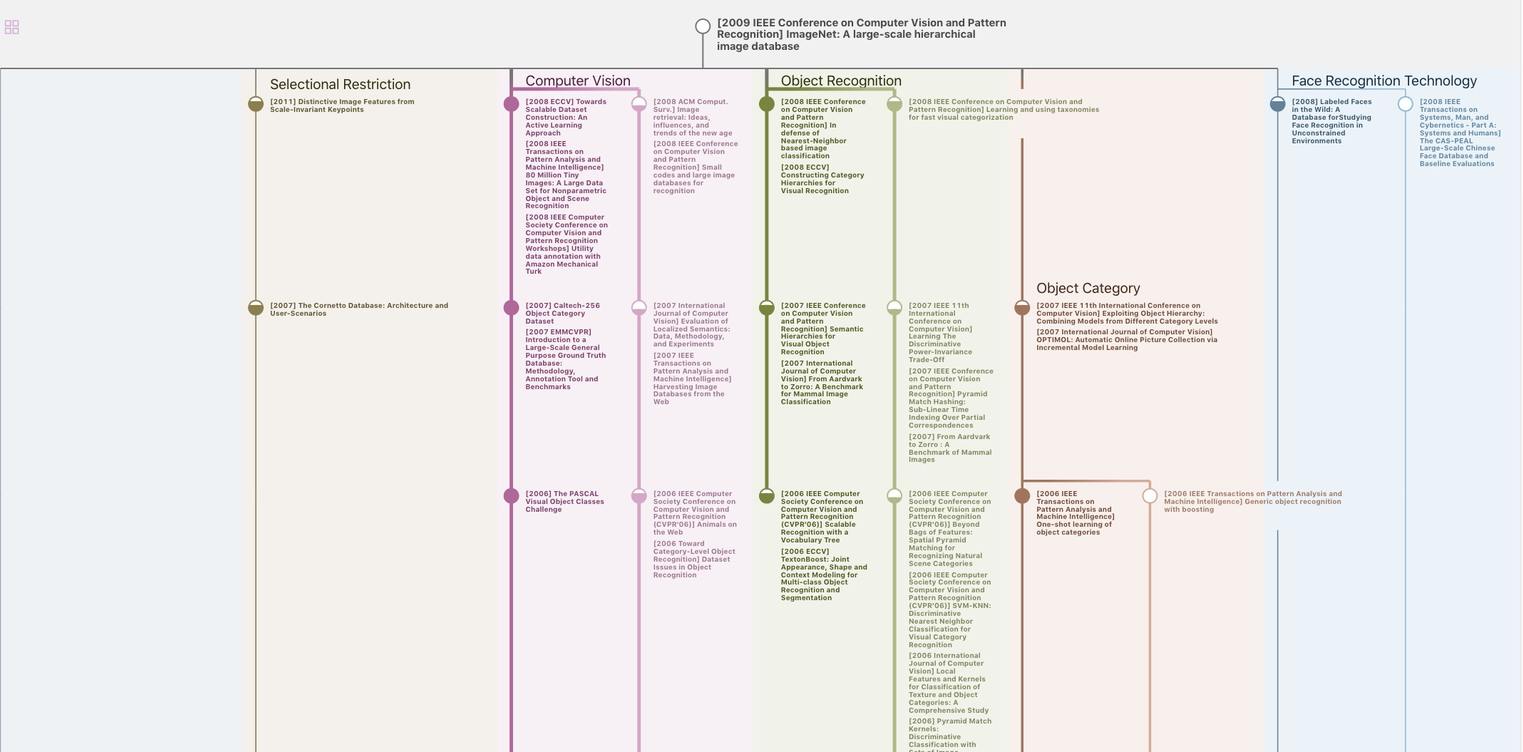
生成溯源树,研究论文发展脉络
Chat Paper
正在生成论文摘要