Intrusion detection system for large-scale IoT NetFlow networks using machine learning with modified Arithmetic Optimization Algorithm.
Internet Things(2023)
摘要
With the rapid expansion of Internet of Things (IoT) networks, the need for robust security measures to detect and report potential threats is becoming more urgent. In this paper, we propose a Network Intrusion Detection System (NIDS), as a security measure, for large-scale IoT NetFlow-based networks. The proposed NIDS employs Machine Learning (ML) boosted by a modified version of the Arithmetic Optimization Algorithm (AOA) to determine the most suitable set of features. The selected seven features are used to train several ML models, including Random Forest and Extra Trees. Our research utilized four large datasets, released in 2021, containing IoT traffic data represented in a standard set of 43 NetFlow-based features. Reducing the number of features from 43 to 7 enhanced the prediction time and, consequently, the performance in the real world. Interestingly, the proposed NIDS exhibited a very accurate and robust detection model for IoT NetFlow data, which can be generalized for other Intrusion Detection datasets. Our proposed NIDS achieved up to 99% and 98% accuracy for binary and multi-classification, respectively. These scores were similar to those achieved by the state-of-the-art systems despite decreasing the number of utilized features by up to 84%.
更多查看译文
关键词
iot,large-scale
AI 理解论文
溯源树
样例
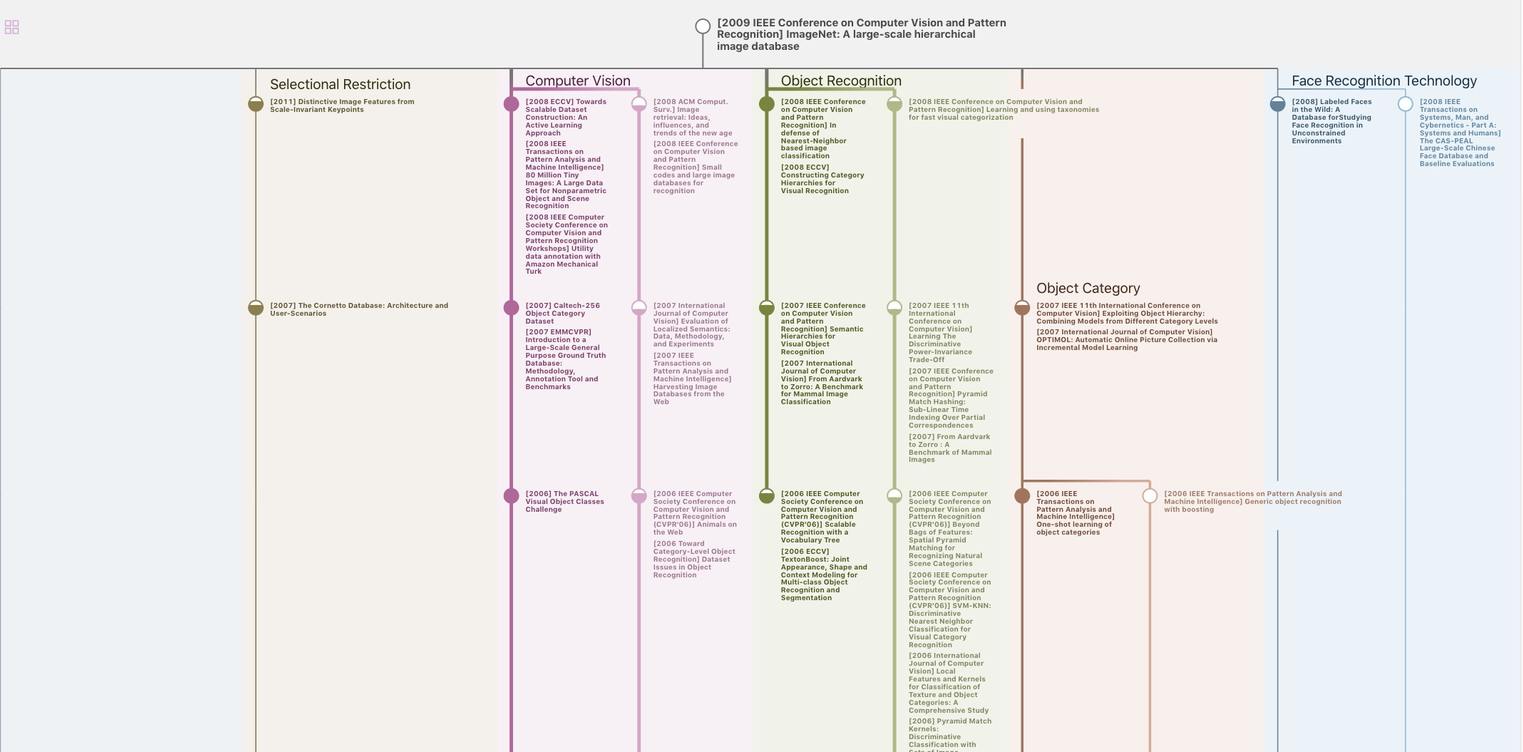
生成溯源树,研究论文发展脉络
Chat Paper
正在生成论文摘要