Adder Neural Networks for Speaker Verification.
ICCCS(2023)
Abstract
In recent years, the speaker verification (SV) technology based on deep neural networks is the most advanced and mainstream technology in the industry. However, since deep neural networks require a lot of storage resources and energy costs, this kind of SV technology cannot be deployed directly on devices with limited resources and low power consumption. In order to address this issue, we propose to use Adder Neural Network (AdderNet), which can replace the traditional multiplication in the network architecture with cheap addition. Additionally, we introduce an additive self-attention pooling layer, to apply the adder operation in the Self-attention pool (SAP) layer. The proposed speaker verification network can significantly reduce energy consumption and model computation costs with the performance maintained. Experimental results on the VoxCeleb1 dataset show our model consumes half of the energy of the original model, while the optimal EER is only reduced by 0.22%. Further, experiments on the CN-celeb dataset show that our method can achieve the CNN approach to approximate the results, demonstrating its generality.
MoreTranslated text
Key words
speaker verification,low-cost,neural network quantization
AI Read Science
Must-Reading Tree
Example
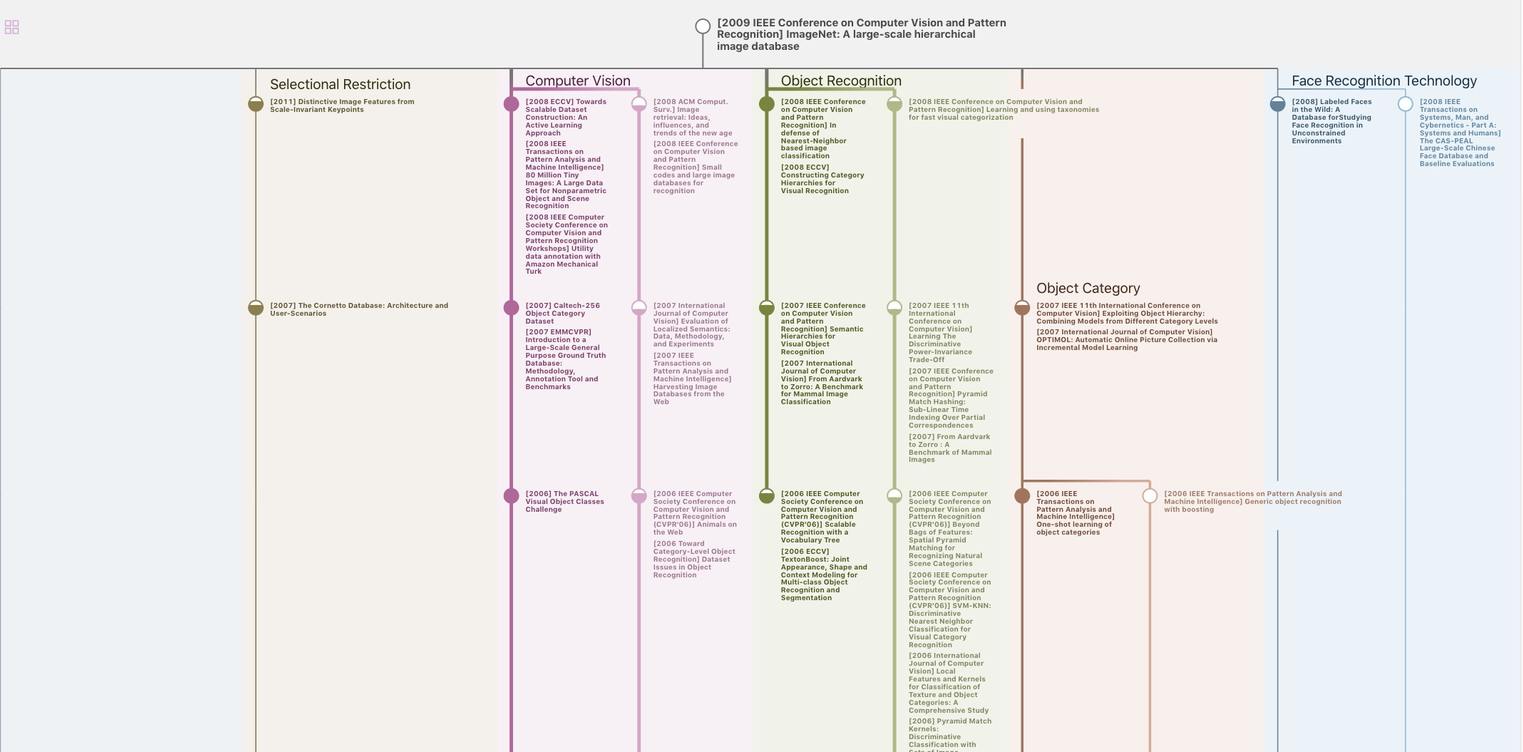
Generate MRT to find the research sequence of this paper
Chat Paper
Summary is being generated by the instructions you defined