Leveraging Transferable Knowledge Concept Graph Embedding for Cold-Start Cognitive Diagnosis
PROCEEDINGS OF THE 46TH INTERNATIONAL ACM SIGIR CONFERENCE ON RESEARCH AND DEVELOPMENT IN INFORMATION RETRIEVAL, SIGIR 2023(2023)
摘要
Cognitive diagnosis (CD) aims to reveal the proficiency of students on specific knowledge concepts and traits of test exercises (e.g., difficulty). It plays a critical role in intelligent education systems by supporting personalized learning guidance. However, recent developments in CD mostly concentrate on improving the accuracy of diagnostic results and often overlook the important and practical task: domain-level zero-shot cognitive diagnosis (DZCD). The primary challenge of DZCD is the deficiency of student behavior data in the target domain due to the absence of student-exercise interactions or unavailability of exercising records for training purposes. To tackle the cold-start issue, we propose a two-stage solution named TechCD (Transferable knowledgE Concept grapH embedding framework for Cognitive Diagnosis). The fundamental notion involves utilizing a pedagogical knowledge concept graph (KCG) as a mediator to connect disparate domains, allowing the transmission of student cognitive signals from established domains to the zero-shot cold-start domain. Specifically, a naive yet effective graph convolutional network (GCN) with the bottom-layer discarding operation is initially employed over the KCG to learn transferable student cognitive states and domain-specific exercise traits. Moreover, we give three implementations of the general TechCD framework following the typical cognitive diagnosis solutions. Finally, extensive experiments on real-world datasets not only prove that Tech can effectively perform zero-shot diagnosis, but also give some popular applications such as exercise recommendation.
更多查看译文
关键词
cognitive diagnosis,student performance prediction,cold-start,knowledge concept graph
AI 理解论文
溯源树
样例
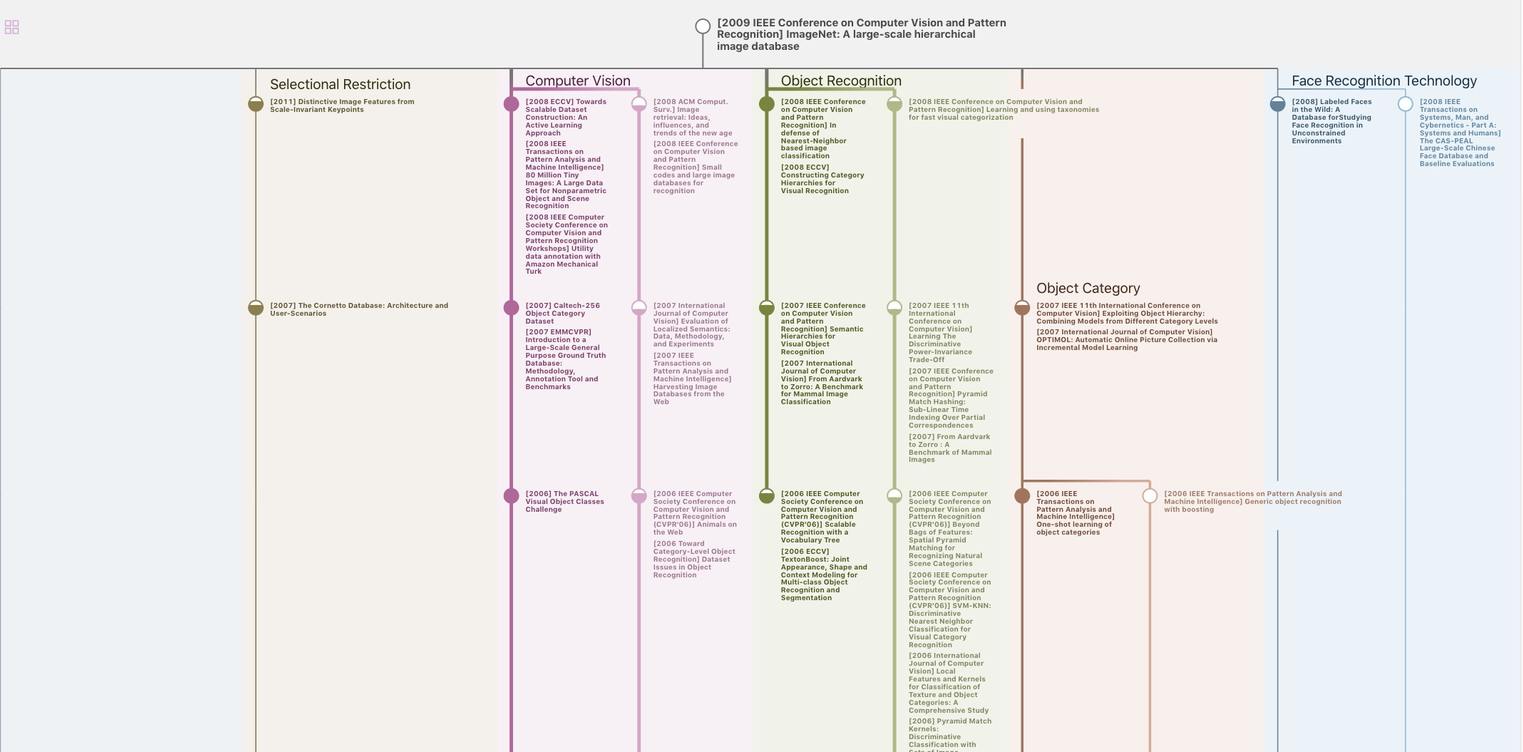
生成溯源树,研究论文发展脉络
Chat Paper
正在生成论文摘要