Deep Reinforcement Learning For Connection Density Maximization in NOMA-based NB-IoT Networks.
ICCCS(2023)
Abstract
Narrowband Internet of things (NB-IoT) is a promising radio access technology to support massive machine-type communications (mMTC) in 5G and beyond wireless networks. To address the issue of connection density maximization in a non-orthogonal multiple access (NOMA) based NB-IoT network, in this paper, we investigate a deep reinforcement learning (DRL) approach. Firstly, we formulate the radio resource allocation problem of NB-IoT devices with various tone modes as a complex combinatorial optimization problem, aiming to maximize the connection density. Then we propose a DRL based NB-IoT resource allocation mechanism, which includes three different policy-based DRL algorithms, namely proximal policy optimization (PPO), asynchronous advantage actor critic (A3C), and multi-agent PPO to deal with stochastic packets arrivals and solve the optimization problem. Besides, considering the frequently fluctuating wireless network with large invalid action spaces, we also propose an action mask mechanism to accelerate training. The simulation results demonstrate that PPO outperforms A3C and MAPPO among our proposed DRL algorithms, and any of the three DRL algorithms outperforms the benchmark schemes.
MoreTranslated text
Key words
5G,mMTC,NB-IoT,NOMA,resource allocation,deep reinforcement learning
AI Read Science
Must-Reading Tree
Example
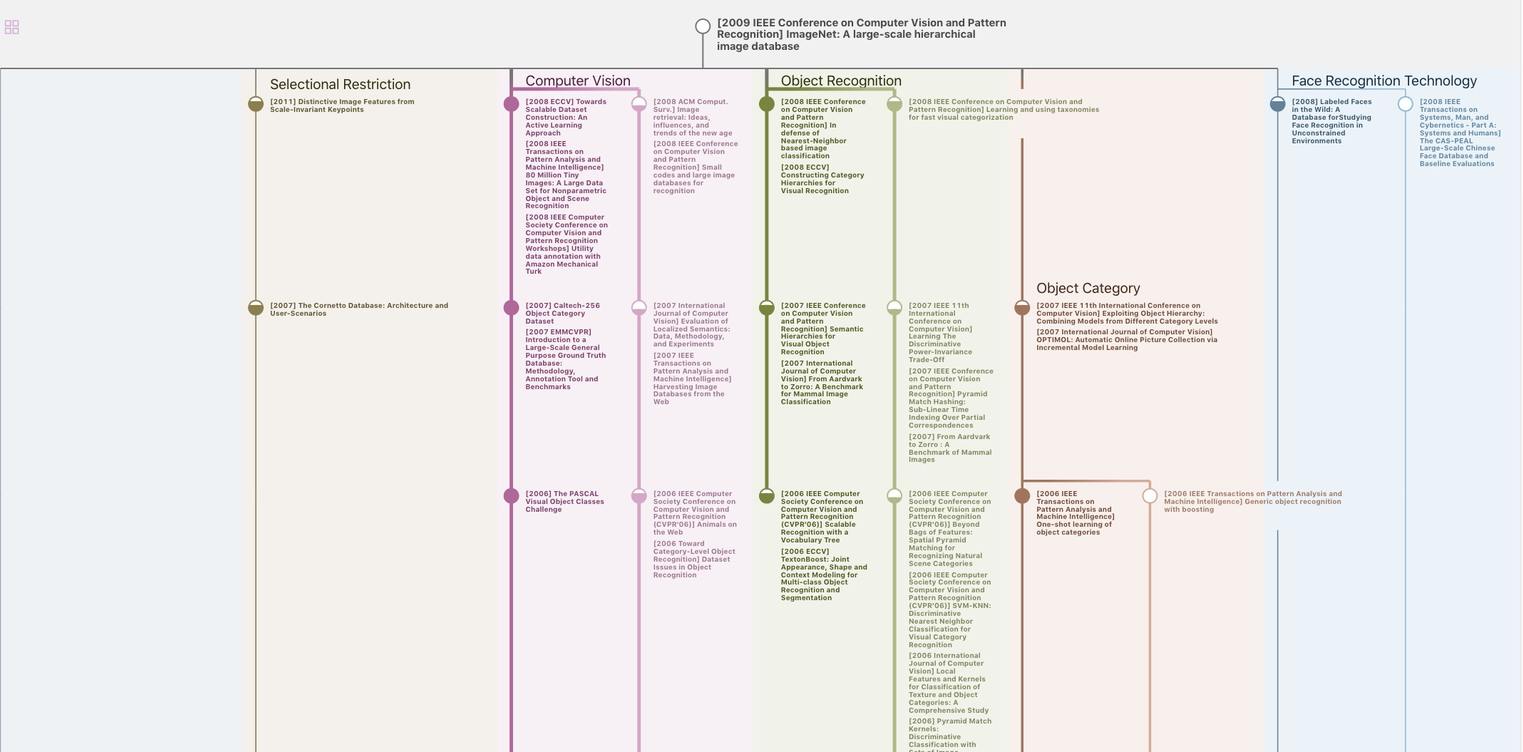
Generate MRT to find the research sequence of this paper
Chat Paper
Summary is being generated by the instructions you defined