Evaluating Cross-modal Generative Models Using Retrieval Task
PROCEEDINGS OF THE 46TH INTERNATIONAL ACM SIGIR CONFERENCE ON RESEARCH AND DEVELOPMENT IN INFORMATION RETRIEVAL, SIGIR 2023(2023)
摘要
Generative models have taken the world by storm - image generative models such as Stable Diffusion and DALL-E generate photorealistic images, whereas image captioning models such as BLIP, GIT, ClipCap, and ViT-GPT2 generate descriptive and informative captions. While it may be true that these models produce remarkable results, their systematic evaluation is missing, making it hard to advance the research further. Currently, heuristic metrics such as the Inception Score and the Frechet Inception Distance are the most prevalent metrics for the image generation task, while BLEU, CIDEr, SPICE, METEOR, BERTScore, and CLIPScore are common for the image captioning task. Unfortunately, these are poorly interpretable and are not based on the solid user-behavior model that the Information Retrieval community has worked towards. In this paper, we present a novel cross-modal retrieval framework to evaluate the effectiveness of cross-modal (image-to-text and text-to-image) generative models using reference text and images. We propose the use of scoring models based on user behavior, such as Normalized Discounted Cumulative Gain (nDCG'@K) and Rank-Biased Precision (RBP'@K) adjusted for incomplete judgments. Experiments using ECCV Caption and Flickr8k-EXPERTS benchmark datasets demonstrate the effectiveness of various image captioning and image generation models for the proposed retrieval task. Results also indicate that the nDCG'@K and RBP'@K scores are consistent with heuristics-driven metrics, excluding CLIPScore, in model selection.
更多查看译文
关键词
Evaluation method,cross-modal generative modal,cross-modal retrieval
AI 理解论文
溯源树
样例
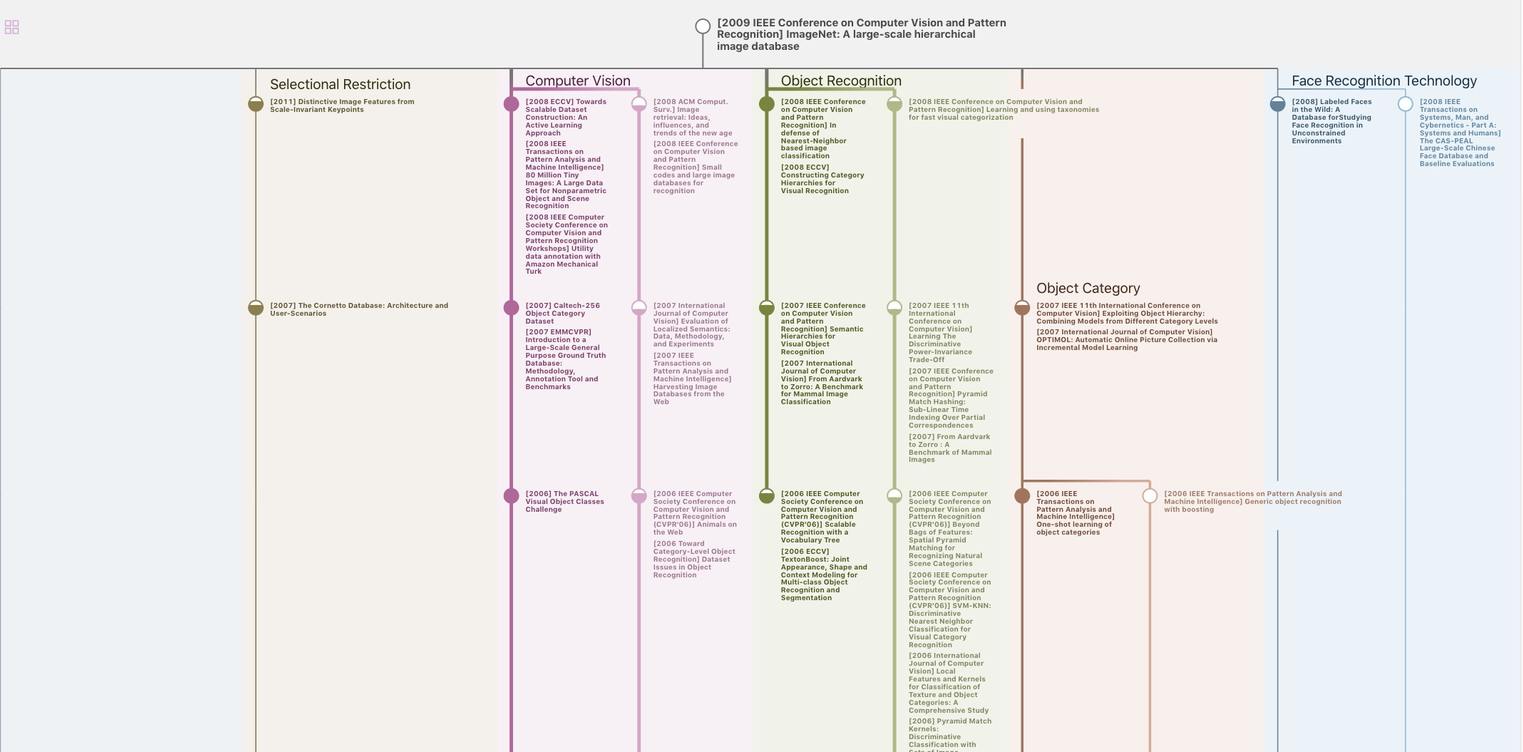
生成溯源树,研究论文发展脉络
Chat Paper
正在生成论文摘要