An Effective Framework for Enhancing Query Answering in a Heterogeneous Data Lake
PROCEEDINGS OF THE 46TH INTERNATIONAL ACM SIGIR CONFERENCE ON RESEARCH AND DEVELOPMENT IN INFORMATION RETRIEVAL, SIGIR 2023(2023)
Abstract
There has been a growing interest in cross-source searching to gain rich knowledge in recent years. A data lake collects massive raw and heterogeneous data with different data schemas and query interfaces. Many real-life applications require query answering over the heterogeneous data lake, such as e-commerce, bioinformatics and healthcare. In this paper, we propose LakeAns that semantically integrates heterogeneous data schemas of the lake to enhance the semantics of query answers. To this end, we propose a novel framework to efficiently and effectively perform the cross-source searching. The framework exploits a reinforcement learning method to semantically integrate the data schemas and further create a global relational schema for the heterogeneous data. It then performs a query answering algorithm based on the global schema to find answers across multiple data sources. We conduct extensive experimental evaluations using real-life data to verify that our approach outperforms existing solutions in terms of effectiveness and efficiency.
MoreTranslated text
Key words
heterogeneous data lake,relational schema,query answering
AI Read Science
Must-Reading Tree
Example
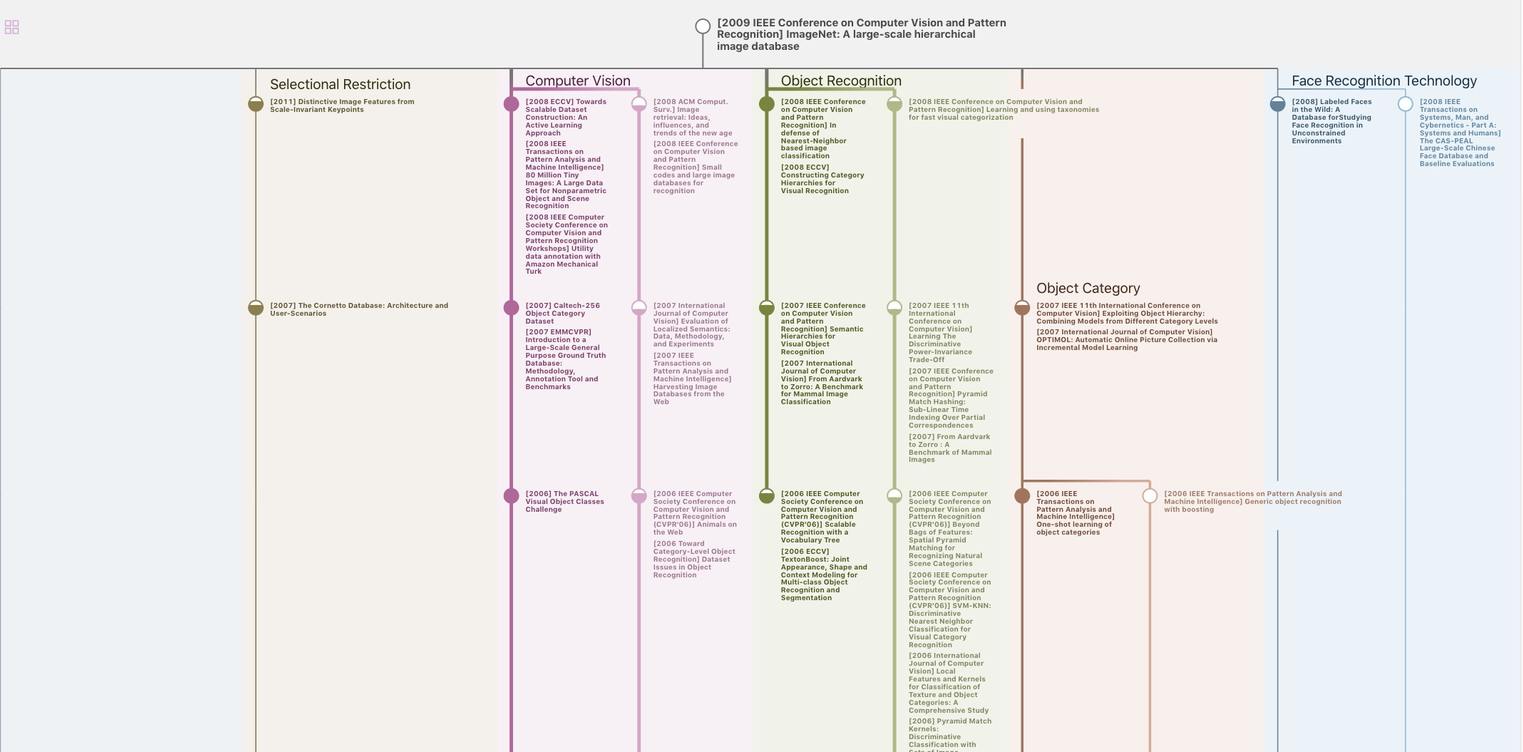
Generate MRT to find the research sequence of this paper
Chat Paper
Summary is being generated by the instructions you defined