FLIRT: Federated Learning for Information Retrieval
PROCEEDINGS OF THE 46TH INTERNATIONAL ACM SIGIR CONFERENCE ON RESEARCH AND DEVELOPMENT IN INFORMATION RETRIEVAL, SIGIR 2023(2023)
摘要
A wide range of core information retrieval (IR) tasks, such as searching, ranking, and filtering, to name a few, have seen tremendous improvements thanks to machine learning (ML) and artificial intelligence (AI). The traditional centralized approach to training AI/ML models is still predominant: large volumes of data generated by end users must be transferred from their origins and shared with remote locations for processing. However, this centralized paradigm suffers from significant privacy issues and does not take full advantage of the computing power of client devices like modern smartphones. A possible answer to this need is provided by federated learning (FL), which enables collaborative training of predictive models among a set of cooperating edge devices without disclosing any private local data. Unfortunately, FL is still far from being fully exploited in the IR ecosystem. In this workshop proposal, we have the ambition to start filling this gap. More specifically, the first workshop on "Federated Learning for Information ReTrieval" (FLIRT) is willing to provide an open forum for researchers and practitioners where they can exchange ideas, identify key challenges, and define the roadmap toward a successful application of FL in the broad IR area.
更多查看译文
关键词
Federated learning,information retrieval
AI 理解论文
溯源树
样例
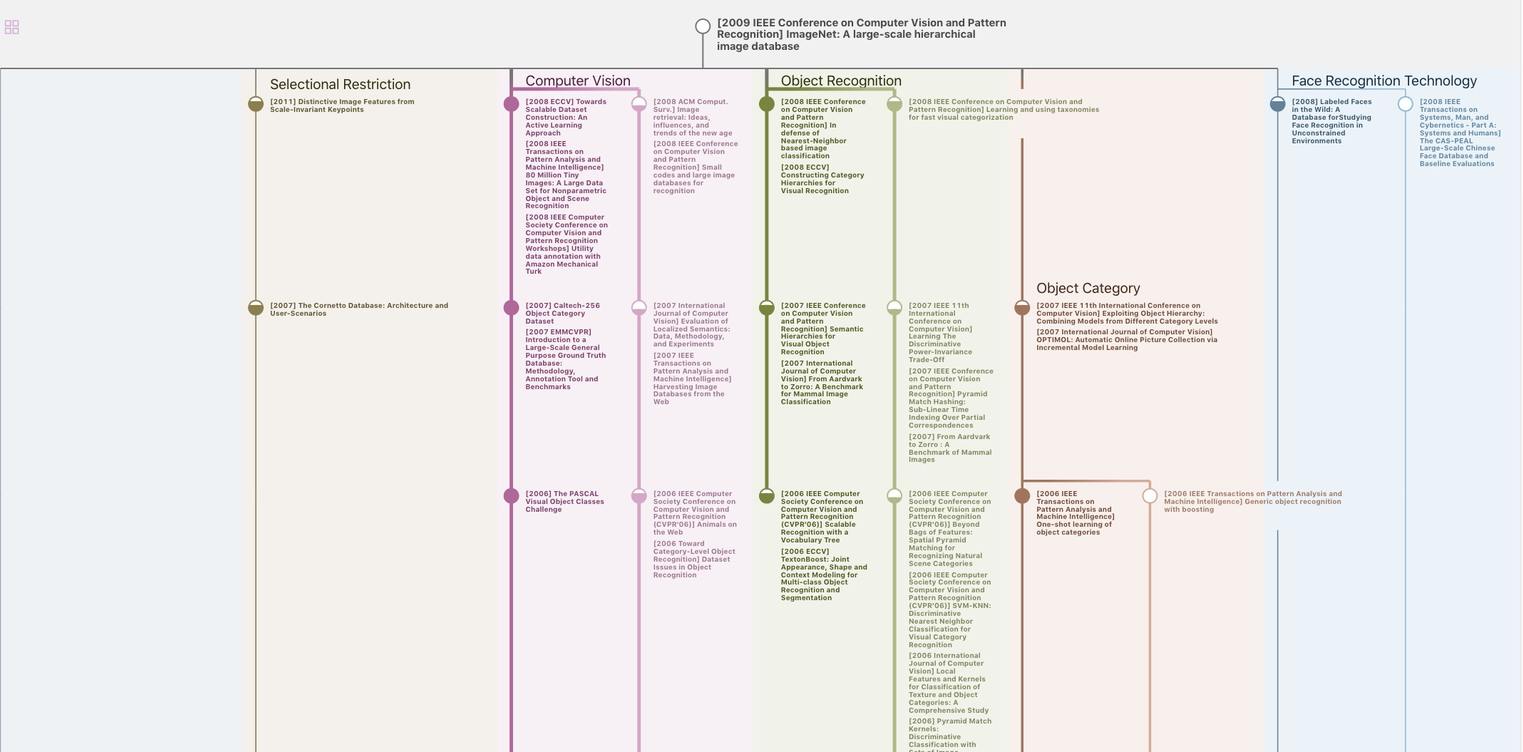
生成溯源树,研究论文发展脉络
Chat Paper
正在生成论文摘要