NF-Net: Near and Far Network for Crowd Counting.
ICCCS(2023)
Abstract
In recent years, the research of crowd counting has aroused widespread attention in many fields such as video surveillance, public security, biomedicine, etc., and crowd counting has become one of the research hotspots in the field of computer vision. Due to factors such as the uncertain distribution of crowds, challenging scale changes, and complex scenes, most state-of-the-art methods adopt the method of constructing a density map and then use it as a learning objective to train a density estimator, however, this method generally performs poorly in the sparse crowd scene with few people. On the contrary, this paper proposes an end-to-end far-near distance network, called NF-Net. First of all, we designed a depth information module (DIM). The introduction of depth information weights into the module can distinguish far and near features well, divide the picture into four different patches, and understand the importance of each feature of each image position. Different receptive fields are assigned to groups of people with different degrees of distance. Secondly, a Near-block is proposed for patch application space for sparse crowds. After feature fusion, the output feature map enters the Near-block, and the method of deepening the network depth is used to enhance the feature extraction ability of pedestrians in sparse scenes, and a new network loss is proposed. Computational method A-Loss. Finally, we conduct extensive experiments on public datasets, demonstrating the effectiveness of NF-Net over baselines. In particular, our method currently achieves state-of-the-art performance on the ShanghaiTech dataset over previous methods. It has a great advantage in scenes where the crowd is sparser.
MoreTranslated text
Key words
object counting,CNNs,crowd counting,deep learning
AI Read Science
Must-Reading Tree
Example
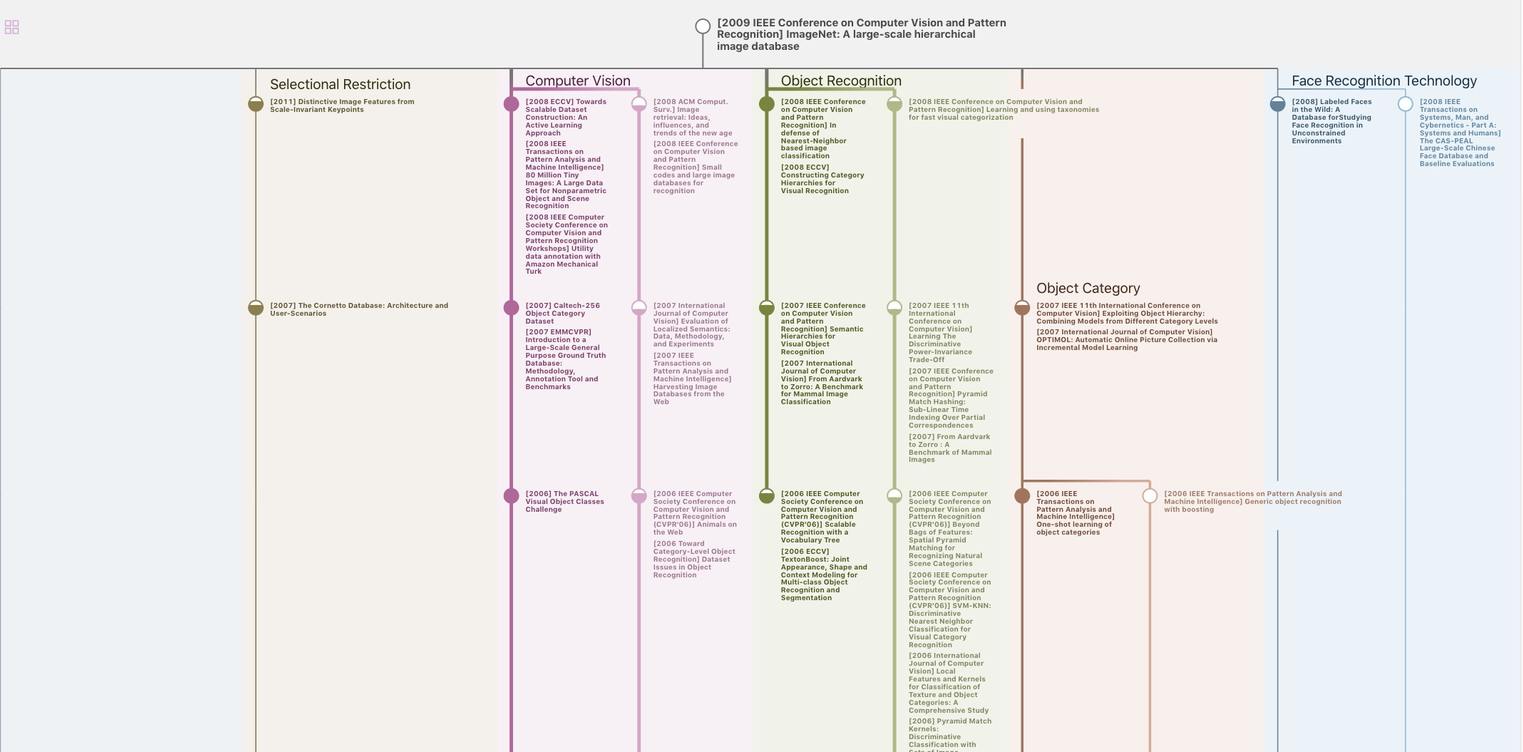
Generate MRT to find the research sequence of this paper
Chat Paper
Summary is being generated by the instructions you defined