ML-LJP: Multi-Law Aware Legal Judgment Prediction
PROCEEDINGS OF THE 46TH INTERNATIONAL ACM SIGIR CONFERENCE ON RESEARCH AND DEVELOPMENT IN INFORMATION RETRIEVAL, SIGIR 2023(2023)
摘要
Legal judgment prediction (LJP) is a significant task in legal intelligence, which aims to assist the judges and determine the judgment result based on the case's fact description. The judgment result consists of law articles, charge, and prison term. The law articles serve as the basis for the charge and the prison term, which can be divided into two types, named as charge-related law article and term-related law article, respectively. Recently, many methods have been proposed and made tremendous progress in LJP. However, the existing methods only focus on the prediction of the charge-related law articles, ignoring the term-related law articles (e.g., laws about lenient treatment), which limits the performance in the prison term prediction. In this paper, following the actual legal process, we expand the law article prediction as a multi-label classification task that includes both the charge-related law articles and term-related law articles and propose a novel multi-law aware LJP (ML-LJP) method to improve the performance of LJP. Given the case's fact description, firstly, the label (e.g., law article and charge) definitions in the Code of Law are used to transform the representation of the fact into several label-specific representations and make the prediction of the law articles and the charge. To distinguish the similar content of different label definitions, contrastive learning is conducted in the training. Then, a graph attention network (GAT) is applied to learn the interactions among the multiple law articles for the prediction of the prison term. Since numbers (e.g., amount of theft and weight of drugs) are important for LJP but often ignored by conventional encoders, we design a corresponding number representation method to locate and better represent these effective numbers. Extensive experiments on real-world dataset show that our method achieves the best results compared to the state-of-the-art models, especially in the task of prison term prediction where ML-LJP achieves a 10.07% relative improvement over the best baseline.
更多查看译文
关键词
LegalAI,Multi-task Learning,Graph Attention Network
AI 理解论文
溯源树
样例
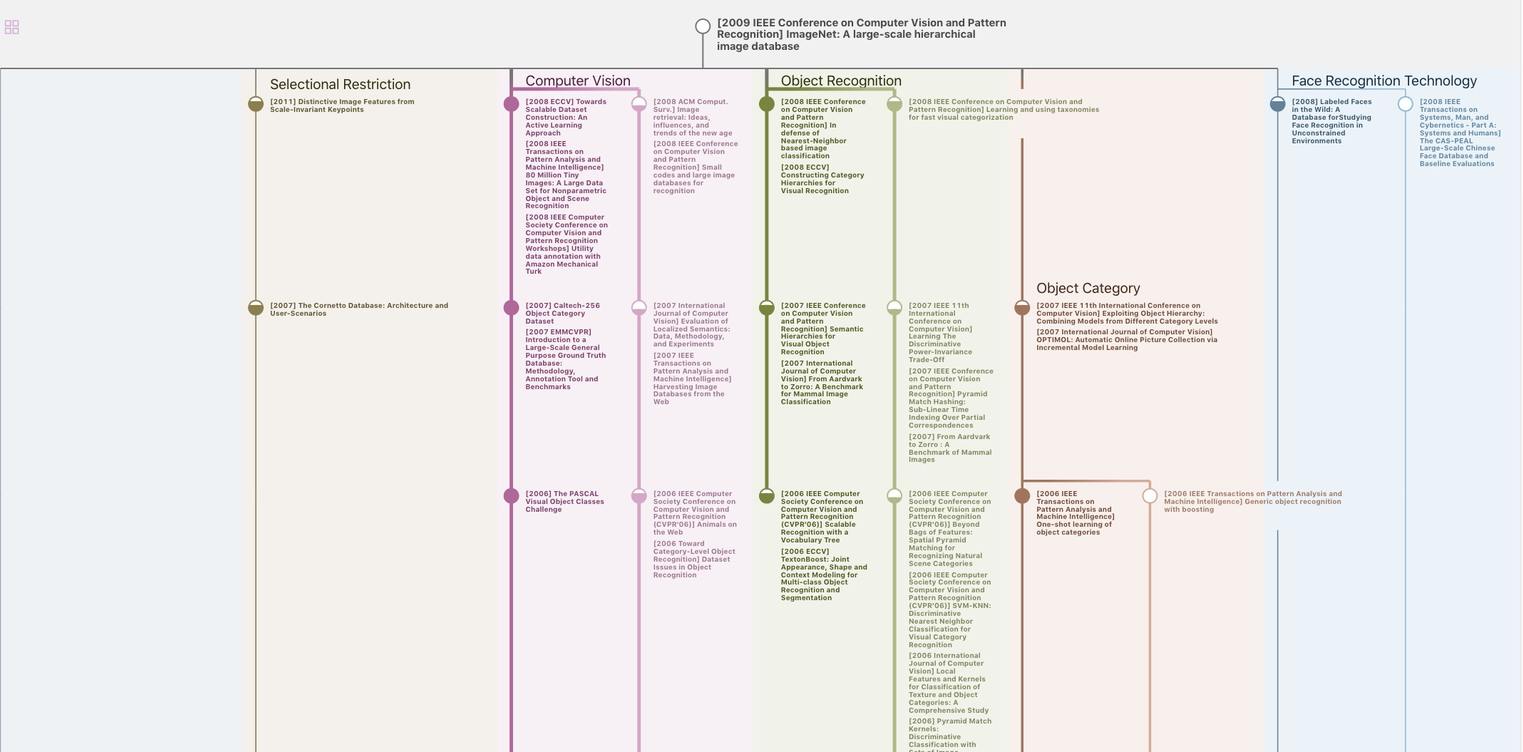
生成溯源树,研究论文发展脉络
Chat Paper
正在生成论文摘要