FINAL: Factorized Interaction Layer for CTR Prediction
PROCEEDINGS OF THE 46TH INTERNATIONAL ACM SIGIR CONFERENCE ON RESEARCH AND DEVELOPMENT IN INFORMATION RETRIEVAL, SIGIR 2023(2023)
摘要
Multi-layer perceptron (MLP) serves as a core component in many deep models for click-through rate (CTR) prediction. However, vanilla MLP networks are inefficient in learning multiplicative feature interactions, making feature interaction learning an essential topic for CTR prediction. Existing feature interaction networks are effective in complementing the learning of MLPs, but they often fail to match the performance of MLPs when applied alone. Thus, their integration with MLP networks is necessary to achieve improved performance. This situation motivates us to explore a better alternative to the MLP backbone that could potentially replace MLPs. Inspired by factorization machines, in this paper, we propose FINAL, a factorized interaction layer that extends the widely-used linear layer and is capable of learning 2nd-order feature interactions. Similar to MLPs, multiple FINAL layers can be stacked into a FINAL block, yielding feature interactions with an exponential degree growth. We unify feature interactions and MLPs into a single FINAL block and empirically show its effectiveness as a replacement for the MLP block. Furthermore, we explore the ensemble of two FINAL blocks as an enhanced two-stream CTR model, setting a new state-of-the-art on open benchmark datasets. FINAL can be easily adopted as a building block and has achieved business metric gains in multiple applications at Huawei. Our source code will be made available at MindSpore/models.
更多查看译文
关键词
Recommender systems,CTR prediction,factorized interaction
AI 理解论文
溯源树
样例
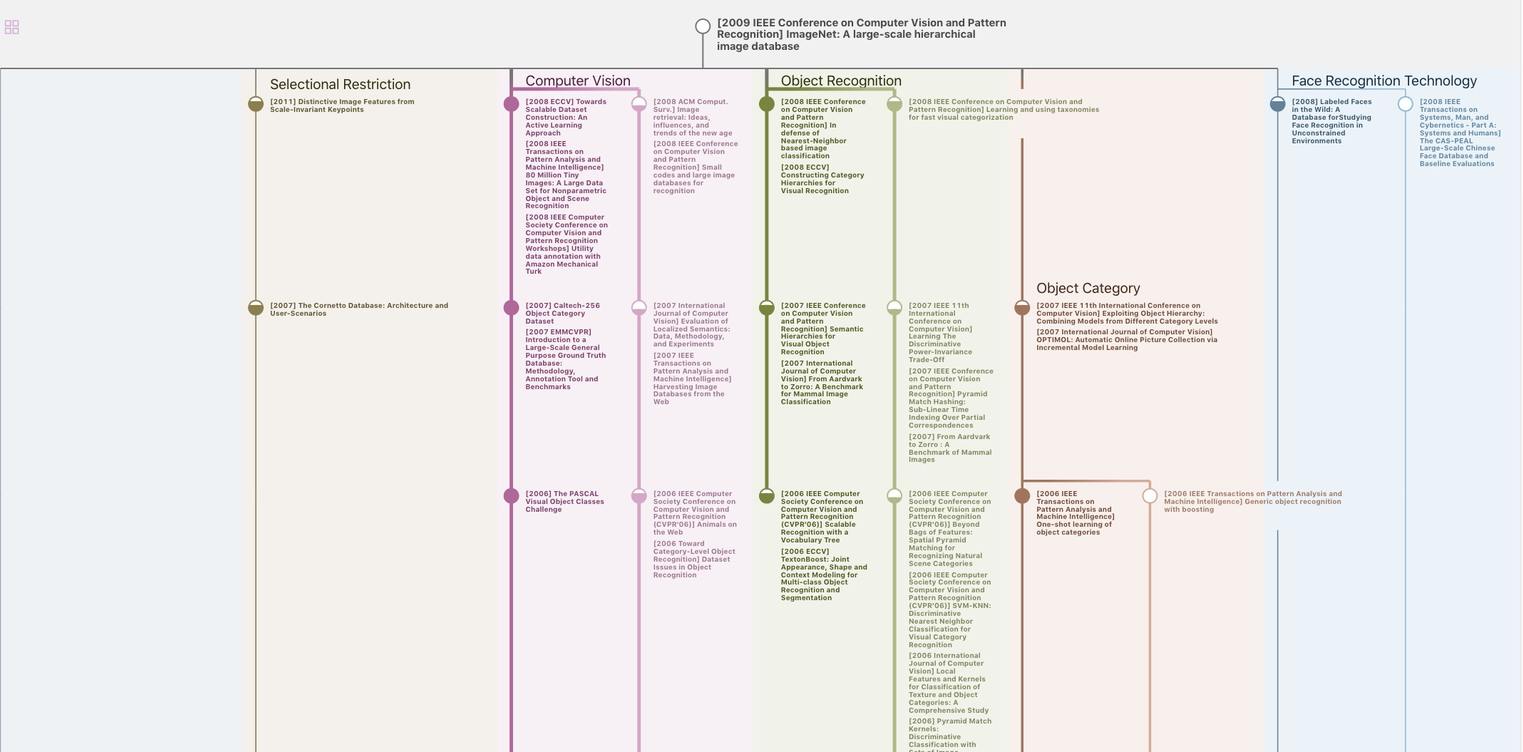
生成溯源树,研究论文发展脉络
Chat Paper
正在生成论文摘要