RiverText: A Python Library for Training and Evaluating IncrementalWord Embeddings from Text Data Streams
SIGIR(2023)
摘要
Word embeddings have become essential components in various information retrieval and natural language processing tasks, such as ranking, document classification, and question answering. However, despite their widespread use, traditional word embedding models present a limitation in their static nature, which hampers their ability to adapt to the constantly evolving language patterns that emerge in sources such as social media and the web (e.g., new hashtags or brand names). To overcome this problem, incremental word embedding algorithms are introduced, capable of dynamically updating word representations in response to new language patterns and processing continuous data streams. This paper presents RiverText, a Python library for training and evaluating incremental word embeddings from text data streams. Our tool is a resource for the information retrieval and natural language processing communities that work with word embeddings in streaming scenarios, such as analyzing social media. The library implements different incrementalword embedding techniques, such as Skip-gram, Continuous Bag of Words, and Word Context Matrix, in a standardized framework. In addition, it uses PyTorch as its backend for neural network training. We have implemented a module that adapts existing intrinsic static word embedding evaluation tasks for word similarity and word categorization to a streaming setting. Finally, we compare the implemented methods with different hyperparameter settings and discuss the results. Our open-source library is available at https://github.com/dccuchile/ rivertext.
更多查看译文
关键词
word embedding,incremental learning,data streams
AI 理解论文
溯源树
样例
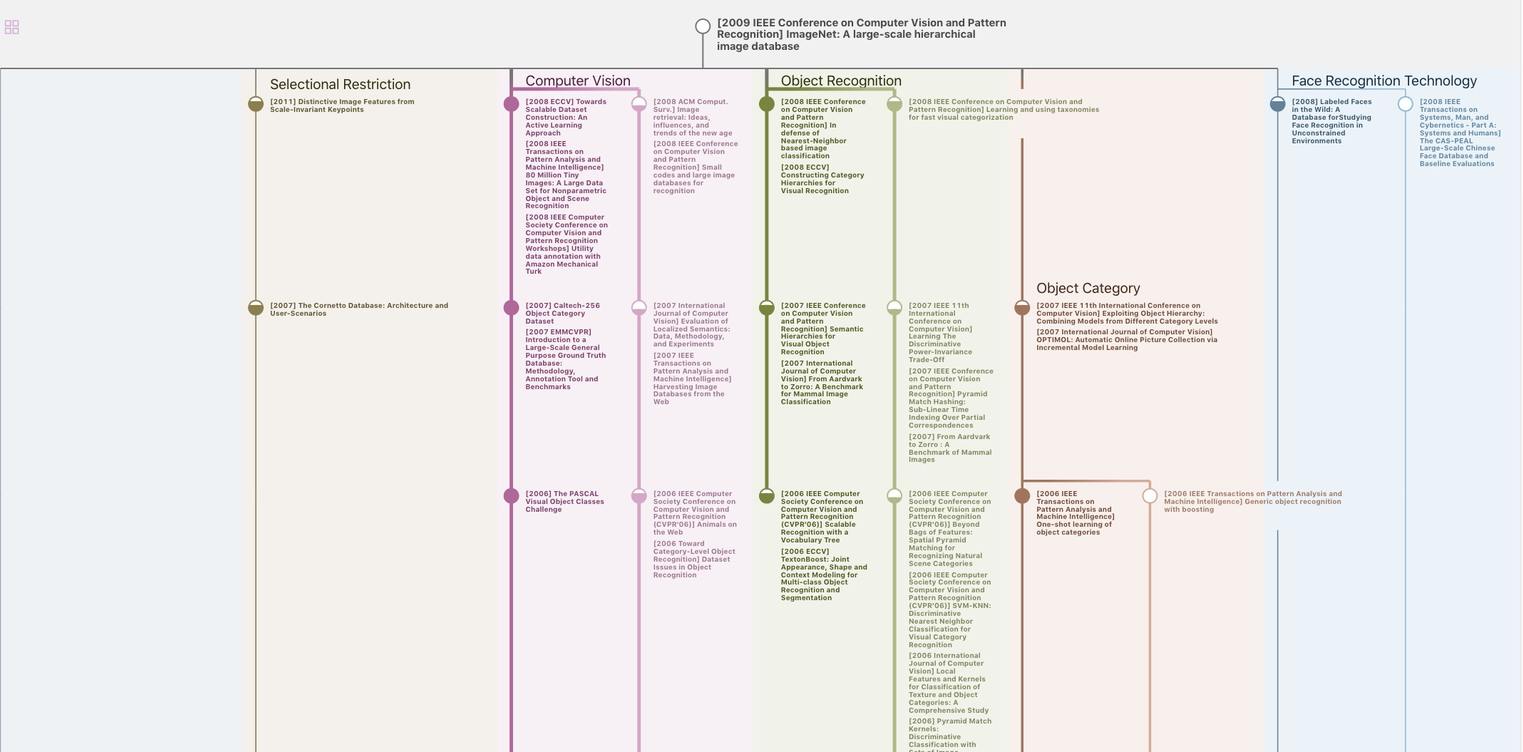
生成溯源树,研究论文发展脉络
Chat Paper
正在生成论文摘要