DCBT: A Simple But Effective Way for Unified Warm and Cold Recommendation
PROCEEDINGS OF THE 46TH INTERNATIONAL ACM SIGIR CONFERENCE ON RESEARCH AND DEVELOPMENT IN INFORMATION RETRIEVAL, SIGIR 2023(2023)
Abstract
The cold-start problem of conversion rate prediction is a common challenge in online advertising systems. To alleviate this problem, a large number of methods either use content information or uncertainty methods, or use meta-learning-based methods to improve the ranking performance of cold-start items. However, they can work for cold-start scenarios but fail to adaptively unify warm and cold recommendations into one model, requiring additional human efforts or knowledge to adapt to different scenarios. Meanwhile, none of them pay attention to the discrepancy between model predictions and true likelihoods of cold items, while over- or under-estimation is harmful to the ROI (Return on Investment) of advertising placements. In this paper, in order to address the above issues, we propose a framework called Distribution-Constrained Batch Transformer (DCBT). Specifically, the framework introduces a Transformer module into the batch dimension to automatically choose proper information from warm samples to enhance the representation of cold samples and preserve the property of warm samples. In addition, to avoid the distribution of cold samples being affected by the warm samples, the framework adds MMD loss to constrain the sample distribution before and after feeding into the Transformer module. Extensive offline experiments on two real-world datasets show that our proposed method attains state-of-the-art performance in AUC and PCOC (Predicted CVR over CVR) for warm and cold items. An online A/B test demonstrates that the DCBT model obtained a 20.08% improvement in CVR and a 13.21% increase in GMV (Gross Merchandise Volume).
MoreTranslated text
Key words
Cold-Start,Advertising Systems,Transformer
AI Read Science
Must-Reading Tree
Example
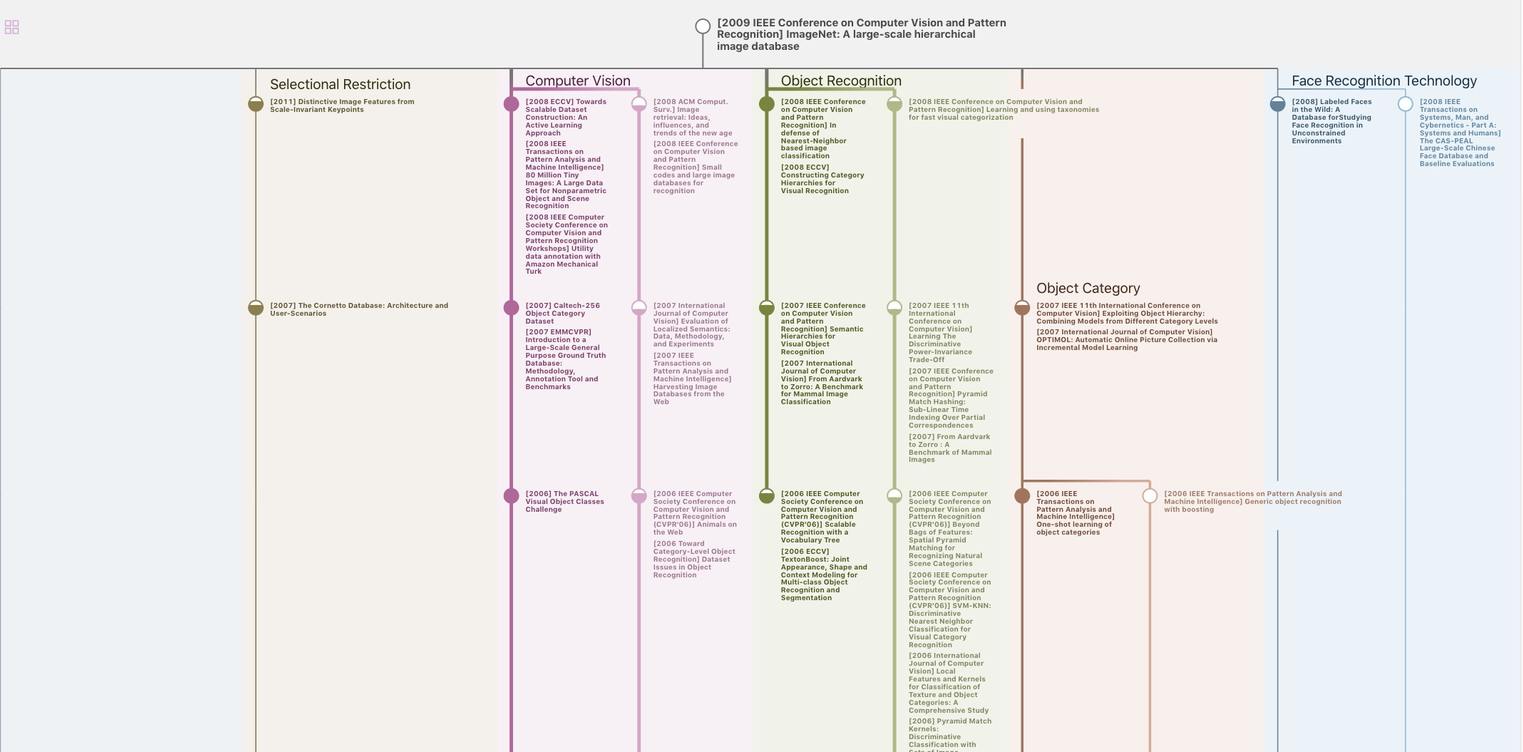
Generate MRT to find the research sequence of this paper
Chat Paper
Summary is being generated by the instructions you defined